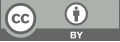
The Evolution of YOLO: from YOLOv1 to YOLOv11 with a Focus on YOLOv7's Innovations in Object Detection
- 1 Courant Institute of Mathematical Sciences, New York University, NY, USA
* Author to whom correspondence should be addressed.
Abstract
Throughout the evolution of You Only Look Once (YOLO) series, staring from base YOLO to latest YOLOv11, each version takes advantages of different techniques and mechanism, incorporating innovations that enhance object detection capabilities by improving both speed and accuracy. From introduction of anchor boxes in YOLOv2 to multi-scale predictions in YOLOv3 and Cross-Stage Partial Networks in YOLOv4, each iteration has brought unique improvements. In YOLOv7, two major advancements, Extended Efficient Layer Aggregation Network and Planned Re-parameterized Convolution, were introduced to address challenges in feature aggregation and parameter utilization, while maintaining optimal gradient flow. Additionally, advanced label assignment strategies, such as lead head guided label assigner and coarse-to-fine label assigner, further improve learning efficiency. These innovations enable YOLOv7 to set new standards in object detection, especially for applications in autonomous driving, video surveillance, medical imaging, and beyond
Keywords
Object Detection, YOLO series, YOLOv7
[1]. Ren, S., He, K., Girshick, R., & Sun, J. (2016). Faster R-CNN: Towards real-time object detection with region proposal networks. IEEE transactions on pattern analysis and machine intelligence, 39(6), 1137-1149.
[2]. Carion, N., Massa, F., Synnaeve, G., Usunier, N., Kirillov, A., & Zagoruyko, S. (2020, August). End-to-end object detection with transformers. In European conference on computer vision (pp. 213-229). Cham: Springer International Publishing.
[3]. Redmon, J. (2016). You only look once: Unified, real-time object detection. In Proceedings of the IEEE conference on computer vision and pattern recognition.
[4]. Girshick, R., Donahue, J., Darrell, T., & Malik, J. (2014). Rich feature hierarchies for accurate object detection and semantic segmentation. In Proceedings of the IEEE conference on computer vision and pattern recognition (pp. 580-587).
[5]. Szegedy, C., Liu, W., Jia, Y., Sermanet, P., Reed, S., Anguelov, D., ... & Rabinovich, A. (2015). Going deeper with convolutions. In Proceedings of the IEEE conference on computer vision and pattern recognition (pp. 1-9).
[6]. Everingham, M., Eslami, S. A., Van Gool, L., Williams, C. K., Winn, J., & Zisserman, A. (2015). The pascal visual object classes challenge: A retrospective. International journal of computer vision, 111, 98-136.
[7]. Ren, S., He, K., Girshick, R., & Sun, J. (2016). Faster R-CNN: Towards real-time object detection with region proposal networks. IEEE transactions on pattern analysis and machine intelligence, 39(6), 1137-1149.
[8]. Redmon, J., & Farhadi, A. (2017). YOLO9000: better, faster, stronger. In Proceedings of the IEEE conference on computer vision and pattern recognition (pp. 7263-7271).
[9]. Farhadi, A., & Redmon, J. (2018, June). Yolov3: An incremental improvement. In Computer vision and pattern recognition (Vol. 1804, pp. 1-6). Berlin/Heidelberg, Germany: Springer.
[10]. Srivastava, S., Divekar, A. V., Anilkumar, C., Naik, I., Kulkarni, V., & Pattabiraman, V. (2021). Comparative analysis of deep learning image detection algorithms. Journal of Big data, 8(1), 66.
[11]. Bochkovskiy, A., Wang, C. Y., & Liao, H. Y. M. (2020). Yolov4: Optimal speed and accuracy of object detection. arXiv preprint arXiv:2004.10934.
[12]. Li, C., Li, L., Jiang, H., Weng, K., Geng, Y., Li, L., ... & Wei, X. (2022). YOLOv6: A single-stage object detection framework for industrial applications. arXiv preprint arXiv:2209.02976.
