Volume 12
Published on September 2023Volume title: Proceedings of the 2023 International Conference on Mechatronics and Smart Systems
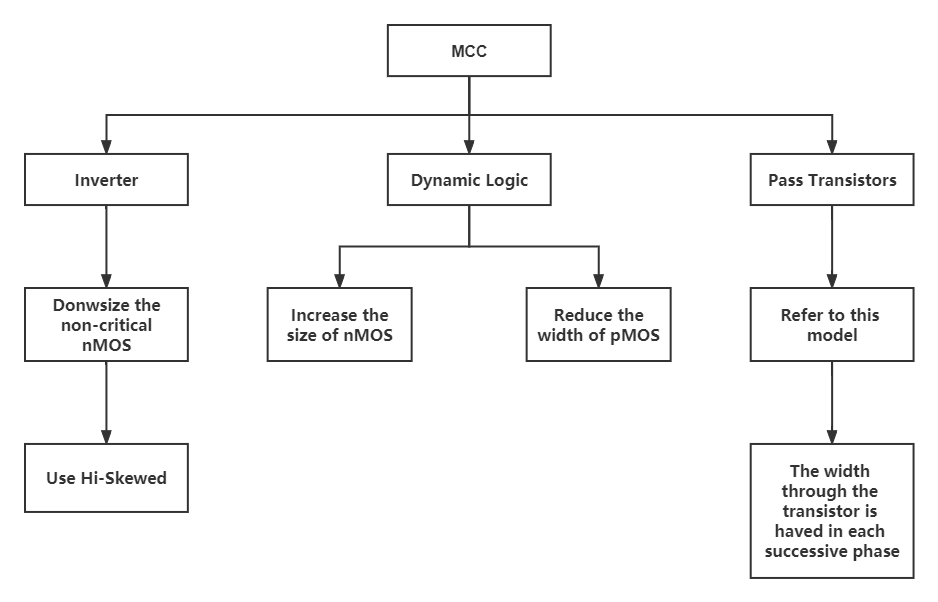
With the increasingly diverse functional requirements of contemporary electronic products, the complexity of CMOS circuits often used in chips becomes higher and the number of transistors used increases. To solve the resulting performance problems of CMOS circuits, researchers have searched for many transistor sizing technologies. This paper summarizes three methods of CMOS circuit optimization. The paper introduces these three methods in terms of principle, effect, and application scenarios, and compares them respectively. Through analysis and simulation, it can be found that the use of these methods in circuit design can effectively achieve the purpose of improving speed, reducing power consumption, and improving the overall performance of the circuit. This lays a solid foundation for finally being able to present a good product with excellent performance and enhance the market competitiveness of the product. CMOS circuits are widely used, and circuit optimization is of great importance to the overall circuit design, and better optimization methods can even promote the development of the entire electronics and chip manufacturing fields.
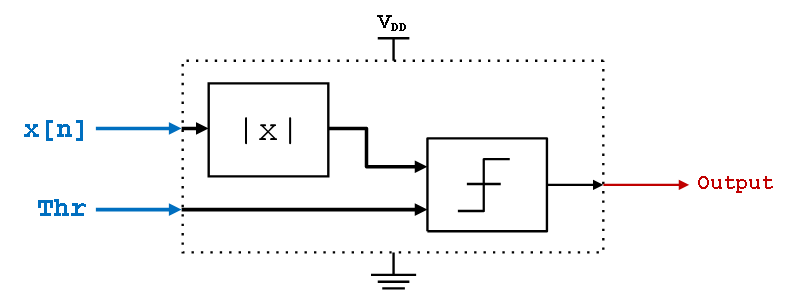
In recent years, as the rapid advancement of the electronic industry and the higher requirements for instruments and circuits, the absolute value circuit has found an increasingly wide utilization in digital signal processing. An absolute value detector is one of the most widely used algorithms used for detecting spikes. Based on traditional designs of absolute value circuits, many optimized designs with better performance have been proposed. Based on previous analysis and research, absolute value circuits can be optimized into different sructures to meet different application requirements. This paper introduces three different absolute value circuits for different uses in digital signal processing by comparing the optimized parts to traditional absolute value circuits and introducing how the optimization can meet the needs of different applications. These optimized absolute value circuit meet the requirements of higher precision and more stable temperature in biological signal processing field, more convenient to apply in the analog-to-digital conversion in ADC field and minimization the latency energy in chip signal processing field. Absolute value circuits can be optimized in different ways to be applied in more fields in the future.
