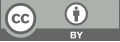
Research Advanced in Speech Emotion Recognition based on Deep Learning
- 1 New York University
* Author to whom correspondence should be addressed.
Abstract
Speech Emotion Recognition (SER)’s burgeoning significance within intelligent systems is underscored by its transformative impact across various fields, from human-computer interaction, and virtual assistants to mental health monitoring. Over the rapid development of this technology in the past two decades, studies have continuously confronted and overcome kinds of real-world challenges, such as data scarcity, environmental noise, and cross-language differences. This survey focuses on recent innovations in SER, particularly deep learning architectures, and synthetic data augmentation, and addresses recent developments in cross-domain and multimodal SER techniques, which have expanded the applicability of SER to more diverse datasets.
Keywords
Speech emotion recognition, deep learning, data augmentation
[1]. Higginbotham,Adam “Welcome to Rosalind Picard’s touchy- feelyworld of empathictech.” URL:http://www.wired.co.uklmagazine/archive/2012 /11(features/emotion-machines.)
[2]. H. Zhao, N. Ye and R. Wang, “A Survey on Automatic Emotion Recognition Using Audio Big Data and Deep Learning Architectures,” 2018 IEEE 4th International Conference on Big Data Security on Cloud (BigDataSecurity), IEEE International Conference on High Performance and Smart Computing, (HPSC) and IEEE International Conference on Intelligent Data and Security (IDS), Omaha, NE, USA, 2018, pp. 139142.
[3]. Chang-Hyun Park, Dong-Wook Lee and Kwee-Bo Sim, “Emotion recognition of speech based on RNN,” Proceedings. International Conference on Machine Learning and Cybernetics, Beijing, China, 2002, pp. 22102213 vol.4.
[4]. T. M. Wani, T. S. Gunawan, S. A. A. Qadri, M. Kartiwi and E. Ambikairajah, “A Comprehensive Review of Speech Emotion Recognition Systems,” in IEEE Access, vol. 9, pp. 47795-47814, 2021.
[5]. L. Zhang, Y. Wang, J. Du and X. Wang, “CNN-BiGRU Speech Emotion Recognition Based on Attention Mechanism,” 2023 2nd International Conference on Artificial Intelligence and Intelligent Information Processing (AIIIP), Hangzhou, China, 2023, pp. 85-89.
[6]. D. Chandola, E. Altarawneh, M. Jenkin and M. Papagelis, “SERC-GCN: Speech Emotion Recognition In Conversation Using Graph Convolutional Networks,” ICASSP 2024 - 2024 IEEE International Conference on Acoustics, Speech and Signal Processing (ICASSP), Seoul, Korea, Republic of, 2024, pp. 76-80.
[7]. I. R. Ulgen, Z. Du, C. Busso and B. Sisman, ”Revealing Emotional Clusters in Speaker Embeddings: A Contrastive Learning Strategy for Speech Emotion Recognition,” ICASSP 2024 - 2024 IEEE International Conference on Acoustics, Speech and Signal Processing (ICASSP), Seoul, Korea, Republic of, 2024, pp. 12081-12085.
[8]. Alexei Baevski, Henry Zhou, Abdelrahman Mohamed, and Michael Auli, “Wav2vec 2.0: A framework for self-supervised learning of speech representations,” in Proceedings of the 34th International Con- ference on Neural Information Processing Systems, Red Hook, NY, USA, 2020, NIPS’20, Curran Associates Inc.
[9]. Livingstone, S. R., Russo, F. A. (2018). The Ryerson Audio-Visual Database of Emotional Speech and Song (RAVDESS): A dynamic, multimodal set of facial and vocal expressions in North American English. PLoS ONE, 13(5), e0196391.
[10]. Busso, C., Bulut, M., Lee, C., Kazemzadeh, A., Mower, E., Kim, S., Chang, J. N., Lee, S., Narayanan, S. S. (2008). IEMOCAP: interactive emotional dyadic motion capture database. Language Resources and Evaluation, 42(4), 335–359.
