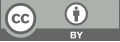
Optimal Inference of Social Network Structure: A Maximum Likelihood Framework for Erdős–Rényi Models
- 1 Nanjing Foreign Language School, Nanjing, China
* Author to whom correspondence should be addressed.
Abstract
In contemporary society, social networks have played a conspicuous role in people’s life, yet accurately analyzing them is still challenging. This paper applies maximum likelihood estimation for Erdős–Rényi graph in social network analysis. It begins with interpreting the significance of social network analysis and the objective of the research. Subsequently, theoretical knowledge of Erdős–Rényi graph and Maximum Likelihood Estimation are presented, including definitions, properties, and the derivation of Maximum Likelihood Estimation calculation formula for estimating the probability of connection in Erdős–Rényi graph. After that, a real-world example is involved in order to thoroughly comprehend the mechanism of this model. Through multiple trials of simulations, the accuracy of Maximum Likelihood Estimation is explored by illustrating a graph about the relationship between the number of nodes and percentage error with the help of python program, revealing the trend that a larger network size contributes to a more accurate estimation. The advantages, including simplicity and statistical rigor and disadvantages that contain the uniform assumption of connection probability and limitations are comprehensively analyzed as well, followed by a proposal of future research directions related to a more sophisticate model added by functions in order to be applied to friendship social networks, aiming to offer insights to further studies.
Keywords
Erdős–Rényi Graph, Maximum Likelihood Estimation, Social Network Analysis, Adjacency Matrix
[1]. Lambert, J., Barnstable, G., Minter, E., Cooper, J., & McEwan, D. (2022). Taking a one-week break from social media improves well-being, depression, and anxiety: A randomized controlled trial. Cyberpsychology, Behavior, and Social Networking, 25(6), 287–293.
[2]. Vanden Abeele, M. M. P., & Nguyen, M. H. (2024). Digital media as ambiguous goods: Examining the digital well-being experiences and disconnection practices of Belgian adults. European Journal of Communication, 39(1), 122–144.
[3]. Maganioti, A.E., Chrissanthi, H.D., Charalabos, P.C., Andreas, R.D., George, P.N. and Christos, C.N. (2010) Cointegration of Event-Related Potential (ERP) Signals in Experiments with Different Electromagnetic Field (EMF) Conditions. Health, 2, 400-406.
[4]. Zhu, X., Liu, Z., Cambria, E., Yu, X., Fan, X., Chen, H., & Wang, R. (2025). A client–server based recognition system: Non-contact single/multiple emotional and behavioral state assessment methods. Computer Methods and Programs in Biomedicine, 260, 108564.
[5]. Wang, R., Zhu, J., Wang, S., Wang, T., Huang, J., & Zhu, X. (2024). Multi-modal emotion recognition using tensor decomposition fusion and self-supervised multi-tasking. International Journal of Multimedia Information Retrieval, 13(4), 39.
[6]. Bootorabi, F., Haapasalo, J., Smith, E., Haapasalo, H. and Parkkila, S. (2011) Carbonic Anhydrase VII—A Potential Prognostic Marker in Gliomas. Health, 3, 6-12.
[7]. Koonin LM, Bialek S, et al. (2022). Building a New Generation of Public Health Leaders Forged in a Pandemic. JAMA Network Open, 5(5), e2213677.
[8]. Glendinning, I. (2013). Comparison of policies for academic integrity in higher education across the European Union.
[9]. Peng, P., Qu, L., Wang, Q., Wang, S., & Yan, T. (2025). Maximum likelihood estimation in the sparse Rasch model. arXiv preprint arXiv:2501.07770.De Ayala, R. J. (2019). The Rasch model in educational assessment: A primer. Measurement: Interdisciplinary Research and Perspectives, 17(2), 105-118.
[10]. Tarima, S., & Flournoy, N. (2019). Asymptotic properties of maximum likelihood estimators with sample size recalculation. Statistical Papers, 60(2), 373-394.
[11]. Wang, F., Ju, M., Zhu, X., Zhu, Q., Wang, H., Qian, C., & Wang, R. (2025). A Geometric algebra-enhanced network for skin lesion detection with diagnostic prior. The Journal of Supercomputing, 81(1), 1-24.
[12]. Zhao, Z., Zhu, X., Wei, X., Wang, X., & Zuo, J. (2021, June). Application of Workflow Technology in the Integrated Management Platform of Smart Park. In 2021 IEEE 4th Advanced Information Management, Communicates, Electronic and Automation Control Conference (IMCEC) (Vol. 4, pp. 1433-1437). IEEE.
[13]. Zhang, Y., Zhao, H., Zhu, X., Zhao, Z., & Zuo, J. (2019, October). Strain Measurement Quantization Technology based on DAS System. In 2019 IEEE 3rd Advanced Information Management, Communicates, Electronic and Automation Control Conference (IMCEC) (pp. 214-218). IEEE
Cite this article
Tao,C. (2025). Optimal Inference of Social Network Structure: A Maximum Likelihood Framework for Erdős–Rényi Models. Theoretical and Natural Science,100,165-171.
Data availability
The datasets used and/or analyzed during the current study will be available from the authors upon reasonable request.
Disclaimer/Publisher's Note
The statements, opinions and data contained in all publications are solely those of the individual author(s) and contributor(s) and not of EWA Publishing and/or the editor(s). EWA Publishing and/or the editor(s) disclaim responsibility for any injury to people or property resulting from any ideas, methods, instructions or products referred to in the content.
About volume
Volume title: Proceedings of the 3rd International Conference on Mathematical Physics and Computational Simulation
© 2024 by the author(s). Licensee EWA Publishing, Oxford, UK. This article is an open access article distributed under the terms and
conditions of the Creative Commons Attribution (CC BY) license. Authors who
publish this series agree to the following terms:
1. Authors retain copyright and grant the series right of first publication with the work simultaneously licensed under a Creative Commons
Attribution License that allows others to share the work with an acknowledgment of the work's authorship and initial publication in this
series.
2. Authors are able to enter into separate, additional contractual arrangements for the non-exclusive distribution of the series's published
version of the work (e.g., post it to an institutional repository or publish it in a book), with an acknowledgment of its initial
publication in this series.
3. Authors are permitted and encouraged to post their work online (e.g., in institutional repositories or on their website) prior to and
during the submission process, as it can lead to productive exchanges, as well as earlier and greater citation of published work (See
Open access policy for details).