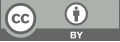
Non-Contact Human Body Dimension Estimation Methods Based on Deep Learning: A Critical Analysis
- 1 School of Artificial Intelligence, South China Normal University, Guangzhou, China
* Author to whom correspondence should be addressed.
Abstract
With the advancement of information technology, Human Body Dimension Estimation (HBDE) is moving towards digitalization and non-contact methods. This paper critically reviews non-contact HBDE methods based on deep learning, primarily covering image-based methods, the Adaptive Body Structure Segmentation (ABSS) algorithm with height and weight measurements, and 3D meshes or point cloud data methods. Convolutional Neural Networks (CNNs) excel in image measurement, while the ABSS algorithm with adaptive segmentation achieves high accuracy. Recent studies, such as the Grey Wolf Optimizer-Elman Neural Network (GBWO-ENN) model, have significantly improved prediction accuracy by optimizing neural network structures. Additionally, 3D point cloud data combined with the Skinned Multi-Person Linear (SMPL) model has proven effective in complex scenarios. This paper summarizes the performance of various methods, analyzes common datasets, and explores future development directions for non-contact HBDE. Despite significant progress, this field still faces challenges such as data quality, scarcity of extreme samples, and privacy issues. Future research should focus on building high-quality datasets and developing lightweight measurement networks to meet practical application needs.
Keywords
Non-contact measurement, human body dimension estimation, deep learning, convolutional neural networks
[1]. Song, Y., Wirta, J., Kämäräinen, J. K. (2020). Anthropometric clothing measurements from 3d body scans. Machine Vision and Applications, 31(4): 7.
[2]. Dibra, E., Jain, H., Öztireli, C., Ziegler, R., Gross, M. (2016). Hs-nets: Estimating human body shape from silhouettes with convolutional neural networks. In International Conference on 3D Vision (3DV), 108–117.
[3]. Hu, X. R., Liu, J. W., Liu, J. P., et al. (2020). Multi-feature extraction and dimension measurement for dressed human bodies via adaptive body structure segmentation. Computer Engineering, 46(2): 238-246.
[4]. Hu, X. R., Liu, J. W., Liu, J. P., et al. (2021). Prediction of 3D human dimensions based on improved GA-BP-MC neural network. Computer Engineering and Science, 43(08): 1443-1453.
[5]. Ruiz, N., Bellver, M., Bolkart, T., Arora, A., Lin, M. C., Romero, J., Bala, R. (2022). Human body measurement estimation with adversarial augmentation. In International Conference on 3D Vision (3DV), Prague, Czech Republic, September 12-16. IEEE: 219-230. DOI: 10.1109/3DV57758.2022.00031.
[6]. Yang, X. W., Li, Y. T., Han, X., et al. (2024). Human dimension prediction model integrating GBWO and ENN. Computer Technology and Development, 34(06): 132-139. DOI: 10.20165/j.cnki.ISSN1673-629X.2024.0064.
[7]. Hu, X. R., Liu, J. W. (2023). Prediction of human circumference dimensions based on improved WOA-ENN-MC model. Computer Applications and Software, 40(05): 190-199.
[8]. Bogo, A., Kanazawa, A., Lassner, P., et al. (2016). Keep it SMPL: Automatic estimation of 3D human pose and shape from a single image. Proc of European Conference on Computer Vision (ECCV): 1.
[9]. Choutas, V., Müller, L., Huang, C. H. P., Tang, S., Tzionas, D., Black, M. J. (2022). Accurate 3D body shape regression using metric and semantic attributes. In IEEE/CVF Conference on Computer Vision and Pattern Recognition (CVPR 2022): 2708-2718. DOI: 10.1109/CVPR52688.2022.00274.
[10]. Alldieck, T., Marcus, M., Xu, W. P., et al. (2018). Video-based reconstruction of 3D people models. Proc of IEEE Conference on Computer Vision and Pattern Recognition (CVPR): 1.
[11]. Alldieck, T., Marcus, M. (2019). Learning to reconstruct people in clothing from a single RGB camera. Proc of IEEE Conference on Computer Vision and Pattern Recognition (CVPR): 1.
[12]. Sun, Y. L., Miao, Y. W., Bao, C. (2019). Global registration cumulative error minimization based 3D human body reconstruction using RGB-D scanning data. Journal of Computer-Aided Design & Computer Graphics, 31(9): 1467-1476.
[13]. Kazhdan, M., Hoppe, H. (2013). Screened Poisson surface reconstruction. ACM Transactions on Graphics, 32(3): Article No. 29.
[14]. González, T. Y., Mayer, H. A. (2021). A neural anthropometer learning from body dimensions computed on human 3D meshes. IEEE Symposium Series on Computational Intelligence (SSCI): 1-8.
[15]. Škorvánková, D., Riečický, A., Madaras, M. (2022). Automatic estimation of anthropometric human body measurements. Proceedings of the 17th International Joint Conference on Computer Vision, Imaging and Computer Graphics Theory and Applications (VISIGRAPP): 537-544. DOI: 10.5220/0010878100003124.
Cite this article
Zhang,Y. (2024). Non-Contact Human Body Dimension Estimation Methods Based on Deep Learning: A Critical Analysis. Theoretical and Natural Science,52,214-221.
Data availability
The datasets used and/or analyzed during the current study will be available from the authors upon reasonable request.
Disclaimer/Publisher's Note
The statements, opinions and data contained in all publications are solely those of the individual author(s) and contributor(s) and not of EWA Publishing and/or the editor(s). EWA Publishing and/or the editor(s) disclaim responsibility for any injury to people or property resulting from any ideas, methods, instructions or products referred to in the content.
About volume
Volume title: Proceedings of CONF-MPCS 2024 Workshop: Quantum Machine Learning: Bridging Quantum Physics and Computational Simulations
© 2024 by the author(s). Licensee EWA Publishing, Oxford, UK. This article is an open access article distributed under the terms and
conditions of the Creative Commons Attribution (CC BY) license. Authors who
publish this series agree to the following terms:
1. Authors retain copyright and grant the series right of first publication with the work simultaneously licensed under a Creative Commons
Attribution License that allows others to share the work with an acknowledgment of the work's authorship and initial publication in this
series.
2. Authors are able to enter into separate, additional contractual arrangements for the non-exclusive distribution of the series's published
version of the work (e.g., post it to an institutional repository or publish it in a book), with an acknowledgment of its initial
publication in this series.
3. Authors are permitted and encouraged to post their work online (e.g., in institutional repositories or on their website) prior to and
during the submission process, as it can lead to productive exchanges, as well as earlier and greater citation of published work (See
Open access policy for details).