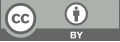
Research on wind speed prediction for Formula 1 grand prix based on multi-scale decomposition and time series neural network model
- 1 Beijing Institute of graphic communication
- 2 Beijing Institute of graphic communication
* Author to whom correspondence should be addressed.
Abstract
Wind speed variations have a significant impact on the aerodynamic characteristics of F1 racing cars and the race outcomes. To enhance the accuracy and stability of wind speed forecasting, this paper proposes a method based on multi-scale decomposition and time series neural network models. Initially, wind speed data collected from F1 Grand Prix events between 2018 and 2023 are subjected to Empirical Mode Decomposition (EMD), which decomposes the complex nonlinear and non-stationary time series data into several Intrinsic Mode Functions (IMFs) with different time scales. Subsequently, a Long Short-Term Memory network (LSTM) is utilized to predict each IMF component, and the predicted results of all IMFs are reconstructed into the overall wind speed forecast. Experimental results demonstrate that the method based on multi-scale decomposition and time series neural network models significantly outperforms traditional models such as Random Forest, Gradient Boosting Decision Tree (GBDT), and single LSTM models in terms of forecasting accuracy and stability. This study offers a novel perspective and approach for wind speed prediction in F1 Grand Prix events, with important theoretical significance and practical application value.
Keywords
Multi-scale Decomposition, Time Series Neural Network, Wind Speed Prediction
[1]. Preosti, E. Data Analysis for Formula 1. Berkeley Scientific Journal 2021, 25, 1-12.
[2]. Al Muharrami, M.S.; Almousa, S.M. Study of Open and Closed Wheels Aerodynamic of Racing Cars in Wind Tunnels with Movable Ground at Different Car Speeds. In Proceedings f the 18th Australasian Fluid Mechanics Conference, Launceston, Australia, 3-7 December 012.
[3]. Abdulwahab, S.F.; Chen, Y. Aerodynamic Evaluation of Racing Wings of a Formula Car. ournal of Applied Mechanics and Materials 2015, 776, 352-356.
[4]. Tan, J.J.; Myler, P.; Tan, W.A. Investigation and Analysis on Racing Car Front Wings. DEStech Transactions on Engineering and Technology Research 2017, 45, 132-138.
[5]. H.; Tian, H.Q.; Chen, C.; Li, Y. A Hybrid Statistical Method to Predict Wind Speed and Wind Power. Renewable Energy 2010, 35, 1857-1861.
[6]. Ye, X.W.; Ding, Y.; Wan, H.P. Machine Learning Approaches for Wind Speed Forecasting Using Long-Term Monitoring Data: A Comparative Study. Smart Structures and Systems 2019, 23, 375-386.
[7]. Younis, A.; Belabbes, F.; Cotfas, P.A.; Cotfas, D.T. Utilizing the Honeybees Mating-Inspired Firefly Algorithm to Extract Parameters of the Wind Speed Weibull Model. Forecasting 2024, 6, 20-30.
[8]. Rangaraj, A.G.; Srinath, Y.; Boopathi, K. Statistical Post-Processing of Numerical Weather Prediction Data Using Distribution-Based Scaling for Wind Energy. Wind Engineering 2024, 48, 145-160.
[9]. Kim, D.; Seomun, G.; Lee, Y.; Cho, H.; Chin, K.; Kim, M.H. Forecasting Building Energy Demand and On-Site Power Generation for Residential Buildings Using Long and Short-Term Memory Method with Transfer Learning. Applied Energy 2024, 343, 121025.
[10]. Cho, D.; Lee, S.; Im, J. Urban Summer Air Temperature Forecasting Through a Fusion of a Numerical Weather. In Handbook of Geospatial Approaches to Environmental Modeling; Springer: Berlin/Heidelberg, Germany, 2024; pp. 245-259.
[11]. Joseph, L.P.; Deo, R.C.; Casillas-Perez, D.; Prasad, R. Multi-Step-Ahead Wind Speed Forecast System: Hybrid Multivariate Decomposition and Feature Selection-Based Gated Additive Tree Ensemble Model. IEEE Transactions on Sustainable Energy 2024, 15, 105-120.
[12]. Ghose, B.; Pandit, P.; Mazumder, C.; Sinha, K. Comparative Study of EMD based Modelling Techniques for Improved Agricultural Price Forecasting. Journal of the Indian Society of Agricultural Statistics 2023, 77, 151432.
Cite this article
Chen,H.;Zhao,E. (2025). Research on wind speed prediction for Formula 1 grand prix based on multi-scale decomposition and time series neural network model. Theoretical and Natural Science,95,8-17.
Data availability
The datasets used and/or analyzed during the current study will be available from the authors upon reasonable request.
Disclaimer/Publisher's Note
The statements, opinions and data contained in all publications are solely those of the individual author(s) and contributor(s) and not of EWA Publishing and/or the editor(s). EWA Publishing and/or the editor(s) disclaim responsibility for any injury to people or property resulting from any ideas, methods, instructions or products referred to in the content.
About volume
Volume title: Proceedings of the 2nd International Conference on Applied Physics and Mathematical Modeling
© 2024 by the author(s). Licensee EWA Publishing, Oxford, UK. This article is an open access article distributed under the terms and
conditions of the Creative Commons Attribution (CC BY) license. Authors who
publish this series agree to the following terms:
1. Authors retain copyright and grant the series right of first publication with the work simultaneously licensed under a Creative Commons
Attribution License that allows others to share the work with an acknowledgment of the work's authorship and initial publication in this
series.
2. Authors are able to enter into separate, additional contractual arrangements for the non-exclusive distribution of the series's published
version of the work (e.g., post it to an institutional repository or publish it in a book), with an acknowledgment of its initial
publication in this series.
3. Authors are permitted and encouraged to post their work online (e.g., in institutional repositories or on their website) prior to and
during the submission process, as it can lead to productive exchanges, as well as earlier and greater citation of published work (See
Open access policy for details).