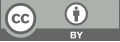
Research on the influencing factors of housing price based on multiple linear regression and random forest
- 1 Stony Brook College, Anhui University, Anhui, 230000, China
- 2 Changchun Second Experimental High School, Jilin, 130000, China
* Author to whom correspondence should be addressed.
Abstract
This paper aims to use multiple linear regression model and random forest models to analyze and study the factors affecting the housing price in Boston. The multiple linear regression model describes the relationship between multiple independent variables and one dependent variable through linear equations, and the random forest improves the accuracy and robustness by constructing multiple decision trees and combining their prediction results. To deal with complex nonlinear relationships and high dimensional data. Housing price is an important index to reflect the level and condition of economic and social development of a region, so it is of theoretical value and practical significance to explore its influencing factors and ways and degrees. Multiple factors are selected to analyze the weight and importance of each influencing factor, so as to help the government and decision makers to formulate more accurate policies, promote the stable development of the market, and provide scientific decision-making support for real estate developers, investors and ordinary buyers. In this study, the random forest model based on decision tree was used to clean, select and reduce the acquired housing price data, and to find out the main factors affecting housing price from the perspective of information gain, so as to obtain a relatively complete mathematical model and provide a reference scheme for future research by scholars.
Keywords
House price, multiple linear regression, random forest
[1]. Sheng J and Pan D D 2016 Analysis of influencing factors of housing price based on enhanced regression tree: A case study of Boston area. Statistics and Applications, 5(3), 299-304.
[2]. Li Y Q 2018 Housing price prediction model based on Random forest. Communications World, 306-308.
[3]. Zhao R 2019 Analysis of correlation between house price data in Boston based on regression method. Statistics and Applications, 9(3), 335-344.
[4]. Zhang Q Q 2021 Housing Price Prediction Based on Multiple Linear Regression. SCIENTIFIC PROGRAMMING.
[5]. Yang Y M and Tan S K 2011 Study on the influence of real Estate Price Factors on Housing price and its fluctuation in one hour city circle of the Pearl River Delta. China Land Science, 25(6), 54-59.
[6]. Cao T Y and Chen M Q 2019 Study on random forest variable selection based on studentized range distribution. Statistics and Information Forum, 36(8) 15-22.
[7]. Li S D 2021 Housing price forecasting model based on multivariate linear regression. Science and Technology Innovation, 91-92.
[8]. Guo L, Guo W W 2019 Prediction of thermal coal high calorific value based on SVR and random forest model. Energy Engineering, 44(1), 35-42.
[9]. Wang J T, et al. 2019 Gini Index and decision tree method for mitigating random consistency. Science in China: Information Science, 54(1), 159-190.
[10]. Zheng Y F 2007 Research on the spatial difference of housing prices in different urban areas of Hangzhou. Economic Forum, 32-34.
Cite this article
Jiang,X.;Li,C. (2024). Research on the influencing factors of housing price based on multiple linear regression and random forest. Theoretical and Natural Science,52,49-57.
Data availability
The datasets used and/or analyzed during the current study will be available from the authors upon reasonable request.
Disclaimer/Publisher's Note
The statements, opinions and data contained in all publications are solely those of the individual author(s) and contributor(s) and not of EWA Publishing and/or the editor(s). EWA Publishing and/or the editor(s) disclaim responsibility for any injury to people or property resulting from any ideas, methods, instructions or products referred to in the content.
About volume
Volume title: Proceedings of CONF-MPCS 2024 Workshop: Quantum Machine Learning: Bridging Quantum Physics and Computational Simulations
© 2024 by the author(s). Licensee EWA Publishing, Oxford, UK. This article is an open access article distributed under the terms and
conditions of the Creative Commons Attribution (CC BY) license. Authors who
publish this series agree to the following terms:
1. Authors retain copyright and grant the series right of first publication with the work simultaneously licensed under a Creative Commons
Attribution License that allows others to share the work with an acknowledgment of the work's authorship and initial publication in this
series.
2. Authors are able to enter into separate, additional contractual arrangements for the non-exclusive distribution of the series's published
version of the work (e.g., post it to an institutional repository or publish it in a book), with an acknowledgment of its initial
publication in this series.
3. Authors are permitted and encouraged to post their work online (e.g., in institutional repositories or on their website) prior to and
during the submission process, as it can lead to productive exchanges, as well as earlier and greater citation of published work (See
Open access policy for details).