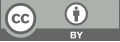
Exploring Spotify's Music Popularity Dynamics and Forecasting with Machine Learning
- 1 High School Affiliated to Renmin University of China
* Author to whom correspondence should be addressed.
Abstract
Spotify, one of the largest music streaming service providers, boasts a vast user base and an extensive music catalog. To gain a deeper understanding of Spotify users' music preferences and behavioral patterns, this paper conducts a thorough analysis and explores the correlation between music features and user behavior. It collects Spotify users' music listening data and extracts various music features such as energy, danceability, valence, and beats per minute (BPM). These features not only reflect the musical style and rhythm but also potentially correlate with users' preferences and listening habits. Then we employ two machine learning models, Decision Trees and Random Forests, to model and analyze Spotify users' behavior data. Through these models, we can accurately identify potential relationships between music features and user behavior. The experimental results indicate that music features such as energy, danceability, valence, and BPM have a significant impact on users' music selection and preferences. By analyzing the most popular songs on Spotify over different time periods, we discover that songs with high energy, danceability, and valence tend to be more popular among users. This finding not only validates the correlation between music features and user behavior but also reveals the user preferences and market demands behind music trends. For music streaming service providers, understanding users' music preferences and behavioral patterns is crucial. By deeply analyzing user data, music streaming services can better satisfy users' needs, enhance user experience, and stand out in the fiercely competitive market. These findings have important practical implications for music streaming service providers and provide new insights and methodologies for the research and development of the music industry.
Keywords
Music, Popular Trend, Decision Tree, Random Forest.
[1]. Interiano Myra, et al. Music Trends and Predictability of success in Contemporary Songs in and out of the Top Charts. Royal Society Open Science, 2018.
[2]. Lee Junghyuk, Lee Jong-Seok. Music Popularity: Metrics, Characteristics, and Audio-based Prediction. IEEE Transactions on Multimedia, 2018.
[3]. Li Kun, et al. Research for Music Trend Prediction Based on LSTM-RPA[C]. Computer Engineering and Applications, 2022, 58(24).
[4]. Liu Xiangli. Music Trend Prediction Based on Improved LSTM and Random Forest Algotithm. Journal of Sensors, 2022.
[5]. Cai Honghao, et al. Research on the Influence Factors and Genre Development Trends of Music Based on PageRank and LSTM Model. IEEE Xplore, 2021.
[6]. Yu Weisheng, et al. Music Popular Trends Prediction Based on Time Series. Computer Engineering & Science, 2018.
[7]. Wang Zhen-ye, et al. Music Trend Forecast Based on LSTM-Att Method. Computer Technology and Development, 2020.
[8]. Xu Yichen, et al. Prediction Model of Music Popular Trend Based on NNS and DM Technology. Mathematical Modeling for Next-Generation Big Data Technologies, 2022.
[9]. Xuyan Wang. Prediction Algorithm of Music Trend Based on Neural Network. The International Conference on Cyber Security Intelligence and Analytics, 2022.
[10]. Kim Yekyung, et al. Now Playing the Future Billboard: Mining Music Listening Behaviors of Twitter Users for Hit Song Prediction. Proceedings of the first international workshop on Social Media Retrieval and Analysis, 2014.
Cite this article
Shu,M. (2024). Exploring Spotify's Music Popularity Dynamics and Forecasting with Machine Learning. Theoretical and Natural Science,53,83-89.
Data availability
The datasets used and/or analyzed during the current study will be available from the authors upon reasonable request.
Disclaimer/Publisher's Note
The statements, opinions and data contained in all publications are solely those of the individual author(s) and contributor(s) and not of EWA Publishing and/or the editor(s). EWA Publishing and/or the editor(s) disclaim responsibility for any injury to people or property resulting from any ideas, methods, instructions or products referred to in the content.
About volume
Volume title: Proceedings of the 2nd International Conference on Applied Physics and Mathematical Modeling
© 2024 by the author(s). Licensee EWA Publishing, Oxford, UK. This article is an open access article distributed under the terms and
conditions of the Creative Commons Attribution (CC BY) license. Authors who
publish this series agree to the following terms:
1. Authors retain copyright and grant the series right of first publication with the work simultaneously licensed under a Creative Commons
Attribution License that allows others to share the work with an acknowledgment of the work's authorship and initial publication in this
series.
2. Authors are able to enter into separate, additional contractual arrangements for the non-exclusive distribution of the series's published
version of the work (e.g., post it to an institutional repository or publish it in a book), with an acknowledgment of its initial
publication in this series.
3. Authors are permitted and encouraged to post their work online (e.g., in institutional repositories or on their website) prior to and
during the submission process, as it can lead to productive exchanges, as well as earlier and greater citation of published work (See
Open access policy for details).