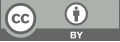
Research Advanced in Image Recognition under Autonomous Driving Scene Based on Deep Learning
- 1 Ira A. Fulton Schools of Engineering, Arizona State University, AZ, USA
* Author to whom correspondence should be addressed.
Abstract
Image recognition has always been a fundamental research task in the computer vision community, aimed at identifying the categories of objects in images and has been widely used in many fields, especially in autonomous driving. Early image recognition technologies were mostly based on machine learning, and their recognition speed and accuracy could not meet the application requirements of complex autonomous driving scenarios. With the great success of convolutional neural networks, image recognition technology based on deep learning has attracted increasing research interest. Taking the autonomous driving scenario as an example, this article introduces the latest research progress of image recognition technology, including representative methods and their basic pipelines. In addition, this paper also introduces the commonly used dataset in image recognition and discusses the existing problems of image recognition in autonomous driving tasks. Finally, this paper looks forward to the future development directions of this field, hoping bring some new insight to advance the further development of image recognition under autonomous driving scene.
Keywords
Image recognition, autonomous driving, deep learning
[1]. Fujiyoshi, H., Hirakawa, T., & Yamashita, T. (2019). Deep learning-based image recognition for autonomous driving. IATSS Research, 43(4), 244-252.
[2]. Zhao, J., Zhao, W., Deng, B., Wang, Z., Zhang, F., Zheng, W., Cao, W., Nan, J., Lian, Y., & Burke, A. F. (2024). Autonomous driving system: A comprehensive survey. Expert Systems with Applications, 242, 122836.1-122836.27.
[3]. Lai, Y. (2019). A Comparison of Traditional Machine Learning and Deep Learning in Image Recognition. Journal of Physics. Conference Series, 1314(1), 12148.
[4]. Yu, W., & Ren, P. (2021). Vehicle and pedestrian target detection in auto driving scene. Journal of Physics: Conference Series, 2132(1), 12013.
[5]. Padmavathi, B., Dhivya, S., Datchanamoorthy, K., Banu, A. K., & Karthikeyan, S. M. (2024). Lane and Traffic Sign Detection in Self-Driving Cars using Deep Learning. International Journal of Vehicle Structures and Systems, 16(1), 45-49.
[6]. Geiger, A., Lenz, P., & Urtasun, R. (2012). Are we ready for autonomous driving? The KITTI vision benchmark suite. 2012 IEEE Conference on Computer Vision and Pattern Recognition, 3354-3361.
[7]. Cordts, M., Omran, M., Ramos, S., Rehfeld, T., Enzweiler, M., Benenson, R., Franke, U., Roth, S., & Schiele, B. (2016). The Cityscapes Dataset for Semantic Urban Scene Understanding. 2016 IEEE Conference on Computer Vision and Pattern Recognition (CVPR), 3213–3223.
[8]. Sun, P. , Kretzschmar, H. , Dotiwalla, X. , Chouard, A. , Patnaik, V. , & Tsui, P. , et al. (2020). Scalability in Perception for Autonomous Driving: Waymo Open Dataset. 2020 IEEE/CVF Conference on Computer Vision and Pattern Recognition (CVPR), 2443-2451.
[9]. Zhang, Y., Carballo, A., Yang, H., & Takeda, K. (2023). Perception and sensing for autonomous vehicles under adverse weather conditions: A survey. ISPRS Journal of Photogrammetry and Remote Sensing, 196, 146-177.
[10]. Hataba, M., Sherif, A., Mahmoud, M., Abdallah, M., & Alasmary, W. (2022). Security and Privacy Issues in Autonomous Vehicles: A Layer-Based Survey. IEEE Open Journal of the Communications Society, 3, 811–829.
Cite this article
Zhu,Z. (2025). Research Advanced in Image Recognition under Autonomous Driving Scene Based on Deep Learning. Theoretical and Natural Science,86,53-59.
Data availability
The datasets used and/or analyzed during the current study will be available from the authors upon reasonable request.
Disclaimer/Publisher's Note
The statements, opinions and data contained in all publications are solely those of the individual author(s) and contributor(s) and not of EWA Publishing and/or the editor(s). EWA Publishing and/or the editor(s) disclaim responsibility for any injury to people or property resulting from any ideas, methods, instructions or products referred to in the content.
About volume
Volume title: Proceedings of the 4th International Conference on Computing Innovation and Applied Physics
© 2024 by the author(s). Licensee EWA Publishing, Oxford, UK. This article is an open access article distributed under the terms and
conditions of the Creative Commons Attribution (CC BY) license. Authors who
publish this series agree to the following terms:
1. Authors retain copyright and grant the series right of first publication with the work simultaneously licensed under a Creative Commons
Attribution License that allows others to share the work with an acknowledgment of the work's authorship and initial publication in this
series.
2. Authors are able to enter into separate, additional contractual arrangements for the non-exclusive distribution of the series's published
version of the work (e.g., post it to an institutional repository or publish it in a book), with an acknowledgment of its initial
publication in this series.
3. Authors are permitted and encouraged to post their work online (e.g., in institutional repositories or on their website) prior to and
during the submission process, as it can lead to productive exchanges, as well as earlier and greater citation of published work (See
Open access policy for details).