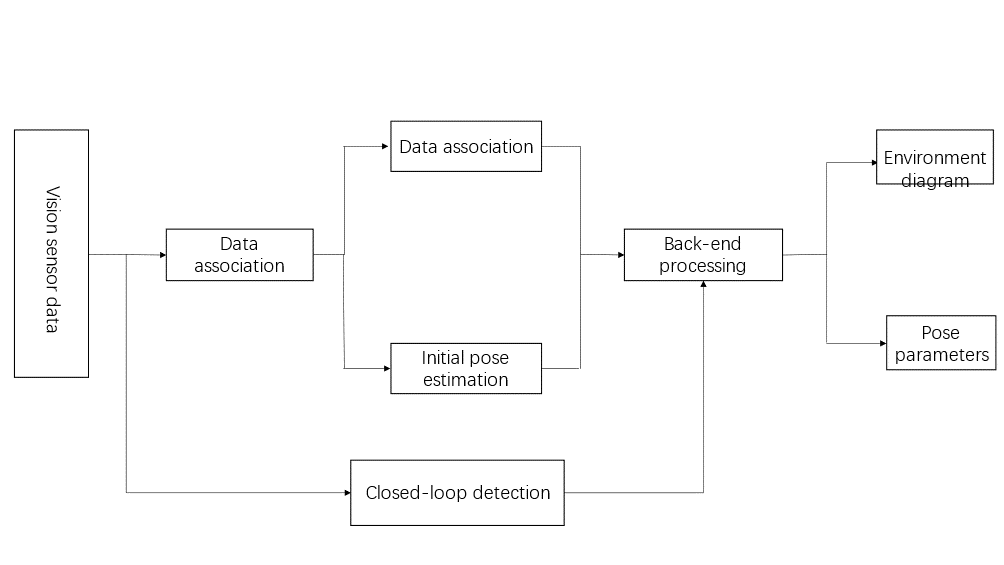
With the continuous development and improvement of sensors, sensor technology is also constantly making breakthroughs. It is also widely used in various fields of medicine today. This article summarizes the practice of two types of SLAM (Simultaneous Localization and Mapping) in medicine. The detection and matching of human luminal feature points under SLAM were studied so as to reconstruct the human internal environment so that doctors can obtain three-dimensional reconstruction feedback within the surgical range and improve the safety of surgery. And for different surgical environments, the same SLAM is improved on the basis of innovation, which is more suitable for different scenarios. Three algorithms are described in this article for taking images of the inside of the abdominal cavity and collecting data based on the images using different algorithms. Based on the collected data, the internal abdominal cavity is reconstructed in 3D. Finally, a three-dimensional visualization system for the abdominal cavity was constructed. It has important research significance in the field of medical auxiliary research.
