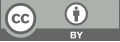
Comparative analysis of machine learning in diagnosing Parkinson's: Utilizing vocal characteristics
- 1 Hillsborough High School
* Author to whom correspondence should be addressed.
Abstract
Parkinson’s disease is a neurodegenerative disorder that affects movement. Diagnosing Parkinson’s disease has traditionally involved clinical assessments by neurologists, and this practice still persists today to a significant extent. However, clinical assessments can be prone to subjectivity. In this study, a comprehensive predictive modeling approach was undertaken, employing nine dis¬tinct machine learning algorithms and six different model evaluation metrics to identify the best per¬forming algorithms. The findings reveal that, using only 12 vocal characteristics, KNeighborsClassfier (KNC), MLPClassifier (MLP), and XGBClassifier (XGBC) achieved the highest score of 0.87. This score is generally considered very good, indicating that the model is robust and possesses strong predictive power. This study marks a crucial initial step in leveraging machine learning techniques for more effective and potentially more accurate diagnosis of Parkinson’s disease based on patients’ vocal characteristics.
Keywords
Parkinson’s disease, machine learning, vocal characteristics.
[1]. Pagano G, Niccolini F, Politis M. Imaging in Parkinson’s disease. Clin Med (Lond), 2016,16(4): 371-5.
[2]. Rusz J, Tykalová T, Novotný M, et al. Distinct patterns of speech disorder in early-onset and late-onset de novo Parkinson’s disease. NPJ, 2021.
[3]. Kaggle, Parkinson’s Disease Dataset. (2021), Available online at: https: //www.kaggle.com/datasets/gargmanas/parkinsonsdataset
[4]. Naidu G, Zuva T, Sibanda, E. M. A Review of Evaluation Metrics in Machine Learning Algorithms In Artificial Intelligence Application in Networks and Systems. Springer, 2023.
[5]. Iqbal H. Machine Learning: Algorithms, Real-World Applications and Research Directions. SN COMPUT. SCI, 2021, 2 (160).
[6]. Bonaccorso G. Machine Learning Algorithms. Packt Publishing, 2017
[7]. Song YY, Lu Y. Decision tree methods: Applications for classification and prediction. Shanghai Arch Psychiatry, 2015, 27(2): 130-5.
[8]. McCarty DA, Kim HW, Lee HK. Evaluation of Light Gradient Boosted Machine Learning Technique in Large Scale Land Use and Land Cover Classification. Environments, 2020, 7(10): 84.
[9]. Huang S, Cai N, Pacheco PP, Narrandes S, Wang Y, Xu W. Applications of Support Vector Machine (SVM) Learning in Cancer Genomics. Cancer Genomics Proteomics, 201, 15(1): 41-51.
[10]. Vidhya, 12 Important Model Evaluation Metrics for Machine Learning Everyone Should Know (Updated 2023). (2023), Available online at: https: //www.analyticsvidhya.com/ blog/2019/08/11-important-model-evaluation-error-metrics/
Cite this article
Yu,Y. (2024). Comparative analysis of machine learning in diagnosing Parkinson's: Utilizing vocal characteristics . Theoretical and Natural Science,40,1-8.
Data availability
The datasets used and/or analyzed during the current study will be available from the authors upon reasonable request.
Disclaimer/Publisher's Note
The statements, opinions and data contained in all publications are solely those of the individual author(s) and contributor(s) and not of EWA Publishing and/or the editor(s). EWA Publishing and/or the editor(s) disclaim responsibility for any injury to people or property resulting from any ideas, methods, instructions or products referred to in the content.
About volume
Volume title: Proceedings of the 4th International Conference on Biological Engineering and Medical Science
© 2024 by the author(s). Licensee EWA Publishing, Oxford, UK. This article is an open access article distributed under the terms and
conditions of the Creative Commons Attribution (CC BY) license. Authors who
publish this series agree to the following terms:
1. Authors retain copyright and grant the series right of first publication with the work simultaneously licensed under a Creative Commons
Attribution License that allows others to share the work with an acknowledgment of the work's authorship and initial publication in this
series.
2. Authors are able to enter into separate, additional contractual arrangements for the non-exclusive distribution of the series's published
version of the work (e.g., post it to an institutional repository or publish it in a book), with an acknowledgment of its initial
publication in this series.
3. Authors are permitted and encouraged to post their work online (e.g., in institutional repositories or on their website) prior to and
during the submission process, as it can lead to productive exchanges, as well as earlier and greater citation of published work (See
Open access policy for details).