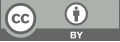
Sequential dual generative adversarial network for snowflake noise elimination
- 1 School of Computer Science, Zhongyuan University of Technology, Zhengzhou, China
- 2 School of Automation, Hangzhou Dianzi University, Hangzhou, China
- 3 School of Computer Science, Zhongyuan University of Technology, Zhengzhou, China
- 4 Zhongyuan University of Technology
* Author to whom correspondence should be addressed.
Abstract
Aiming at the problem of snowflake occlusion and haze veil effect in the collected images by the vehicle-mounted camera for the influence of snowflakes and haze. The decoupling and double-supervised weather elimination network was proposed based on the sequential reconstruction of high-frequency and low-frequency components in background images. Decoupling the desnow and dehaze assignment to two sub-networks sequentially, the network filters the high-frequency feature vectors in the image background region by the eigenvalues of the convolution kernel in the spatial domain, and then the second subnetwork performs image coloring and edge fine-tuning tasks based on edge context features obtained by generative adversarial networks. The algorithm has tested on the SRRS-6000 dataset, which verified the effectiveness in the significant performance on noise removal. The Peak Signal to Noise Ratio (PSNR) and Structural Similarity (SSIM) has reached 33.29dB/0.94, 32.8dB/0.9316, 30.13dB/0.93, 25.88dB/0.82 on Snow100K-S、Snow100K-M、Snow100K-L、I&O-Haze dataset, respectively. Experiments have shown that the decoupling and double supervised method have efficient snowflake and haze removal performance in image denoising tasks, and the adaptability of unmanned assistance systems under complex weather conditions has been enhanced.
Keywords
Snow Removal, Generative, Generative adversarial Network, Sequential Network
[1]. Li, H., Li, J., Zhao, D., and Xu, L., ‘Dehazeflow: Multi-Scale Conditional Flow Network for Single Image Dehazing‘, in, Proceedings of the 29th ACM International Conference on Multimedia, (2021)
[2]. Bharath Raj, N., ‘Single Image Haze Removal Using a Generative Adversarial Network‘, arXiv e-prints, 2018, p. arXiv: 1810.09479.
[3]. Chen, W.-T., Fang, H.-Y., Ding, J.-J., Tsai, C.-C., and Kuo, S.-Y., ‘Jstasr: Joint Size and Transparency-Aware Snow Removal Algorithm Based on Modified Partial Convolution and Veiling Effect Removal‘, in, European Conference on Computer Vision, (Springer, 2020)
[4]. Liu, Y.-F., Jaw, D.-W., Huang, S.-C., and Hwang, J.-N., ‘Desnownet: Context-Aware Deep Network for Snow Removal‘, IEEE Transactions on Image Processing, 2018, 27, (6), pp. 3064-3073.
[5]. Ancuti, C., Ancuti, C.O., Timofte, R., and Vleeschouwer, C.D., ‘I-Haze: A Dehazing Benchmark with Real Hazy and Haze-Free Indoor Images‘, in, International Conference on Advanced Concepts for Intelligent Vision Systems, (Springer, 2018)
[6]. Ancuti, C.O., Ancuti, C., Timofte, R., and De Vleeschouwer, C., ‘O-Haze: A Dehazing Benchmark with Real Hazy and Haze-Free Outdoor Images‘, in, Proceedings of the IEEE conference on computer vision and pattern recognition workshops, (2018)
[7]. Hore, A. and Ziou, D., ‘Image Quality Metrics: Psnr Vs. Ssim‘, in, 2010 20th international conference on pattern recognition, (IEEE, 2010)
[8]. Fu, X., Huang, J., Ding, X., Liao, Y., and Paisley, J., ‘Clearing the Skies: A Deep Network Architecture for Single-Image Rain Removal‘, IEEE Transactions on Image Processing, 2017, 26, (6), pp. 2944-2956.
[9]. Cai, B., Xu, X., Jia, K., Qing, C., and Tao, D., ‘Dehazenet: An End-to-End System for Single Image Haze Removal‘, IEEE Transactions on Image Processing, 2016, 25, (11), pp. 5187-5198.
[10]. Liu, X., Suganuma, M., Sun, Z., and Okatani, T., ‘Dual Residual Networks Leveraging the Potential of Paired Operations for Image Restoration‘, in, Proceedings of the IEEE/CVF Conference on Computer Vision and Pattern Recognition, (2019)
[11]. Dong, H., Pan, J., Xiang, L., Hu, Z., Zhang, X., Wang, F., and Yang, M.-H., ‘Multi-Scale Boosted Dehazing Network with Dense Feature Fusion‘, in, Proceedings of the IEEE/CVF conference on computer vision and pattern recognition, (2020)
[12]. Hong, M., Xie, Y., Li, C., and Qu, Y., ‘Distilling Image Dehazing with Heterogeneous Task Imitation‘, in, Proceedings of the IEEE/CVF Conference on Computer Vision and Pattern Recognition, (2020)
[13]. Qi, G.-J., ‘Loss-Sensitive Generative Adversarial Networks on Lipschitz Densities‘, International Journal of Computer Vision, 2020, 128, (5), pp. 1118-1140.
Cite this article
Li,Y.;Bao,X.;Qiang,Z.;Yang,G. (2025). Sequential dual generative adversarial network for snowflake noise elimination. Theoretical and Natural Science,95,18-30.
Data availability
The datasets used and/or analyzed during the current study will be available from the authors upon reasonable request.
Disclaimer/Publisher's Note
The statements, opinions and data contained in all publications are solely those of the individual author(s) and contributor(s) and not of EWA Publishing and/or the editor(s). EWA Publishing and/or the editor(s) disclaim responsibility for any injury to people or property resulting from any ideas, methods, instructions or products referred to in the content.
About volume
Volume title: Proceedings of the 2nd International Conference on Applied Physics and Mathematical Modeling
© 2024 by the author(s). Licensee EWA Publishing, Oxford, UK. This article is an open access article distributed under the terms and
conditions of the Creative Commons Attribution (CC BY) license. Authors who
publish this series agree to the following terms:
1. Authors retain copyright and grant the series right of first publication with the work simultaneously licensed under a Creative Commons
Attribution License that allows others to share the work with an acknowledgment of the work's authorship and initial publication in this
series.
2. Authors are able to enter into separate, additional contractual arrangements for the non-exclusive distribution of the series's published
version of the work (e.g., post it to an institutional repository or publish it in a book), with an acknowledgment of its initial
publication in this series.
3. Authors are permitted and encouraged to post their work online (e.g., in institutional repositories or on their website) prior to and
during the submission process, as it can lead to productive exchanges, as well as earlier and greater citation of published work (See
Open access policy for details).