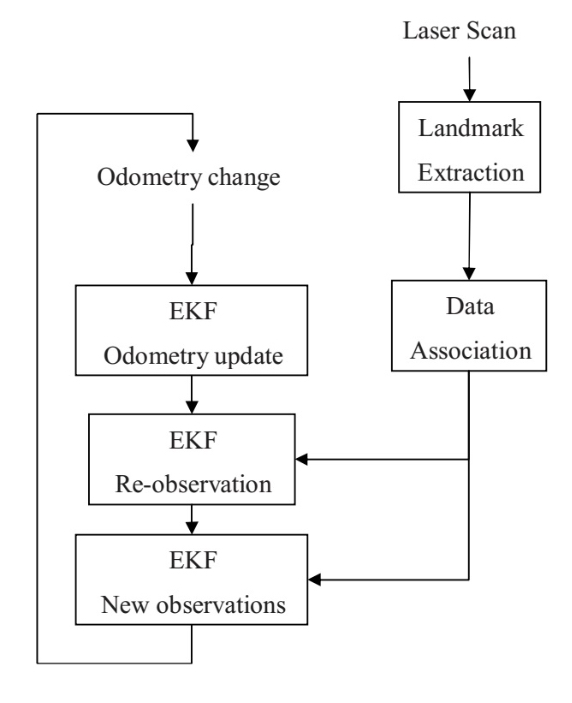
This paper presents the utilization of Simultaneous Localization and Mapping (SLAM) technology in medical endoscopic imaging. The fundamental components, hardware configuration, and process of SLAM are introduced in detail, with a focus on sensor acquisition, data preprocessing, feature extraction and matching, state estimation and update, map construction, and optimization modules. The application of SLAM in the medical field is then discussed, specifically highlighting real-time localization and reconstruction of endoscopic imaging. The integration of SLAM technology can assist doctors in accurately identifying the site of lesions, thereby enhancing surgical precision and safety. Furthermore, the paper introduces various commonly used SLAM algorithms, including the Kalman filter, extended Kalman filter, particle filter, optimization algorithms, among others. It emphasizes the significance of algorithm selection and optimization tailored to different scenarios and requirements. In conclusion, the application of SLAM technology in medical endoscopy imaging holds immense potential, offering improved accuracy and visualization during surgical procedures. This technology provides valuable support for doctors in diagnosis and treatment, ultimately enhancing patient outcomes.
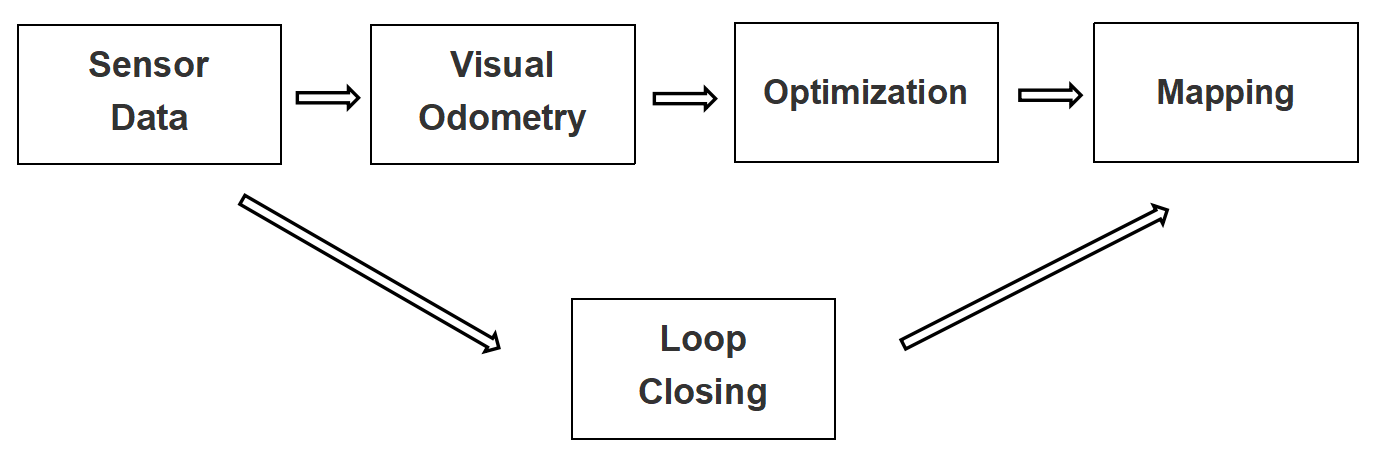
Simultaneous Localization and Mapping (SLAM) technology has many applications, such as intelligent robots, autonomous driving, and smart cities. It is a key technology for achieving autonomous robot navigation and environmental awareness. Robots need to perceive the surrounding environment in real-time and construct maps while conducting autonomous localization and path planning, which involves algorithms of SLAM technology. This article aims to introduce the research on visual SLAM algorithms in dynamic scenes. This article will first introduce the traditional SLAM algorithm, its research status, and its limitations. Next, introduce the research on the visual SLAM algorithm in dynamic scenes. Finally, the current visual SLAM algorithm's problems and future research directions were discussed. The research in this article will have significant value for the research and application of autonomous robot navigation, environmental perception, and smart cities.
