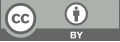
Evaluation of Adaptive Filtering Algorithms in Signal Denoising: Insights from Simulation and Algorithmic Enhancement
- 1 School of Changwang, Nanjing University of Information Science and Technology, Nanjing, Jiangsu Province, China
* Author to whom correspondence should be addressed.
Abstract
Signal denoising is an important research direction in the field of signal processing, with widespread applications in communication, audio processing, medical signal analysis, and other areas. With the development of technology, traditional noise reduction methods are gradually facing bottlenecks in efficiency and accuracy, especially in dynamically changing noise environments. Adaptive filtering algorithms have become effective tools for solving noise elimination problems due to their ability to adjust filter parameters in real time based on the characteristics of the input signal. However, classical adaptive algorithms, such as Least Mean Square (LMS) and Normalized Least Mean Square (NLMS) algorithms, despite their success in many applications, still face issues such as slow convergence and insufficient performance when handling different types of noise. This study aims to explore the application of adaptive filtering algorithms in signal denoising, particularly those based on second-order statistics, evaluate their performance in different noise environments, and optimize their enhancement. Initially, simulations were undertaken to implement the LMS, NLMS, and second-order statistics-based adaptive filtering algorithms for noise removal experiments. These experiments employed various noise power levels and signal types to assess the performance of each algorithm, focusing on metrics such as Signal-to-Noise Ratio (SNR), Mean Squared Error (MSE), and the convergence speed of filter parameters. The research results show that the adaptive filtering algorithm based on second-order statistics has significant advantages over LMS and NLMS algorithms in various noise environments, especially in cases of higher noise power, where its denoising effect is more significant, and the convergence speed is also faster. Additionally, to address the computational complexity of the algorithm, this study proposes a simplification strategy to optimize the practical application performance of the algorithm.
Keywords
Signal Denoising, Adaptive Filtering Algorithms, Second-order Statistics, Least Mean Square Algorithm, Simulation Evaluation
[1]. Guo, C., Wen, Y., Li, P., & Wen, J. (2016). Adaptive noise cancellation based on EMD in water-supply pipeline leak detection. Measurement, 79, 188-197.
[2]. Yadav, N. K., Dhawan, A., Tiwari, M., & Jha, S. K. (2024). Modified Model of RLS Adaptive Filter for Noise Cancellation. Circuits, Systems, and Signal Processing, 43(5), 3238-3260.
[3]. Ding, Y., Wang, Y., Yang, Y., Du, C., & Dong, W. (2021). An adaptive microwave photonic filter with LMS algorithm. Journal of Electromagnetic Waves and Applications, 36(8), 1076–1088.
[4]. Maurya, A. K. (2018). Cascade–cascade least mean square (LMS) adaptive noise cancellation. Circuits, Systems, and Signal Processing, 37(9), 3785-3826.
[5]. Teuber, T., Remmele, S., Hesser, J., & Steidl, G. (2012). Denoising by second order statistics. Signal Processing, 92(12), 2837-2847.
[6]. do Prado, R. A., Guedes, R. M., Henriques, F. D. R., da Costa, F. M., Tarrataca, L. D., & Haddad, D. B. (2020). On the Analysis of the Incremental l0-LMS Algorithm for Distributed Systems.
[7]. Yi, S., Jin, X., Su, T., Tang, Z., Wang, F., Xiang, N., & Kong, J. (2017). Online denoising based on the Second-Order Adaptive Statistics model. Sensors, 17(7), 1668.
Cite this article
Wang,W. (2025). Evaluation of Adaptive Filtering Algorithms in Signal Denoising: Insights from Simulation and Algorithmic Enhancement. Theoretical and Natural Science,101,60-68.
Data availability
The datasets used and/or analyzed during the current study will be available from the authors upon reasonable request.
Disclaimer/Publisher's Note
The statements, opinions and data contained in all publications are solely those of the individual author(s) and contributor(s) and not of EWA Publishing and/or the editor(s). EWA Publishing and/or the editor(s) disclaim responsibility for any injury to people or property resulting from any ideas, methods, instructions or products referred to in the content.
About volume
Volume title: Proceedings of CONF-MPCS 2025 Symposium: Mastering Optimization: Strategies for Maximum Efficiency
© 2024 by the author(s). Licensee EWA Publishing, Oxford, UK. This article is an open access article distributed under the terms and
conditions of the Creative Commons Attribution (CC BY) license. Authors who
publish this series agree to the following terms:
1. Authors retain copyright and grant the series right of first publication with the work simultaneously licensed under a Creative Commons
Attribution License that allows others to share the work with an acknowledgment of the work's authorship and initial publication in this
series.
2. Authors are able to enter into separate, additional contractual arrangements for the non-exclusive distribution of the series's published
version of the work (e.g., post it to an institutional repository or publish it in a book), with an acknowledgment of its initial
publication in this series.
3. Authors are permitted and encouraged to post their work online (e.g., in institutional repositories or on their website) prior to and
during the submission process, as it can lead to productive exchanges, as well as earlier and greater citation of published work (See
Open access policy for details).