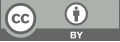
Predicting Housing Price Using Regression Analysis: A Study Based on California Housing Price
- 1 Shanghai International High School of BANZ, Shanghai, 200000, China
* Author to whom correspondence should be addressed.
Abstract
The purpose of the essay is to examine the factors influencing housing prices in California, which can help people to obtain a perspective to observe the US economy. This paper uses the California House Price dataset to analyze the factors influencing house prices of blocks of California by performing correlation analysis and multiple linear regression. From the analyses, it is discovered that median income has the strongest correlation with median house price of blocks in California. A model predicting California house prices is also shown. It is observed that median income has the most significant correlation with median housing price in California from the correlation analysis. Additionally, the linear regression model is obtained to estimate the housing prices, with a good fit of the R2 value at 0.637. Though the issue of multicollinearity occurs, this model still provides an insight into explaining housing prices in California. For a more advanced housing price predicting model, this variable and other factors of blocks in California may be included.
Keywords
Housing price, linear regression model, influencing factors
[1]. Tsatsaronis, K. and Zhu, H. (2004) What drives housing price dynamics: cross-country evidence. BIS Quarterly Review, March.
[2]. Bohn, S. and Duan, J., (2025) California’s Economy, Public Institute of California. International Journal of Information Engineering & Electronic Business.
[3]. Park, B. and Bae, J.K. (2015) Using machine learning algorithms for housing price prediction: The case of Fairfax County, Virginia housing data. Expert systems with applications, 42(6), 2928-2934.
[4]. Nguyen, N. and Cripps, A. (2001) Predicting housing value: A comparison of multiple regression analysis and artificial neural networks. Journal of real estate research, 22(3), 313-336.
[5]. Truong, Q., Nguyen, M., Dang, H. and Mei, B. (2020) Housing price prediction via improved machine learning techniques. Procedia Computer Science, 174, 433-442.
[6]. Fedotova, O., Teixeira, L. and Alvelos, H. (2013) Software Effort Estimation with Multiple Linear Regression: Review and Practical Application. J. Inf. Sci. Eng., 29(5), 925-945.
[7]. Zhang, Q. (2021) Housing price prediction based on multiple linear regression. Scientific Programming, 1, 7678931.
[8]. Thamarai, M. and Malarvizhi, S.P. (2020) House Price Prediction Modeling Using Machine Learning. International Journal of Information Engineering & Electronic Business, 12(2).
[9]. Coulson, N.E. and Daniel P.M. (2008) Estimating time, age and vintage effects in housing prices. Journal of Housing Economics, 17, 138-151.
[10]. Sani, M.M. and Rahim, A. (2015) Price to income ratio approach in housing affordability. Journal of Economics, Business and Management, 3, 1190-1193.
Cite this article
Gu,Y. (2025). Predicting Housing Price Using Regression Analysis: A Study Based on California Housing Price. Theoretical and Natural Science,105,128-134.
Data availability
The datasets used and/or analyzed during the current study will be available from the authors upon reasonable request.
Disclaimer/Publisher's Note
The statements, opinions and data contained in all publications are solely those of the individual author(s) and contributor(s) and not of EWA Publishing and/or the editor(s). EWA Publishing and/or the editor(s) disclaim responsibility for any injury to people or property resulting from any ideas, methods, instructions or products referred to in the content.
About volume
Volume title: Proceedings of the 3rd International Conference on Mathematical Physics and Computational Simulation
© 2024 by the author(s). Licensee EWA Publishing, Oxford, UK. This article is an open access article distributed under the terms and
conditions of the Creative Commons Attribution (CC BY) license. Authors who
publish this series agree to the following terms:
1. Authors retain copyright and grant the series right of first publication with the work simultaneously licensed under a Creative Commons
Attribution License that allows others to share the work with an acknowledgment of the work's authorship and initial publication in this
series.
2. Authors are able to enter into separate, additional contractual arrangements for the non-exclusive distribution of the series's published
version of the work (e.g., post it to an institutional repository or publish it in a book), with an acknowledgment of its initial
publication in this series.
3. Authors are permitted and encouraged to post their work online (e.g., in institutional repositories or on their website) prior to and
during the submission process, as it can lead to productive exchanges, as well as earlier and greater citation of published work (See
Open access policy for details).