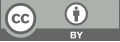
Multi-Frame Dual-Stream 2DCNN-LSTM Model for Automatic Modulation Recognition
- 1 Xi’an University, Xi'an, China
* Author to whom correspondence should be addressed.
Abstract
This paper proposes a multi-frame dual-stream 2DCNN-LSTM model (MF-DS-2DCNN-LSTM) for automatic modulation recognition. The model discretizes long sequences into two-dimensional frame structures and uses 2D CNN and LSTM together to model the spatiotemporal features of multi-channel IQ/AP signals. By employing a frame-based strategy, the original signal is reshaped into” small images,” with the 2D CNN extracting intra-frame spatial structures and inter-channel interaction features, while the LSTM captures the temporal evolution between frames. This approach integrates hierarchical modeling concepts from image processing and video analysis, and utilizes the Crested Porcupine Optimizer for hyperparameter tuning. Simulations show that, when recognizing nine modulation types, the model significantly outperforms methods such as CLDNN, achieving an average accuracy of 91.4% under high-SNR conditions (SNR above 2 dB). Moreover, the model maintains an accuracy of over 90% in small-sample training scenarios for SNRs above 4 dB. After optimization with the Crested Porcupine Optimizer, the model’s performance improved by 2.2%, and a 20.7% reduction in parameters was achieved.
Keywords
Automatic Modulation Recognition, CNN, LSTM, Crested Porcupine Optimizer
[1]. Ramezani-Kebrya, A., Kim, I. M., Kim, D. I., Chan, F., & Inkol, R. (2013). Likelihood-based modulation classification for multiple-antenna receiver. IEEE Transactions on Communications, 61(9), 3816-3829.
[2]. Abdi, A., Dobre, O. A., Choudhry, R., Bar-Ness, Y., & Su, W. (2004, October). Modulation classification in fading channels using antenna arrays. In IEEE MILCOM 2004. Military Communications Conference, 2004. (Vol. 1, pp. 211-217). IEEE.
[3]. El-Mahdy, A. E., & Namazi, N. M. (2002). Classification of multiple M-ary frequency-shift keying signals over a Rayleigh fading channel. IEEE Transactions on Communications, 50(6), 967-974.
[4]. Wei, W., & Mendel, J. M. (2000). Maximum-likelihood classification for digital amplitude-phase modulations. IEEE transactions on Communications, 48(2), 189-193.
[5]. Swami, A., & Sadler, B. M. (2000). Hierarchical digital modulation classification using cumulants. IEEE Transactions on communications, 48(3), 416-429.
[6]. Gardner, W. A., & Spooner, C. M. (1988, October). Cyclic spectral analysis for signal detection and modulation recognition. In MILCOM 88, 21st Century Military Communications-What's Possible?'. Conference record. Military Communications Conference (pp. 419-424). IEEE.
[7]. Marchand, P., Le Martret, C., & Lacoume, J. L. (1997, July). Classification of linear modulations by a combination of different orders cyclic cumulants. In Proceedings of the IEEE Signal Processing Workshop on Higher-Order Statistics (pp. 47-51). IEEE.
[8]. O’Shea, T. J., Corgan, J., & Clancy, T. C. (2016). Convolutional radio modulation recognition networks. In Engineering Applications of Neural Networks: 17th International Conference, EANN 2016, Aberdeen, UK, September 2-5, 2016, Proceedings 17 (pp. 213-226). Springer International Publishing.
[9]. Qi, P., Zhou, X., Zheng, S., & Li, Z. (2020). Automatic modulation classification based on deep residual networks with multimodal information. IEEE Transactions on Cognitive Communications and Networking, 7(1), 21-33.
[10]. Wu, Y., Li, X., & Fang, J. (2018, June). A deep learning approach for modulation recognition via exploiting temporal correlations. In 2018 IEEE 19th international workshop on signal processing advances in wireless communications (SPAWC) (pp. 1-5). IEEE.
[11]. Liu, F., Zhang, Z., & Zhou, R. (2021). Automatic modulation recognition based on CNN and GRU. Tsinghua Science and Technology, 27(2), 422-431.
[12]. Chen, C., Zhang, N., Yang, C., Gao, Y., & Sheng, Y. (2023, May). Multiple-Stream Model with CNN-LSTM for Automatic Modulation Recognition. In 2023 International Conference on Microwave and Millimeter Wave Technology (ICMMT) (pp. 1-3). IEEE.
[13]. Wang, Z., Wang, P., & Lan, P. (2022, December). Automatic modulation classification based on CNN, LSTM and attention mechanism. In 2022 IEEE 8th International Conference on Computer and Communications (ICCC) (pp. 105-110). IEEE.
[14]. Liang, R., Yang, L., Wu, S., Li, H., & Jiang, C. (2021, October). A three-stream CNN-LSTM network for automatic modulation classification. In 2021 13th International Conference on Wireless Communications and Signal Processing (WCSP) (pp. 1-5). IEEE.
[15]. Abdel-Basset, M., Mohamed, R., & Abouhawwash, M. (2024). Crested Porcupine Optimizer: A new nature-inspired metaheuristic. Knowledge-Based Systems, 284, 111257.
Cite this article
Zhou,Z. (2025). Multi-Frame Dual-Stream 2DCNN-LSTM Model for Automatic Modulation Recognition. Theoretical and Natural Science,101,107-116.
Data availability
The datasets used and/or analyzed during the current study will be available from the authors upon reasonable request.
Disclaimer/Publisher's Note
The statements, opinions and data contained in all publications are solely those of the individual author(s) and contributor(s) and not of EWA Publishing and/or the editor(s). EWA Publishing and/or the editor(s) disclaim responsibility for any injury to people or property resulting from any ideas, methods, instructions or products referred to in the content.
About volume
Volume title: Proceedings of CONF-MPCS 2025 Symposium: Mastering Optimization: Strategies for Maximum Efficiency
© 2024 by the author(s). Licensee EWA Publishing, Oxford, UK. This article is an open access article distributed under the terms and
conditions of the Creative Commons Attribution (CC BY) license. Authors who
publish this series agree to the following terms:
1. Authors retain copyright and grant the series right of first publication with the work simultaneously licensed under a Creative Commons
Attribution License that allows others to share the work with an acknowledgment of the work's authorship and initial publication in this
series.
2. Authors are able to enter into separate, additional contractual arrangements for the non-exclusive distribution of the series's published
version of the work (e.g., post it to an institutional repository or publish it in a book), with an acknowledgment of its initial
publication in this series.
3. Authors are permitted and encouraged to post their work online (e.g., in institutional repositories or on their website) prior to and
during the submission process, as it can lead to productive exchanges, as well as earlier and greater citation of published work (See
Open access policy for details).