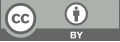
Comparative Analysis of Machine Learning Models for Music Recommendation
- 1 Cornell University, NY, USA
- 2 University of California at Berkeley, CA, USA
- 3 University of Michigan, Ann Arbor, MI, USA
- 4 University of Illinois at Urbana-Champaign, IL, USA
- 5 The George Washington University, DC, USA
- 6 Tsinghua University, Beijing, China
* Author to whom correspondence should be addressed.
Abstract
This study is inspired by the Kaggle competition “WSDM - KKBox’s Music Recommendation Challenge”. The study focuses on doing a comparative study on music recommendation models. Based on the requirements and the given dataset from the Kaggle competition, the problem can be transferred to a classification problem, and therefore, we chose three classification models for the comparative study. The three models are K Nearest Neighbors (KNN), Random Forest, and Light Gradient Boosting Machine (LightGBM). We also did data analysis and data preparation before applying the model and used the handout method and cross-validation method for the validation. For the evaluation, the AUC score is applied to the results and the empirical results show that LightGBM is the best model among these three.
Keywords
Exploratory data analysis (EDA), K Nearest Neighbors (KNN), Random Forest, Light Gradient Boosting Machine (LightGBM), Music Recommendation.
[1]. Ananthram, Priya “EDA of music recommendation system” https://www.kaggle.com/ priyaananthram/eda-of-music-recommendation-system
[2]. Belgiu, Mariana, and Lucian Drăguţ.‘‘Random forest in remote sensing: A review of applications and future directions.’’ ISPRS journal of photogrammetry and remote sensing 114 (2016): 24-31.
[3]. Bobadilla, Jesús, et al. “Recommender systems survey.” Knowledge-based systems 46 (2013): 109-132.
[4]. Fawcett, Tom. “An introduction to ROC analysis.” Pattern recognition letters 27.8 (2006): 861-874.
[5]. Guo, Gongde, et al. “KNN model-based approach in classification.” OTM Confederated International Conferences “On the Move to Meaningful Internet Systems”. Springer, Berlin, Heidelberg, 2003.
[6]. Jiang, Jiawei, et al. “Dimboost: Boosting gradient boosting decision tree to higher dimensions.” Proceedings of the 2018 International Conference on Management of Data. 2018.
[7]. Ke, Guolin, et al. “Lightgbm: A highly efficient gradient boosting decision tree.” Advances in neural information processing systems 30 (2017): 3146-3154.
[8]. Khandelwal, Pranjal. “Which algorithm takes the crown: Light GBM vs XGBOOST?” https://www.analyticsvidhya.com/blog/2017/06/which-algorithm-takes-the-crown-light-gbm-vs-xgboost/
[9]. KKBOX. “WSDM - KKBox's Music Recommendation Challenge” https://www. kaggle .com/c/kkbox-music-recommendation-challengef
[10]. lystdo. “A brief introduction to the 1st place solution” https://www.kaggle.com/c/kkbox-music-recommendation-challenge/discussion/45942
[11]. Pedregosa et al. “OneHotEncoder” https://scikit-learn.org/stable/modules/generated/sklearn. preprocessing.OneHotEncoder.html
[12]. Pedregosa et al. “TruncatedSVD” https://scikit-learn.org/stable/modules/generated/sklearn. decomposition.TruncatedSVD.html
[13]. Vozalis, Manolis G., and Konstantinos G. Margaritis. “Using SVD and demographic data for the enhancement of generalized collaborative filtering.” Information Sciences 177.15 (2007): 3017-3037.
Cite this article
Cheng,X.;Liu,K.;Hu,X.;Liu,T.;Che,C.;Zhu,C. (2024). Comparative Analysis of Machine Learning Models for Music Recommendation. Theoretical and Natural Science,53,249-254.
Data availability
The datasets used and/or analyzed during the current study will be available from the authors upon reasonable request.
Disclaimer/Publisher's Note
The statements, opinions and data contained in all publications are solely those of the individual author(s) and contributor(s) and not of EWA Publishing and/or the editor(s). EWA Publishing and/or the editor(s) disclaim responsibility for any injury to people or property resulting from any ideas, methods, instructions or products referred to in the content.
About volume
Volume title: Proceedings of the 2nd International Conference on Applied Physics and Mathematical Modeling
© 2024 by the author(s). Licensee EWA Publishing, Oxford, UK. This article is an open access article distributed under the terms and
conditions of the Creative Commons Attribution (CC BY) license. Authors who
publish this series agree to the following terms:
1. Authors retain copyright and grant the series right of first publication with the work simultaneously licensed under a Creative Commons
Attribution License that allows others to share the work with an acknowledgment of the work's authorship and initial publication in this
series.
2. Authors are able to enter into separate, additional contractual arrangements for the non-exclusive distribution of the series's published
version of the work (e.g., post it to an institutional repository or publish it in a book), with an acknowledgment of its initial
publication in this series.
3. Authors are permitted and encouraged to post their work online (e.g., in institutional repositories or on their website) prior to and
during the submission process, as it can lead to productive exchanges, as well as earlier and greater citation of published work (See
Open access policy for details).