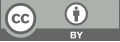
Performance Comparison of ControlNet Models Based on PONY in Complex Human Pose Image Generation
- 1 School of Artificial Intelligence, Nanjing University of Aeronautics and Astronautics, Nanjing, China
* Author to whom correspondence should be addressed.
Abstract
Over the past two years, text-to-image diffusion models have advanced considerably. The PONY model, in particular, excels at generating high-quality anime character images from open-domain text descriptions. However, such text descriptions often lack the granularity needed for detailed control, especially in the context of complex human pose generation. To mitigate this limitation, recent research has introduced ControlNet to enhance the control capabilities of stable diffusion models. Nevertheless, the efficacy of a single model remains suboptimal for generating complex poses, highlighting the potential of combining multiple ControlNet models. This paper introduces the Depth+OpenPose methodology, a multi-ControlNet approach that enables simultaneous local control of depth maps and pose maps, in addition to other global controls. Distinct from single or other combined methods, Depth+OpenPose incorporates an additional conditional input. For addressing limb occlusion issues, depth maps provide positional relationships, while OpenPose captures facial expressions and hand poses, surpassing the performance of single models. Furthermore, Depth+OpenPose demonstrates superior speed and quality relative to other combinations. It is crucial to note that an excessive number of combinations can lead to too many conditional inputs, thereby reducing control efficacy. Through comprehensive quantitative and qualitative experimental comparisons, Depth+OpenPose proves its superiority in terms of speed, image quality, and versatility over existing methodologies.
Keywords
ControlNet, Stable Diffusion, Image Generation, Complex Human Posture, PONY
[1]. Rombach, R., Blattmann, A., Lorenz, D., Esser, P., & Ommer, B. (2022). High-resolution image synthesis with latent diffusion models. In Proceedings of the IEEE/CVF conference on computer vision and pattern recognition. 10684-10695.
[2]. Podell, D., English, Z., Lacey, K., Blattmann, A., Dockhorn, T., Müller, J., ... & Rombach, R. (2023). Sdxl: Improving latent diffusion models for high-resolution image synthesis. arXiv preprint arXiv:2307.01952.
[3]. Zhao, S., Chen, D., Chen, Y. C., Bao, J., Hao, S., Yuan, L., & Wong, K. Y. K. (2024). Uni-controlnet: All-in-one control to text-to-image diffusion models. Advances in Neural Information Processing Systems, 36.
[4]. Zhang, L., Rao, A., & Agrawala, M. (2023). Adding conditional control to text-to-image diffusion models. In Proceedings of the IEEE/CVF International Conference on Computer Vision. pp. 3836-3847.
[5]. Rong, W., Li, Z., Zhang, W., & Sun, L. (2014). An improved CANNY edge detection algorithm. In 2014 IEEE international conference on mechatronics and automation. 577-582.
[6]. Yang, L., Kang, B., Huang, Z., Xu, X., Feng, J., & Zhao, H. (2024). Depth anything: Unleashing the power of large-scale unlabeled data. In Proceedings of the IEEE/CVF Conference on Computer Vision and Pattern Recognition. 10371-10381.
[7]. Cao, Z., Simon, T., Wei, S. E., & Sheikh, Y. (2017). Realtime multi-person 2d pose estimation using part affinity fields. In Proceedings of the IEEE conference on computer vision and pattern recognition. 7291-7299.
[8]. Ho, J., Salimans, T., Gritsenko, A., Chan, W., Norouzi, M., & Fleet, D. J. (2022). Video diffusion models. Advances in Neural Information Processing Systems, 35, 8633-8646.
[9]. Hu, E. J., Shen, Y., Wallis, P., Allen-Zhu, Z., Li, Y., Wang, S., ... & Chen, W. (2021). Lora: Low-rank adaptation of large language models. arXiv preprint arXiv:2106.09685.
[10]. Barratt, S., & Sharma, R. (2018). A note on the inception score. arXiv preprint arXiv:1801.01973.
[11]. Heusel, M., Ramsauer, H., Unterthiner, T., Nessler, B., & Hochreiter, S. (2017). Gans trained by a two time-scale update rule converge to a local nash equilibrium. Advances in neural information processing systems, 30.
[12]. Wu, H., Mao, J., Zhang, Y., Jiang, Y., Li, L., Sun, W., & Ma, W. Y. (2019). Unified visual-semantic embeddings: Bridging vision and language with structured meaning representations. In Proceedings of the IEEE/CVF Conference on Computer Vision and Pattern Recognition. 6609-6618.
Cite this article
Zeng,Q. (2024). Performance Comparison of ControlNet Models Based on PONY in Complex Human Pose Image Generation. Theoretical and Natural Science,52,90-98.
Data availability
The datasets used and/or analyzed during the current study will be available from the authors upon reasonable request.
Disclaimer/Publisher's Note
The statements, opinions and data contained in all publications are solely those of the individual author(s) and contributor(s) and not of EWA Publishing and/or the editor(s). EWA Publishing and/or the editor(s) disclaim responsibility for any injury to people or property resulting from any ideas, methods, instructions or products referred to in the content.
About volume
Volume title: Proceedings of CONF-MPCS 2024 Workshop: Quantum Machine Learning: Bridging Quantum Physics and Computational Simulations
© 2024 by the author(s). Licensee EWA Publishing, Oxford, UK. This article is an open access article distributed under the terms and
conditions of the Creative Commons Attribution (CC BY) license. Authors who
publish this series agree to the following terms:
1. Authors retain copyright and grant the series right of first publication with the work simultaneously licensed under a Creative Commons
Attribution License that allows others to share the work with an acknowledgment of the work's authorship and initial publication in this
series.
2. Authors are able to enter into separate, additional contractual arrangements for the non-exclusive distribution of the series's published
version of the work (e.g., post it to an institutional repository or publish it in a book), with an acknowledgment of its initial
publication in this series.
3. Authors are permitted and encouraged to post their work online (e.g., in institutional repositories or on their website) prior to and
during the submission process, as it can lead to productive exchanges, as well as earlier and greater citation of published work (See
Open access policy for details).