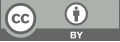
Applying Physics-Informed Extreme Learning Machines to Solve the Euler-Bernoulli Beam Problem
- 1 Shanghai World Foreign Academy
- 2 Imperial College London
* Author to whom correspondence should be addressed.
Abstract
Physics-Informed Neural Networks (PINNs) have been recently utilised to solve forward and backward partial differential equation problems. In this paper, we explore an alternative approach by using Physics-Informed Extreme Learning Machines (PIELM) to address the Euler-Bernoulli beam problem. We experiment with different types of functions combined with various numbers of hidden layers to identify the most effective techniques and conditions for solving partial differential equations with greater efficiency and accuracy. Both the traditional PINN and PIELM methods are applied, and their results are compared. Our findings demonstrate that PIELM offers more efficient computation while maintaining comparable accuracy in the results.
Keywords
Beam theory, partial differential equations, physics-informed neural networks, extreme learning machines
[1]. Lawrence C Evans. Partial differential equations, volume 19. American Mathematical Society, 2022.
[2]. RD Russell and Lawerence F Shampine. A collocation method for boundary value problems. Numerische Mathematik, 19:1–28, 1972.
[3]. Waad Subber and Abhijit Sarkar. A domain decomposition method of stochastic pdes: An iterative solution techniques using a two-level scalable preconditioner. Journal of Computational Physics, 257:298–317, 2014.
[4]. Bernardo Cockburn, George E Karniadakis, and Chi-Wang Shu. The development of discontinuous galerkin methods. In Discontinuous Galerkin methods: theory, computation and applications, pages 3–50. Springer, 2000.
[5]. Randall J LeVeque. Finite difference methods for differential equations. Draft version for use in AMath, 585(6):112, 1998.
[6]. Robert Eymard, Thierry Galloue ̈t, and Raphae`le Herbin. Finite volume methods. Handbook of numerical analysis, 7:713–1018, 2000.
[7]. Carlos A Felippa. Introduction to finite element methods. University of Colorado, 885, 2004.
[8]. Maziar Raissi, Paris Perdikaris, and George E Karniadakis. Physics-informed neural networks: A deep learning framework for solving forward and inverse problems involving nonlinear partial differential equations. Journal of Computational physics, 378:686–707, 2019.
[9]. Yanan Guo, Xiaoqun Cao, Bainian Liu, and Mei Gao. Solving partial differential equations using deep learning and physical constraints. Applied Sciences, 10(17):5917, 2020.
[10]. Lei Yuan, Yi-Qing Ni, Xiang-Yun Deng, and Shuo Hao. A-pinn: Auxiliary physics informed neural networks for forward and inverse problems of nonlinear integro-differential equations. Journal of Computational Physics, 462:111260, 2022.
[11]. SalvatoreCuomo,VincenzoSchianoDiCola,FabioGiampaolo,GianluigiRozza,MaziarRaissi,andFrancescoPiccialli. Scientific machine learning through physics–informed neural networks: Where we are and what’s next. Journal of Scientific Computing, 92(3):88, 2022.
[12]. Vittorio Giavotto, Marco Borri, Paolo Mantegazza, Gianluca Ghiringhelli, V Carmaschi, GC Maffioli, and F Mussi. Anisotropic beam theory and applications. Computers & Structures, 16(1-4):403–413, 1983.
[13]. Zdenek P Baiant and Raymond J Krizek. Journal of the engineering mechanics division. a a, 4:8, 1980.
[14]. Erasmo Carrera, Gaetano Giunta, and Marco Petrolo. Beam structures: classical and advanced theories. John Wiley & Sons, 2011.
[15]. MARK Levinson. A new rectangular beam theory. Journal of Sound and vibration, 74(1):81–87, 1981.
[16]. David Yang Gao. Nonlinear elastic beam theory with application in contact problems and variational approaches. Mechanics Research Communications, 23(1):11–17, 1996.
[17]. Vikas Dwivedi and Balaji Srinivasan. Physics informed extreme learning machine (pielm)–a rapid method for the numerical solution of partial differential equations. Neurocomputing, 391:96–118, 2020.
Cite this article
Pan,S.;Li,H. (2024). Applying Physics-Informed Extreme Learning Machines to Solve the Euler-Bernoulli Beam Problem. Theoretical and Natural Science,53,133-142.
Data availability
The datasets used and/or analyzed during the current study will be available from the authors upon reasonable request.
Disclaimer/Publisher's Note
The statements, opinions and data contained in all publications are solely those of the individual author(s) and contributor(s) and not of EWA Publishing and/or the editor(s). EWA Publishing and/or the editor(s) disclaim responsibility for any injury to people or property resulting from any ideas, methods, instructions or products referred to in the content.
About volume
Volume title: Proceedings of the 2nd International Conference on Applied Physics and Mathematical Modeling
© 2024 by the author(s). Licensee EWA Publishing, Oxford, UK. This article is an open access article distributed under the terms and
conditions of the Creative Commons Attribution (CC BY) license. Authors who
publish this series agree to the following terms:
1. Authors retain copyright and grant the series right of first publication with the work simultaneously licensed under a Creative Commons
Attribution License that allows others to share the work with an acknowledgment of the work's authorship and initial publication in this
series.
2. Authors are able to enter into separate, additional contractual arrangements for the non-exclusive distribution of the series's published
version of the work (e.g., post it to an institutional repository or publish it in a book), with an acknowledgment of its initial
publication in this series.
3. Authors are permitted and encouraged to post their work online (e.g., in institutional repositories or on their website) prior to and
during the submission process, as it can lead to productive exchanges, as well as earlier and greater citation of published work (See
Open access policy for details).