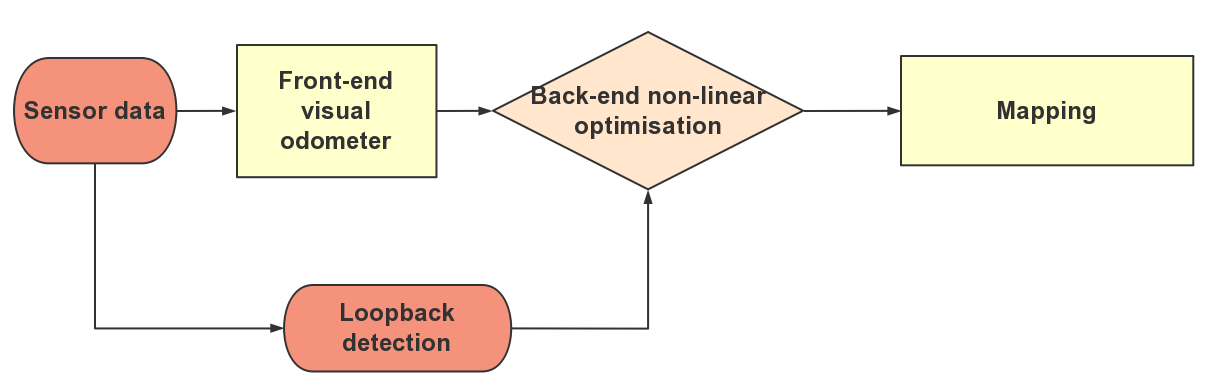
Due to developments in surgery, minimally invasive surgery (MIS) has become a common choice for surgical patients. Because of the small surgical incision in MIS, its postoperative effects will be less fat liquefaction, infection, splitting, numbness of the incision and weakness of the healing abdominal wall muscles compared to traditional surgery. However, many bottlenecks have been encountered in MIS, such as uneven illumination affecting the recognition of organ images. In other words, such problems are about improving the ability of Simultaneous Localization and Mapping (SLAM) to extract information about the environment. This paper summarises some relevant research on SLAM improvement methods. Scientists are now looking for more suitable methods for constructing maps of the human internal environment, including improvements on existing SLAM techniques. In the context of SLAM, what SLAM is and the history of SLAM is described. Traditional SLAM methods as well as SLAM improvement methods are also mentioned. Finally, its advantages and disadvantages are discussed, and its prospects are predicted.
