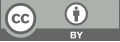
Machine learning drug discovery based on graph neural network and large language model
- 1 Cranbrook schools
* Author to whom correspondence should be addressed.
Abstract
The COVID-19 pandemic has presented an urgent need to understand the long-term health implications faced by survivors. Post-COVID-19 complications, such as acute kidney injury, arrhythmia, and stroke, pose significant challenges to public health. Despite extensive research on COVID-19 complications, a comprehensive understanding of the risk factors remains elusive due to the potential confounding variables present in the data. Traditional statistical models, while insightful, may not fully capture the causal relationships between these risk factors and post-COVID-19 complications. Motivated by this gap in the literature, we propose a novel approach using causal inference models to predict the likelihood of post-COVID-19 complications based on patient demographics and pre-existing conditions. Our model, trained on a dataset of COVID-19 inpatients in Wuhan Province, China, estimates the causal effect of these factors on the likelihood of patients experiencing post-COVID-19 complications. This approach allows us to isolate the causal impact of each factor while accounting for potential confounders, providing a more accurate understanding of the underlying mechanisms driving these relationships. Unlike traditional models that predict the probability of certain outcomes, our model provides insights into the causal relationships between risk factors and complications, offering a more reliable and comprehensive understanding of the underlying mechanisms. This approach can help identify at-risk patients, inform targeted interventions, and contribute to the development of effective prevention and treatment strategies. Our work aims to contribute to the current understanding of the virus and inform public health policies and interventions.
Keywords
Causal Inference, Post-COVID Complication, Risk Factors, Public Health
[1]. Long, B., Brady, W. J., Koyfman, A., & Gottlieb, M. (2020). Cardiovascular complications in COVID-19. The American journal of emergency medicine, 38(7), 1504-1507.
[2]. SeyedAlinaghi, S., Afsahi, A. M., MohsseniPour, M., Behnezhad, F., Salehi, M. A., Barzegary, A., ... & Dadras, O. (2021). Late complications of COVID-19; a systematic review of current evidence. Archives of academic emergency medicine, 9(1).
[3]. Qian, J. Y., Wang, B., Lv, L. L., & Liu, B. C. (2021). Pathogenesis of acute kidney injury in coronavirus disease 2019. Frontiers in physiology, 12, 586589.
[4]. Desai, A. D., Lavelle, M., Boursiquot, B. C., & Wan, E. Y. (2022). Long-term complications of COVID-19. American Journal of Physiology-Cell Physiology, 322(1), C1-C11.
[5]. Gabriel, J. T. (2020). Stroke as a complication and prognostic factor of COVID-19. Neurología (English Edition), 35(5), 318-322.
[6]. Rettner, R. (2021). 30% of people with COVID-19 experience symptoms up to 9 months later, https://www.livescience.com/long-covid-19-most-common-symptoms.html
[7]. Nalbandian, A., Sehgal, K., Gupta, A., Madhavan, M. V., McGroder, C., Stevens, J. S., ... & Wan, E. Y. (2021). Post-acute COVID-19 syndrome. Nature medicine, 27(4), 601-615.
[8]. Zhang, H., Wu, Y., He, Y., Liu, X., Liu, M., Tang, Y., ... & Wang, W. (2022). Age-related risk factors and complications of patients with COVID-19: a population-based retrospective study. Frontiers in medicine, 2643..
[9]. Abumayyaleh, M., Nunez-Gil, I. J., El-Battrawy, I., Estrada, V., Becerra-Muñoz, V. M., Uribarri, A., ... & Akin, I. (2021). Sepsis of patients infected by SARS-CoV-2: real-world experience from the international HOPE-COVID-19-registry and validation of HOPE sepsis score. Frontiers in medicine, 8, 728102.
[10]. Kuang, K., Cui, P., Athey, S., Xiong, R., & Li, B. (2018, July). Stable prediction across unknown environments. In proceedings of the 24th ACM SIGKDD international conference on knowledge discovery & data mining (pp. 1617-1626).
[11]. Beltramo, G., Cottenet, J., Mariet, A. S., Georges, M., Piroth, L., Tubert-Bitter, P., ... & Quantin, C. (2021). Chronic respiratory diseases are predictors of severe outcome in COVID-19 hospitalised patients: a nationwide study. European Respiratory Journal, 58(6).
[12]. Balepur, S. S., & Schlossberg, D. (2016). Hematologic Complications of Tuberculosis. Microbiology Spectrum, 4(6), 10-1128.
Cite this article
Huang,T. (2024). Machine learning drug discovery based on graph neural network and large language model. Theoretical and Natural Science,29,265-275.
Data availability
The datasets used and/or analyzed during the current study will be available from the authors upon reasonable request.
Disclaimer/Publisher's Note
The statements, opinions and data contained in all publications are solely those of the individual author(s) and contributor(s) and not of EWA Publishing and/or the editor(s). EWA Publishing and/or the editor(s) disclaim responsibility for any injury to people or property resulting from any ideas, methods, instructions or products referred to in the content.
About volume
Volume title: Proceedings of the 2nd International Conference on Modern Medicine and Global Health
© 2024 by the author(s). Licensee EWA Publishing, Oxford, UK. This article is an open access article distributed under the terms and
conditions of the Creative Commons Attribution (CC BY) license. Authors who
publish this series agree to the following terms:
1. Authors retain copyright and grant the series right of first publication with the work simultaneously licensed under a Creative Commons
Attribution License that allows others to share the work with an acknowledgment of the work's authorship and initial publication in this
series.
2. Authors are able to enter into separate, additional contractual arrangements for the non-exclusive distribution of the series's published
version of the work (e.g., post it to an institutional repository or publish it in a book), with an acknowledgment of its initial
publication in this series.
3. Authors are permitted and encouraged to post their work online (e.g., in institutional repositories or on their website) prior to and
during the submission process, as it can lead to productive exchanges, as well as earlier and greater citation of published work (See
Open access policy for details).