[13]. Wang, C. Y., Bochkovskiy, A., & Liao, H. Y. M. (2023). YOLOv7: Trainable bag-of-freebies sets new state-of-the-art for real-time object detectors. In Proceedings of the IEEE/CVF conference on computer vision and pattern recognition (pp. 7464-7475).
[14]. Yaseen, M. (2024). What is YOLOv8: An In-Depth Exploration of the Internal Features of the Next-Generation Object Detector. arXiv preprint arXiv:2408.15857.
[15]. Wang, C. Y., Yeh, I. H., & Liao, H. Y. M. (2024). Yolov9: Learning what you want to learn using programmable gradient information. arXiv preprint arXiv:2402.13616.
[16]. Wang, A., Chen, H., Liu, L., Chen, K., Lin, Z., Han, J., & Ding, G. (2024). Yolov10: Real-time end-to-end object detection. arXiv preprint arXiv:2405.14458.
[17]. Terven, J., Córdova-Esparza, D. M., & Romero-González, J. A. (2023). A comprehensive review of yolo architectures in computer vision: From yolov1 to yolov8 and yolo-nas. Machine Learning and Knowledge Extraction, 5(4), 1680-1716.
[18]. Wang, C. Y., & Liao, H. Y. M. (2024). YOLOv1 to YOLOv10: The fastest and most accurate real-time object detection systems. arXiv preprint arXiv:2408.09332.
[19]. Wang, C. Y., Liao, H. Y. M., & Yeh, I. H. (2022). Designing network design strategies through gradient path analysis. arXiv preprint arXiv:2211.04800.
[20]. Ding, X., Zhang, X., Ma, N., Han, J., Ding, G., & Sun, J. (2021). Repvgg: Making vgg-style convnets great again. In Proceedings of the IEEE/CVF conference on computer vision and pattern recognition (pp. 13733-13742).
[21]. He, K., Zhang, X., Ren, S., & Sun, J. (2016). Deep residual learning for image recognition. In Proceedings of the IEEE conference on computer vision and pattern recognition (pp. 770-778).
[22]. Huang, G., Liu, Z., Van Der Maaten, L., & Weinberger, K. Q. (2017). Densely connected convolutional networks. In Proceedings of the IEEE conference on computer vision and pattern recognition (pp. 4700-4708).
[23]. Shigematsu, K. (2023). Small Bird Detection using YOLOv7 with Test-Time Augmentation. arXiv preprint arXiv:2401.01018.
Cite this article
Luo,Y. (2025). The Evolution of YOLO: from YOLOv1 to YOLOv11 with a Focus on YOLOv7's Innovations in Object Detection. Theoretical and Natural Science,87,82-90.
Data availability
The datasets used and/or analyzed during the current study will be available from the authors upon reasonable request.
Disclaimer/Publisher's Note
The statements, opinions and data contained in all publications are solely those of the individual author(s) and contributor(s) and not of EWA Publishing and/or the editor(s). EWA Publishing and/or the editor(s) disclaim responsibility for any injury to people or property resulting from any ideas, methods, instructions or products referred to in the content.
About volume
Volume title: Proceedings of the 4th International Conference on Computing Innovation and Applied Physics
© 2024 by the author(s). Licensee EWA Publishing, Oxford, UK. This article is an open access article distributed under the terms and
conditions of the Creative Commons Attribution (CC BY) license. Authors who
publish this series agree to the following terms:
1. Authors retain copyright and grant the series right of first publication with the work simultaneously licensed under a Creative Commons
Attribution License that allows others to share the work with an acknowledgment of the work's authorship and initial publication in this
series.
2. Authors are able to enter into separate, additional contractual arrangements for the non-exclusive distribution of the series's published
version of the work (e.g., post it to an institutional repository or publish it in a book), with an acknowledgment of its initial
publication in this series.
3. Authors are permitted and encouraged to post their work online (e.g., in institutional repositories or on their website) prior to and
during the submission process, as it can lead to productive exchanges, as well as earlier and greater citation of published work (See
Open access policy for details).