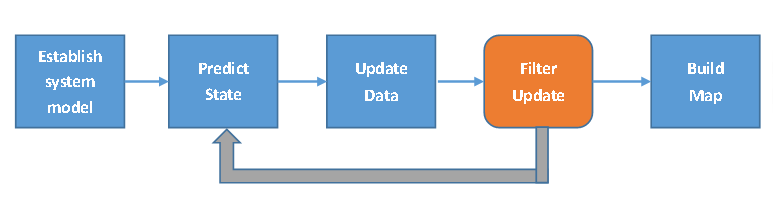
EKF (Extended Kalman Filter) is a non-linear state estimation algorithm based on the development of Kalman filtering technology. In SLAM (Simultaneous Localization and Mapping), EKF technology is widely used to realize robots' independent positioning and map construction. EKF technology mainly estimates the state of the robot by using the information provided by the sensor, and then realizes the robot's self-positioning and the environment's map. This paper uses EKF algorithm for testing, and analyses the simulation results, which compared with Kalman filtering technology, the main difference between the two is that EKF can handle non -linear dynamic systems. In SLAM, robots often detect environmental detection through sensors such as laser radar, camera, and then update the position and posture of the robot by the receiving sensor data. In this process, the EKF technology can perform non -linearity by sensor data by sensor data Transformation makes the state of the robot more accurately estimate. Specifically, Extended Kalman Filtering technology can be divided into two steps: prediction and update. In the predicted phase, EKF uses the current robotic status and motion equation to predict the robot status of the next moment; in the update stage, EKF uses sensors to measure data to update the current state of the robot. By repeating the prediction and updating two steps, you can get the trajectory of the robot movement and the map of the environment where the robot is located.
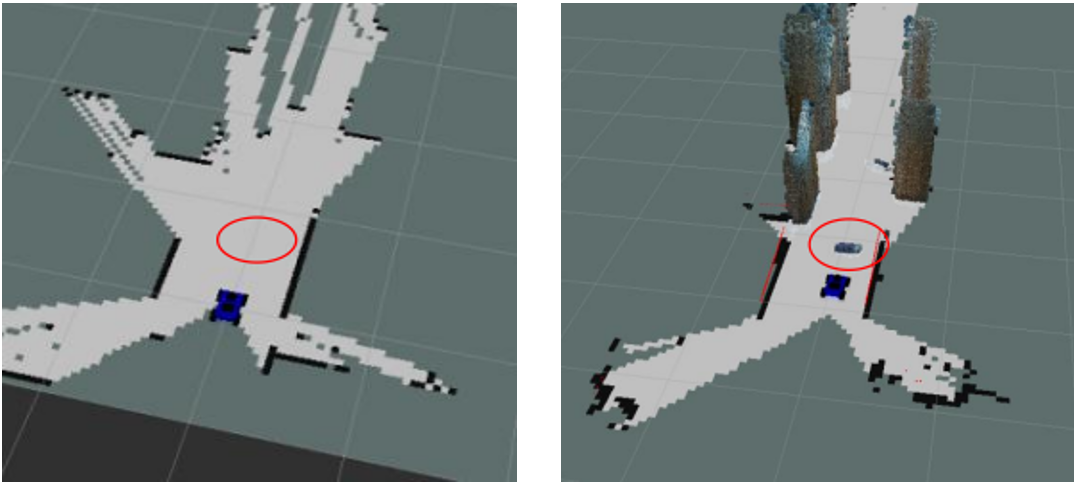
This paper reviews the application progress of Simultaneous localization and mapping (SLAM) in complex indoor environments. SLAM is a technology used to manufacture mobile robots and autonomous vehicle. It can achieve autonomous localization and mapping in unknown environments. In dealing with complex indoor environments, SLAM technology faces many challenges, such as missing local perspectives, detecting moving objects, constructing dense maps, and real-time requirements in large-scale environments. However, with the development of technology, more and more SLAM technologies are being applied to complex indoor environments, among which the fusion of multimodal vision and deep learning technology, enhanced camera positioning technology, and navigation algorithms based on intelligent platforms are currently relatively advanced technologies. This article will briefly introduce the main development process of SLAM technology in dealing with complex indoor environments and the application of deep learning technology. By utilizing this technology, map features can be extracted more accurately, objects can be recognized, and obstacle avoidance can be achieved. This provides a good development direction for SLAM technology.