[11]. K. M. Ibrahim, A. Perzo and S. Leglaive, “Towards Improving Speech Emotion Recognition Using Synthetic Data Augmentation from Emotion Conversion,” ICASSP 2024 - 2024 IEEE International Conference on Acoustics, Speech and Signal Processing (ICASSP), Seoul, Korea, Republic of, 2024, pp. 10636-10640.
[12]. X. Cai, D. Dai, Z. Wu, X. Li, J. Li and H. Meng, “Emotion Controllable Speech Synthesis Using Emotion-Unlabeled Dataset with the Assistance of Cross-Domain Speech Emotion Recognition,” ICASSP 2021- 2021 IEEE International Conference on Acoustics, Speech and Signal Processing (ICASSP), Toronto, ON, Canada, 2021, pp. 5734-5738.
[13]. X.-S. Yang, S. F. Chien, and T. O. Ting, “Bio-Inspired Computing,” Morgan Kaufmann, 2015. DOI: https://doi.org/10.1016/C2014-0-00501-1.
[14]. R. Lotfidereshgi and P. Gournay, ”Biologically inspired speech emotion recognition,” 2017 IEEE International Conference on Acoustics, Speech and Signal Processing (ICASSP), New Orleans, LA, USA, 2017, pp. 5135-5139.
[15]. Y. Li, “Enhancing Speech Emotion Recognition for Real-World Applications via ASR Integration,” 2023 11th International Conference on Affective Computing and Intelligent Interaction Workshops and Demos (ACIIW), Cambridge, MA, USA, 2023, pp. 1-5.
[16]. M. S. Nair and D. P. Gopinath, “Transfer learning for Speech Based Emotion Recognition,” 2022 IEEE International Conference on Signal Processing, Informatics, Communication and Energy Systems (SPICES), THIRUVANANTHAPURAM, India, 2022, pp. 559-564.
[17]. Swapna Mol George, P. Muhamed Ilyas, A review on speech emotion recognition: A survey, recent advances, challenges, and the influence of noise, Neurocomputing, Volume 568, 2024, 127015, ISSN 0925-2312.
[18]. M. Xu, F. Zhang and W. Zhang, “Head Fusion: Improving the Accuracy and Robustness of Speech Emotion Recognition on the IEMOCAP and RAVDESS Dataset,” in IEEE Access, vol. 9, pp. 74539-74549, 2021.
[19]. Ainurrochman and U. L. Yuhana, “Improving Performance of Speech Emotion Recognition Application using Extreme Learning Machine and Utterance-level,” 2024 International Seminar on Intelligent Technology and Its Applications (ISITIA), Mataram, Indonesia, 2024, pp. 466-470.
Cite this article
He,Z. (2025). Research Advanced in Speech Emotion Recognition based on Deep Learning . Theoretical and Natural Science,86,45-52.
Data availability
The datasets used and/or analyzed during the current study will be available from the authors upon reasonable request.
Disclaimer/Publisher's Note
The statements, opinions and data contained in all publications are solely those of the individual author(s) and contributor(s) and not of EWA Publishing and/or the editor(s). EWA Publishing and/or the editor(s) disclaim responsibility for any injury to people or property resulting from any ideas, methods, instructions or products referred to in the content.
About volume
Volume title: Proceedings of the 4th International Conference on Computing Innovation and Applied Physics
© 2024 by the author(s). Licensee EWA Publishing, Oxford, UK. This article is an open access article distributed under the terms and
conditions of the Creative Commons Attribution (CC BY) license. Authors who
publish this series agree to the following terms:
1. Authors retain copyright and grant the series right of first publication with the work simultaneously licensed under a Creative Commons
Attribution License that allows others to share the work with an acknowledgment of the work's authorship and initial publication in this
series.
2. Authors are able to enter into separate, additional contractual arrangements for the non-exclusive distribution of the series's published
version of the work (e.g., post it to an institutional repository or publish it in a book), with an acknowledgment of its initial
publication in this series.
3. Authors are permitted and encouraged to post their work online (e.g., in institutional repositories or on their website) prior to and
during the submission process, as it can lead to productive exchanges, as well as earlier and greater citation of published work (See
Open access policy for details).