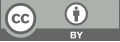
Integrated a Suitability Model to Locate the Potential Storage of Stormwater in New York City and Sacramento
- 1 Institute of Engineering, China Pharmaceutical University, Nanjing City, Jiangsu Province, 211198, China.
- 2 Shenyang Medical College, Liaoning province, Shenyang City, 110034, China.
- 3 Institute of Engineering, China Pharmaceutical University, Nanjing City, Jiangsu Province, 211198, China.
* Author to whom correspondence should be addressed.
Abstract
In this study, an XGBoost model optimized based on the Newton-Raphson algorithm is proposed for the task of kidney stone risk prediction, which improves the parameter updating strategy of the traditional gradient boosting framework by introducing second-order derivative information. In order to verify the effectiveness of the model, the performance differences between decision trees, random forests, standard XGBoost, CatBoost and the optimized model are systematically compared. The experimental results show that the XGBoost model optimized by the Newton-Raphson algorithm reaches 0.875 in both accuracy and recall indexes, which is significantly better than the other compared models, and its balanced assessment indexes both reflect the accurate identification ability of positive samples and verify the reliability of the overall prediction performance. Particularly noteworthy is that although Random Forest and standard XGBoost perform consistently in accuracy and recall, the differences in precision rate and AUC value reveal the essential difference between the two in feature space division and integration strategy: Random Forest reduces the risk of overfitting through feature randomness, while XGBoost relies on the regularization term to control the model complexity. The research results not only confirm the feasibility of the optimization algorithm in improving the performance of medical prediction models, but also provide an intelligent tool with practical application value for early screening and risk assessment of kidney stones in clinical practice with its stable prediction accuracy of 0.875.
Keywords
Kidney stones, Newton-Raphson algorithm, XGBoost
[1]. El-Mir, Abdulkader, et al. "Machine learning prediction of concrete compressive strength using rebound hammer test." Journal of Building Engineering 64 (2023): 105538.
[2]. Zeng, Ziyue, et al. "Accurate prediction of concrete compressive strength based on explainable features using deep learning." Construction and Building Materials 329 (2022): 127082.
[3]. Emad, Wael, et al. "Prediction of concrete materials compressive strength using surrogate models." Structures. Vol. 46. Elsevier, 2022.
[4]. Liu, Kexin, et al. "Development of compressive strength prediction platform for concrete materials based on machine learning techniques." Journal of Building Engineering 80 (2023): 107977.
[5]. Chi, Lin, et al. "Machine learning prediction of compressive strength of concrete with resistivity modification." Materials Today Communications 36 (2023): 106470.
[6]. Ghunimat, Dalin, et al. "Prediction of concrete compressive strength with GGBFS and fly ash using multilayer perceptron algorithm, random forest regression and k-nearest neighbor regression." Asian Journal of Civil Engineering 24.1 (2023): 169-177.
[7]. Liu, Gaoyang, and Bochao Sun. "Concrete compressive strength prediction using an explainable boosting machine model." Case Studies in Construction Materials 18 (2023): e01845.
[8]. Liu, Kexin, et al. "Development of compressive strength prediction platform for concrete materials based on machine learning techniques." Journal of Building Engineering 80 (2023): 107977.
[9]. Li, Hong, et al. "Compressive strength prediction of basalt fiber reinforced concrete via random forest algorithm." Materials Today Communications 30 (2022): 103117.
[10]. Imran, Hamza, et al. "Latest concrete materials dataset and ensemble prediction model for concrete compressive strength containing RCA and GGBFS materials." Construction and Building Materials 325 (2022): 126525.
Cite this article
Wu,H.;Qin,W.;Liu,X. (2025). Integrated a Suitability Model to Locate the Potential Storage of Stormwater in New York City and Sacramento . Theoretical and Natural Science,99,95-101.
Data availability
The datasets used and/or analyzed during the current study will be available from the authors upon reasonable request.
Disclaimer/Publisher's Note
The statements, opinions and data contained in all publications are solely those of the individual author(s) and contributor(s) and not of EWA Publishing and/or the editor(s). EWA Publishing and/or the editor(s) disclaim responsibility for any injury to people or property resulting from any ideas, methods, instructions or products referred to in the content.
About volume
Volume title: Proceedings of the 5th International Conference on Biological Engineering and Medical Science
© 2024 by the author(s). Licensee EWA Publishing, Oxford, UK. This article is an open access article distributed under the terms and
conditions of the Creative Commons Attribution (CC BY) license. Authors who
publish this series agree to the following terms:
1. Authors retain copyright and grant the series right of first publication with the work simultaneously licensed under a Creative Commons
Attribution License that allows others to share the work with an acknowledgment of the work's authorship and initial publication in this
series.
2. Authors are able to enter into separate, additional contractual arrangements for the non-exclusive distribution of the series's published
version of the work (e.g., post it to an institutional repository or publish it in a book), with an acknowledgment of its initial
publication in this series.
3. Authors are permitted and encouraged to post their work online (e.g., in institutional repositories or on their website) prior to and
during the submission process, as it can lead to productive exchanges, as well as earlier and greater citation of published work (See
Open access policy for details).