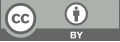
Analysis of modeling nasal narrow space based on visual slam
- 1 School of Mechanical and Electric Engineering, Soochow University, Suzhou, China
* Author to whom correspondence should be addressed.
Abstract
Now, surgeries are becoming more stable, safe, efficient and low-cost. During the surgical treatment of nasal diseases, surgical robotic robotics can help operate accurately and reduce the discomfort after surgery. However, due to the internal space of the nasal cavity being relatively narrow, it is difficult for the nasal surgical robot to contain multiple vision sensors and the monocular camera could not get information about the depth of the 3D objects in the scene, so the existing surgical robots cannot accomplish the three-dimensional modeling about the internal space of nasal cavity well. In practice, doctors still have to analyze pictures from the robotic, which may decrease the efficiency of the surgery and increase the risk to patients. This article designed a SLAM algorithm framework based on a depth estimation network, it can simulate the internal structure of the nasal cavity more accurately through pictures, which come from monocular endoscopic on surgical robotic. The insights gained in this study verify that the method of image segmentation can also make the depth representation of the nasal internal space more accurate and this method may help robots realize their self-position in the narrow area of the nasal cavity, which lays the foundations for the development of fully autonomous surgical robots.
Keywords
Nasal surgery, visual SLAM, depth estimation, narrow space, feature extraction.
[1]. Jianfei Shi & Jia Li. (2018). Efficacy analysis of functional endoscopic sinus surgery in the treatment of chronic rhinosinusitis with nasal polyps. Hebei Medical Journal (03),437-441.
[2]. Xiaoyu Peng. (2017). Master of endoscopic visual SLAM method research in minimally invasive surgery (Dissertation of degree, University of Electronic Science and Technology). Master’s degree https://kns.cnki.net/kcms2/article/abstract?v=gisQO9UvOsYV4fM8BvH 7T7JBrmjZDKUqfRT-FFavuxS98BUi66bdINY6bJvDDJsQadDssVlPZggC0x5Tt0haY7mR QK8nu1N_SxMkknPJNbZGmgm4EF13dJi8BiUkiW2WTTPAg_SwoXHpCuO6L8_aaq8Dh_uFLDkjua_G8yNk-PQJLmOVt7SVhyu5TXFw0Gg4tzo863CT3gc=&uniplatform=NZKPT&language=CHS
[3]. Snavely, N., Seitz, S. M., & Szeliski, R. (2008, June). Skeletal graphs for efficient structure from motion. In 2008 IEEE Conference on Computer Vision and Pattern Recognition (pp. 1-8). IEEE.
[4]. Zhang, R., Tsai, P. S., Cryer, J. E., & Shah, M. (1999). Shape-from-shading: a survey. IEEE transactions on pattern analysis and machine intelligence, 21(8), 690-706.
[5]. Tianteng Bi, Yue Liu, DongDong Weng & YongTian Wang. (2018). A review of single image depth estimation based on supervised learning. Journal of Computer Aided Design and Graphics (08),1383-1393.
[6]. Guizilini, V., Ambrus, R., Pillai, S., Raventos, A., & Gaidon, A. (2020). 3d packing for self-supervised monocular depth estimation. In Proceedings of the IEEE/CVF conference on computer vision and pattern recognition (pp. 2485-2494).
[7]. Xue, F., Cao, J., Zhou, Y., Sheng, F., Wang, Y., & Ming, A. (2021). Boundary-induced and scene-aggregated network for monocular depth prediction. Pattern Recognition, 115, 107901.
[8]. Wenju Li, Mengying Li, Liu Cui, Wanghui Chu, Yi Zhang & Hui Gao. (2023). Monocular depth estimation method based on pyramid split attention network.Journal of Computer Applications(06),1736-1742.
[9]. Laina, I., Rupprecht, C., Belagiannis, V., Tombari, F., & Navab, N. (2016, October). Deeper depth prediction with fully convolutional residual networks. In 2016 Fourth international conference on 3D vision (3DV) (pp. 239-248). IEEE.
[10]. Zhang, H., Zu, K., Lu, J., Zou, Y., & Meng, D. (2021). Epsanet: An efficient pyramid split attention block on convolutional neural network. arXiv preprint arXiv:2105.14447.
[11]. Chen, X., Chen, X., & Zha, Z. J. (2019). Structure-aware residual pyramid network for monocular depth estimation. arXiv preprint arXiv:1907.06023.
Cite this article
Li,C. (2024). Analysis of modeling nasal narrow space based on visual slam. Theoretical and Natural Science,51,121-127.
Data availability
The datasets used and/or analyzed during the current study will be available from the authors upon reasonable request.
Disclaimer/Publisher's Note
The statements, opinions and data contained in all publications are solely those of the individual author(s) and contributor(s) and not of EWA Publishing and/or the editor(s). EWA Publishing and/or the editor(s) disclaim responsibility for any injury to people or property resulting from any ideas, methods, instructions or products referred to in the content.
About volume
Volume title: Proceedings of CONF-MPCS 2024 Workshop: Quantum Machine Learning: Bridging Quantum Physics and Computational Simulations
© 2024 by the author(s). Licensee EWA Publishing, Oxford, UK. This article is an open access article distributed under the terms and
conditions of the Creative Commons Attribution (CC BY) license. Authors who
publish this series agree to the following terms:
1. Authors retain copyright and grant the series right of first publication with the work simultaneously licensed under a Creative Commons
Attribution License that allows others to share the work with an acknowledgment of the work's authorship and initial publication in this
series.
2. Authors are able to enter into separate, additional contractual arrangements for the non-exclusive distribution of the series's published
version of the work (e.g., post it to an institutional repository or publish it in a book), with an acknowledgment of its initial
publication in this series.
3. Authors are permitted and encouraged to post their work online (e.g., in institutional repositories or on their website) prior to and
during the submission process, as it can lead to productive exchanges, as well as earlier and greater citation of published work (See
Open access policy for details).