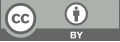
Temporal, Spatial Characteristics and Prediction of Precipitation in Guangzhou Based on the SARIMA Model
- 1 Henan University, Kaifeng, China
* Author to whom correspondence should be addressed.
Abstract
This article obtains nearly 18 years of precipitation data for Guangzhou from the National Centers for Environmental Information (NCEI) under the National Oceanic and Atmospheric Administration (NOAA) and conducts visualization analysis and modeling predictions using R software.Using the classic SARIMA model (the ARIMA model with seasonal components) as well as regression and residual ARIMA models to build and simulate the precipitation series.We also used Basic-bootstrap prediction, which involves resampling the residuals of the fitted model with replacement, using historical values to predict the future and obtain a model parameter, as well as Full-bootstrap prediction (which assumes that the residuals have uncertainty and that the estimated model coefficients themselves also have uncertainty).Therefore, not only are reasonable model residuals obtained through the bootstrap method, but multiple model parameters are also derived from this method to determine more reasonable model parameters, which are then used to forecast the next 12 periods for the fitted model. Comparing the results of the four prediction models, the Full-bootstrap model under the regression model showed the best predictive performance. The study found that there is no significant trend in precipitation changes over time, but short-term fluctuations still exist. Although some extreme values appeared, they remain within a predictable range.Therefore, the relevant city government departments prone to extreme rainfall can make reasonable adjustments to drainage facilities based on local precipitation forecasts
Keywords
SARIMA model, precipitation, bootstrap method, seasonality, regression and residual ARIMA model, R language
[1]. Lan,Y., Shan D. (2024). Analysis of Rainstorm Disasters and Research on Urban Flood Prevention and Disaster Relief Strategies. Yellow River Water Conservancy Committee Shandong Yellow River Administration Bureau; Jinan Yellow River Administration Bureau Zhangqiu Yellow River Administration Bureau, 3.
[2]. Hongtao,L. (2014). Research on Combined Models for Rainfall Prediction in Irrigation Areas. Northeast Agricultural University.
[3]. Rezaiy,R., Shabri,A. (2024. )An innovative hybrid W-EEMD-ARIMA model for drought forecasting using the standardized precipitation index. Natural Hazards,1-30.
[4]. Cheng Maolin. (2015). Regression Prediction Model and Application Based on the Growth Curve of Trigonometric Functions [J]. Journal of Suzhou University of Science and Technology (Natural Science Edition), 32(04): 4-8.
[5]. Doko T., Firmin, Qazi H. (2023). On bootstrapping tests of equal forecast accuracy for nested models. Journal of Forecasting 42.7: 1844-1864.
[6]. Perera, I., Silvapulle, MJ. (2021). Bootstrap based probability forecasting in multiplicative error models. Journal of Econometrics 221.1: 1-24.
[7]. Tahyudin, I., Wahyudi,R., Nambo,H. (2022). SARIMA-LSTM combination for COVID-19 case modeling. IIUM Engineering Journal 23.2: 171-182.
[8]. Davino, C., Romano, R., Vistocco, D. (2022). Handling multicollinearity in quantile regression through the use of principal component regression. Metron, 80(2), 153-174.
[9]. Gimeno, L., Sorí, R., Vazquez, M., Stojanovic, M., Algarra, I., Eiras‐Barca, J., ... & Nieto, R. (2022). Extreme precipitation events. Wiley Interdisciplinary Reviews: Water, 9(6), e1611.
[10]. Yang, L., Franzke, C. L., & Duan, W. (2023). Evaluation and projections of extreme precipitation using a spatial extremes framework. International Journal of Climatology, 43(7), 3453-3475.
Cite this article
Yang,R. (2025). Temporal, Spatial Characteristics and Prediction of Precipitation in Guangzhou Based on the SARIMA Model. Theoretical and Natural Science,87,52-61.
Data availability
The datasets used and/or analyzed during the current study will be available from the authors upon reasonable request.
Disclaimer/Publisher's Note
The statements, opinions and data contained in all publications are solely those of the individual author(s) and contributor(s) and not of EWA Publishing and/or the editor(s). EWA Publishing and/or the editor(s) disclaim responsibility for any injury to people or property resulting from any ideas, methods, instructions or products referred to in the content.
About volume
Volume title: Proceedings of the 4th International Conference on Computing Innovation and Applied Physics
© 2024 by the author(s). Licensee EWA Publishing, Oxford, UK. This article is an open access article distributed under the terms and
conditions of the Creative Commons Attribution (CC BY) license. Authors who
publish this series agree to the following terms:
1. Authors retain copyright and grant the series right of first publication with the work simultaneously licensed under a Creative Commons
Attribution License that allows others to share the work with an acknowledgment of the work's authorship and initial publication in this
series.
2. Authors are able to enter into separate, additional contractual arrangements for the non-exclusive distribution of the series's published
version of the work (e.g., post it to an institutional repository or publish it in a book), with an acknowledgment of its initial
publication in this series.
3. Authors are permitted and encouraged to post their work online (e.g., in institutional repositories or on their website) prior to and
during the submission process, as it can lead to productive exchanges, as well as earlier and greater citation of published work (See
Open access policy for details).