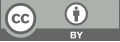
Artificial Intelligence in Gene Annotation: Current Applications, Challenges, and Future Prospects
- 1 School of Biological Science, Universiti Sains Malaysia, Penang, Malaysia
- 2 School of Biological Science, Universiti Sains Malaysia, Penang, Malaysia
* Author to whom correspondence should be addressed.
Abstract
Gene annotation is a critical process in genomics that involves the description of not only the position but also the function of an encoded element of a genome. In general, this provides biological context to sequence data, enabling an advanced level of understanding of genetic information. This is important in areas aligned with genetic engineering, studies of diseases, and evolution. Through ML and DL methodologies, AI enhances functional annotation and gene prediction effectively and accurately. This review focuses on AI in genomic research and assesses its effectiveness compared to traditional annotation tools. Using Escherichia coli as the representative model organism, the study focuses on a systematic approach of gene prediction using web Augustus with functional annotation using DeepGOPlus, an artificial intelligence tool, instead of the conventional BLAST-based annotation using the UniProt database. The study researches the extent of GO term coverage, the specificity of the annotations, and the concordance among these various tools. Artificial intelligence is highly beneficial owing to its speed, scalability, and proficiency in annotating intricate or poorly defined genomic areas. Notable instances include DeepGOPlus, which has demonstrated enhanced coverage by suggesting new terms that were frequently missed by earlier traditional tools. Notwithstanding these, AI tools face challenges such as dependence on high-quality training data, concerns about interpretability, and the need for biological validation to support the predictions. This review emphasizes the transformative impact that artificial intelligence brings to the field of gene annotation by presenting novel applications in many fields, including personalized medicine and synthetic biology, in which traditional methods suffer from severe limitations.
Keywords
artificial intelligence, machine learning, gene sequence, function prediction
[1]. Altschul SF, Gish W, Miller W, Myers EW, Lipman DJ. Basic local alignment search tool. J Mol Biol. 1990;215(3):403-10. Available from:https://pubmed.ncbi.nlm.nih.gov/2231712/
[2]. Libbrecht MW, Noble WS. Machine learning applications in genetics and genomics. Nat Rev Genet. 2015;16(6):321-32. Available from:https://doi.org/10.1038/nrg3920
[3]. Bairoch A, Apweiler R, Wu CH, Barker WC, Boeckmann B, Ferro S, et al. The Universal Protein Resource (UniProt). Nucleic Acids Res. 2004;32(Suppl_1):D115-9. Available from:https://pubmed.ncbi.nlm.nih.gov/15608167/
[4]. The UniProt Consortium. UniProt: a worldwide hub of protein knowledge. Nucleic Acids Res. 2019;47(D1):D506-15. Available from:https://pubmed.ncbi.nlm.nih.gov/30395287/
[5]. Kulmanov M, Khan MA, Hoehndorf R. DeepGO: predicting Gene Ontology terms with deep learning. Bioinformatics. 2018;34(4):660-7. Available from:https://academic.oup.com/bioinformatics/article/34/4/660/4265461?login=false
[6]. Zhou N, Jiang Y, Bergquist TR, Lee AJ, Zhi D, Lan M, et al. The CAFA challenge reports improved protein function prediction and new functional annotations for hundreds of genes through experimental screens. Genome Biol. 2019;20:244.Available from: https://link.springer.com/article/10.1186/s13059-019-1835-8
[7]. Elnaggar A, Heinzinger M, Dallago C, Rehawi G, Yu W, Jones L, et al. ProtTrans: towards cracking the language of life's code through self-supervised deep learning and high performance computing. Sci Rep. 2021;11(1):1-19. Available from:https://arxiv.org/abs/2007.06225
[8]. Samek W, Wiegand T, Müller KR. Explainable artificial intelligence: interpreting, explaining and visualizing deep learning models. IEEE Signal Process Mag. 2017;35(1):86-94. Available from https://link.springer.com/book/10.1007/978-3-030-28954-6
[9]. Senior AW, Evans R, Jumper J, Kirkpatrick J, Sifre L, Green T, et al. AlphaFold: a solution to a 50-year-old grand challenge in biology. Nature. 2020;577(7792):706-10. Available from: https://pubmed.ncbi.nlm.nih.gov/31942072/
[10]. Blattner FR, Plunkett G, Bloch CA, Perna NT, Burland V, Riley M, Collado-Vides J, Glasner JD, Rode CK, Mayhew GF, et al. 1997. The complete genome sequence of Escherichia coli K-12. Science. [accessed 2024 Dec 3];277(5331):1453-1474. https://pmc.ncbi.nlm.nih.gov/articles/PMC2907659/.
[11]. Bottcher S, Stephanopoulos N. 2023. Engineering multicomponent protein nanoparticles with programmable assembly and functionality. BioTechniques [accessed 2024 Dec 5];75(5):267-277. DOI: https://doi.org/10.2144/btn-2023-0023.
Cite this article
Xu,Y.;Cui,J. (2025). Artificial Intelligence in Gene Annotation: Current Applications, Challenges, and Future Prospects. Theoretical and Natural Science,98,8-15.
Data availability
The datasets used and/or analyzed during the current study will be available from the authors upon reasonable request.
Disclaimer/Publisher's Note
The statements, opinions and data contained in all publications are solely those of the individual author(s) and contributor(s) and not of EWA Publishing and/or the editor(s). EWA Publishing and/or the editor(s) disclaim responsibility for any injury to people or property resulting from any ideas, methods, instructions or products referred to in the content.
About volume
Volume title: Proceedings of the 3rd International Conference on Modern Medicine and Global Health
© 2024 by the author(s). Licensee EWA Publishing, Oxford, UK. This article is an open access article distributed under the terms and
conditions of the Creative Commons Attribution (CC BY) license. Authors who
publish this series agree to the following terms:
1. Authors retain copyright and grant the series right of first publication with the work simultaneously licensed under a Creative Commons
Attribution License that allows others to share the work with an acknowledgment of the work's authorship and initial publication in this
series.
2. Authors are able to enter into separate, additional contractual arrangements for the non-exclusive distribution of the series's published
version of the work (e.g., post it to an institutional repository or publish it in a book), with an acknowledgment of its initial
publication in this series.
3. Authors are permitted and encouraged to post their work online (e.g., in institutional repositories or on their website) prior to and
during the submission process, as it can lead to productive exchanges, as well as earlier and greater citation of published work (See
Open access policy for details).