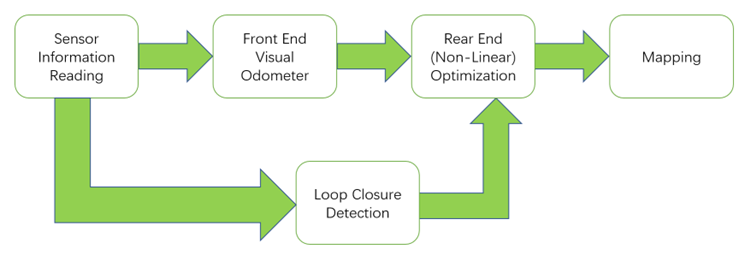
The Simultaneous Localization and Mapping (SLAM) method is widely used in the positioning and mapping of robots. In the medical field, SLAM is used in auxiliary medical robots and surgical robots. In endoscopic surgery, SLAM performs endoscopic positioning and scene graph construction for the surgical environment based on the information collected by the endoscope. For research on endoscopic SLAM, this article will first introduce the application of SLAM in endoscopic surgery in recent years. This paper summarizes the innovations and future work of relevant literature in recent years and identifies existing problems in SLAM in endoscopic surgery. Next, this article will introduce the combination of deep learning and SLAM in endoscopic surgery and list some specific applications. Finally, this paper will give a prospect for the future application of SLAM in endoscopic surgery. The research in this paper will be of great value to applying SLAM in endoscopic surgery and conducive to the development of future endoscopic SLAM.
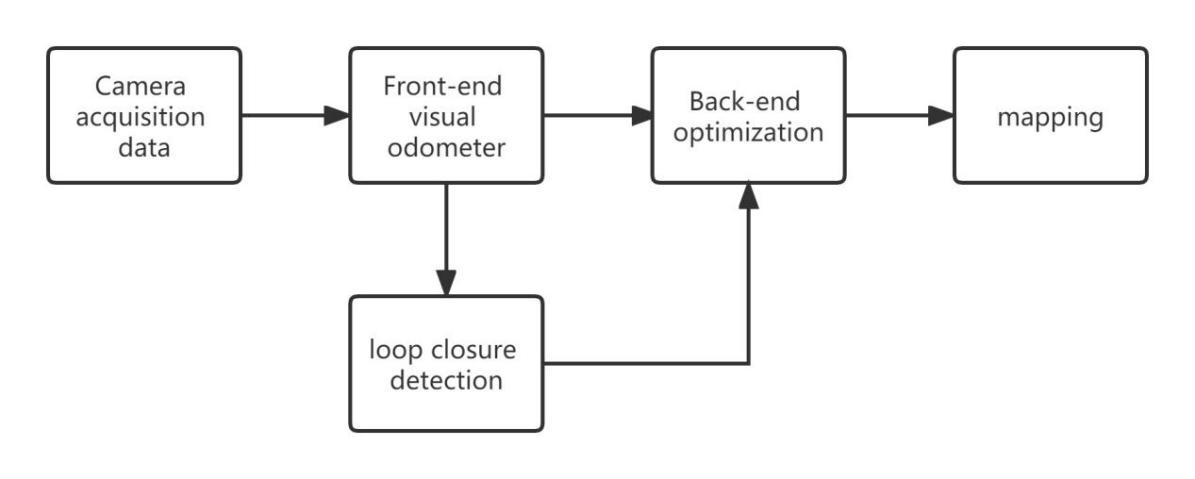
As a combination of modern imaging technology, stereotactic technology, computer technology, and artificial intelligence technology, the surgical navigation system has been rapidly developed and applied in recent years. In recent decades, navigation systems have been used to aid in maxillofacial surgery, but rarely in the oral area. By comparing the applications of navigation systems in oral and maxillofacial surgery, we summarize their advantages and problems and list the advantages and disadvantages of the development process and methods of Simultaneous Localization and Mapping(SLAM). We propose to use tooth contours as natural markers to construct a model of AR oral structure, subtracting the operational time problems caused by making and using artificial markers. The AR structure model of the oral cavity was constructed, the method of applying SLAM technology to the oral surgery was proposed reasonably, and the method optimization scheme was proposed for the more complicated oral root canal surgery.