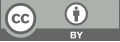
ProteinBERT Algorithms: Applications in Antimicrobial Peptides Classification, Intrinsically Disordered Protein Prediction, and Toxicity Analysis
- 1 Xi’an Jiaotong-Liverpool University, Suzhou, China
* Author to whom correspondence should be addressed.
Abstract
The burgeoning field of computational biology has been markedly enhanced by the integration of advanced machine learning models capable of tackling intricate protein-related challenges. ProteinBERT, a transformer-based deep learning algorithm, has emerged as a formidable tool in deciphering complex patterns within protein sequences. This study delves into ProteinBERT's robust application across three pivotal domains: antimicrobial peptide (AMP) classification, intrinsically disordered protein (IDP) prediction, and protein toxicity prediction. Leveraging domain-specific datasets alongside sophisticated evaluation metrics, ProteinBERT has shown superior performance, surpassing both traditional models and other contemporary deep learning approaches in these areas. The analysis reveals that ProteinBERT not only accurately classifies AMPs, effectively predicts IDP configurations, and reliably forecasts protein toxicity but also sets new benchmarks in the precision of computational predictions. This research underscores the significant capabilities of ProteinBERT and discusses prospective enhancements that could refine its utility in computational protein analysis, aiming to push the boundaries of current methodologies and foster innovations in protein research.
Keywords
Antimicrobial peptides, intrinsically disordered proteins, protein toxicity
[1]. Brandes, N., Ofer, D., Peleg, Y., Rappoport, N., & Linial, M. (2022). ProteinBERT: A universal deep-learning model of protein sequence and function. Bioinformatics, 38(8), 2102–2110.
[2]. Gene Ontology Consortium. (2004). The Gene Ontology (GO) database and informatics resource. Nucleic acids research, 32(suppl_1), D258-D261.
[3]. Oldfield, C. J., & Dunker, A. K. (2014). Intrinsically disordered proteins and intrinsically disordered protein regions. Annual review of biochemistry, 83(1), 553-584.
[4]. Jain, A., & Kihara, D. (2019). NNTox: Gene Ontology-Based Protein Toxicity Prediction Using Neural Network. Scientific Reports, 9(1), 17923.
[5]. Lu, J., Zhang, H., Jin, C., & Quan, X. (2023). A Novel Classification Method for Antimicrobial Peptides Based on ProteinBERT. 2023 42nd Chinese Control Conference (CCC), 8437–8442.
[6]. Brandes, N., Ofer, D., Peleg, Y., Rappoport, N., & Linial, M. (2022). ProteinBERT: a universal deep-learning model of protein sequence and function. Bioinformatics, 38(8), 2102-2110.
[7]. Zhu, X., Guo, C., Feng, H., Huang, Y., Feng, Y., Wang, X., & Wang, R. (2024). A Review of Key Technologies for Emotion Analysis Using Multimodal Information. Cognitive Computation, 1-27.
[8]. Wang R., Zhu J., Wang S., Wang T., Huang J., Zhu X. Multi-modal emotion recognition using tensor decomposition fusion and self-supervised multi-tasking. International Journal of Multimedia Information Retrieval, 2024, 13(4): 39.
[9]. Stone, J. D., Tabrizi, H. B., & Shamsuddin, R. (2023). Enhancing ProteinBERT: Integrating Intrinsically Disordered Proteins for Comprehensive Proteomic Predictions. 2023 IEEE International Conference on Bioinformatics and Biomedicine (BIBM), 3824–3831.
[10]. Vaswani, A. (2017). Attention is all you need. Advances in Neural Information Processing Systems.
[11]. Vig, J., Madani, A., Varshney, L. R., Xiong, C., Socher, R., & Rajani, N. F. (2021). BERTology Meets Biology: Interpreting Attention in Protein Language Models (arXiv:2006.15222).
[12]. Morozov, V., Rodrigues, C. H., & Ascher, D. B. (2023). CSM-Toxin: a web-server for predicting protein toxicity. Pharmaceutics, 15(2), 431.
Cite this article
Li,X. (2024). ProteinBERT Algorithms: Applications in Antimicrobial Peptides Classification, Intrinsically Disordered Protein Prediction, and Toxicity Analysis. Theoretical and Natural Science,71,99-107.
Data availability
The datasets used and/or analyzed during the current study will be available from the authors upon reasonable request.
Disclaimer/Publisher's Note
The statements, opinions and data contained in all publications are solely those of the individual author(s) and contributor(s) and not of EWA Publishing and/or the editor(s). EWA Publishing and/or the editor(s) disclaim responsibility for any injury to people or property resulting from any ideas, methods, instructions or products referred to in the content.
About volume
Volume title: Proceedings of ICBioMed 2024 Workshop: Computational Proteomics in Drug Discovery and Development from Medicinal Plants
© 2024 by the author(s). Licensee EWA Publishing, Oxford, UK. This article is an open access article distributed under the terms and
conditions of the Creative Commons Attribution (CC BY) license. Authors who
publish this series agree to the following terms:
1. Authors retain copyright and grant the series right of first publication with the work simultaneously licensed under a Creative Commons
Attribution License that allows others to share the work with an acknowledgment of the work's authorship and initial publication in this
series.
2. Authors are able to enter into separate, additional contractual arrangements for the non-exclusive distribution of the series's published
version of the work (e.g., post it to an institutional repository or publish it in a book), with an acknowledgment of its initial
publication in this series.
3. Authors are permitted and encouraged to post their work online (e.g., in institutional repositories or on their website) prior to and
during the submission process, as it can lead to productive exchanges, as well as earlier and greater citation of published work (See
Open access policy for details).