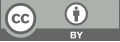
Power allocation based on reinforcement learning in 5G/B5G multi-cell networks
- 1 School of Aeronautics and Astronautics, University of Electronic Science and Technology of China, Chengdu, P.R.China
* Author to whom correspondence should be addressed.
Abstract
The fifth generation of wireless communication technology (5G), has revolutionized the digital landscape with its ultra-fast speeds, massive connectivity, and reduced latency; Beyond 5G (B5G), represents the evolutionary steps towards sixth-generation networks which aims to build upon 5G’s capabilities by integrating advanced technologies like ultra-dense network, edge computing, and enhanced spectral efficiency. This paper investigates the application of two distinct exponential reward functions in reinforcement learning algorithms for power allocation in ultra-dense networked base stations. The primary objective is to maximize the overall network capacity and spectral efficiency. The performance of the proposed reinforcement learning algorithms is compared with the traditional water-filling algorithm, as well as against the other to highlight the differences in learning outcomes resulting from the choice of reward functions. The results show that the exponential function model with reciprocal exponent is superior to the previous two in spectral efficiency and convergence speed and provide valuable insights into the effectiveness of using reinforcement learning for complex resource allocation problems in modern communication networks.
Keywords
Reinforcement learning, ultra-dense networks, reward functions, power allocation
[1]. Yi Yang, Fenglei li, Xinzhe Zhang, Zhixin Liu, Dynamic power allocation in cellular network based on multi-agent double deep reinforcement learning, School of Electrical Engineering, China, Version of Record 11 September 2022.
[2]. F. Meng, P. Chen, L. Wu and J. Cheng, Power Allocation in Multi-User Cellular Networks: Deep Reinforcement Learning Approaches, in IEEE Transactions on Wireless Communications.
[3]. Y. Oh, A. Ullah and W. Choi, Multi-Objective Reinforcement Learning for Power Allocation in Massive MIMO Networks: A Solution to Spectral and Energy Trade-Offs, in IEEE Access.
[4]. K. Antevski et al., A Q-learning strategy for federation of 5G services, ICC 2020 - 2020 IEEE International Conference on Communications (ICC), Dublin, Ireland, 2020, pp. 1-6, doi: 10.1109/ICC40277.2020.9149082.
[5]. Y. Zhang, C. Kang, T. Ma, Y. Teng and D. Guo, Power Allocation in Multi-Cell Networks Using Deep Reinforcement Learning, 2018 IEEE 88th Vehicular Technology Conference (VTC-Fall), Chicago, IL, USA, 2018, pp. 1-6, doi: 10.1109/VTCFall.2018.8690757.
[6]. Sun, M., Hu, L., Wang, Y., Zhang, H., Wang, J. (2022). Deep Reinforcement Learning for Resource
[7]. Allocation in Multi-cell Cellular Networks. In: Hassanien, A.E., Xu, Y., Zhao, Z., Mohammed, S., Fan, Z. (eds) Business Intelligence and Information Technology. BIIT 2021.
[8]. H. V. Hoang Phuc and H. Hoang Kha, Massive MIMO Power Allocation Using Deep Reinforcement Learning, 2023 International Symposium on Electrical and Electronics Engineering (ISEE), Ho Chi Minh, Vietnam, 2023, pp. 97-102.
[9]. F. B. Mismar, B. L. Evans and A. Alkhateeb, Deep Reinforcement Learning for 5G Networks: Joint Beamforming, Power Control, and Interference Coordination, in IEEE Transactions on Communications.
[10]. F. H. Costa Neto, D. C. Ara´ujo, M. P. Mota, T. F. Maciel and A. L. F. de Almeida, Uplink Power Control Framework Based on Reinforcement Learning for 5G Networks, in IEEE Transactions on Vehicular Technology.
[11]. ZHOU Shuo, QIU Runhe, TANG Minjun, Power allocation algorithm for CR-NOMA system based on tabu search and Q-learning
[12]. HU Langtao, BI Songjiao, LIU Quanjin, WU Jianlan, YANG Rui. Multi-Cell NOMA Energy Efficiency Optimization Power Allocation Algorithm Based on Reinforcement Learning[J]. Journal of University of Electronic Science and Technology of China, 2022, 51(3): 384-391.
[13]. D. Ma, Y. Wang, S. Wu and W. Wang, Using Reinforcement Learning for 5G RAN Slicing Resource
[14]. Allocation in New Power Load Management System, 2023 9th International Conference on Computer and Communications (ICCC), Chengdu, China, 2023, pp. 1164-1169.
Cite this article
Ren,K. (2025). Power allocation based on reinforcement learning in 5G/B5G multi-cell networks. Theoretical and Natural Science,95,53-62.
Data availability
The datasets used and/or analyzed during the current study will be available from the authors upon reasonable request.
Disclaimer/Publisher's Note
The statements, opinions and data contained in all publications are solely those of the individual author(s) and contributor(s) and not of EWA Publishing and/or the editor(s). EWA Publishing and/or the editor(s) disclaim responsibility for any injury to people or property resulting from any ideas, methods, instructions or products referred to in the content.
About volume
Volume title: Proceedings of the 2nd International Conference on Applied Physics and Mathematical Modeling
© 2024 by the author(s). Licensee EWA Publishing, Oxford, UK. This article is an open access article distributed under the terms and
conditions of the Creative Commons Attribution (CC BY) license. Authors who
publish this series agree to the following terms:
1. Authors retain copyright and grant the series right of first publication with the work simultaneously licensed under a Creative Commons
Attribution License that allows others to share the work with an acknowledgment of the work's authorship and initial publication in this
series.
2. Authors are able to enter into separate, additional contractual arrangements for the non-exclusive distribution of the series's published
version of the work (e.g., post it to an institutional repository or publish it in a book), with an acknowledgment of its initial
publication in this series.
3. Authors are permitted and encouraged to post their work online (e.g., in institutional repositories or on their website) prior to and
during the submission process, as it can lead to productive exchanges, as well as earlier and greater citation of published work (See
Open access policy for details).