Volume 7
Published on April 2024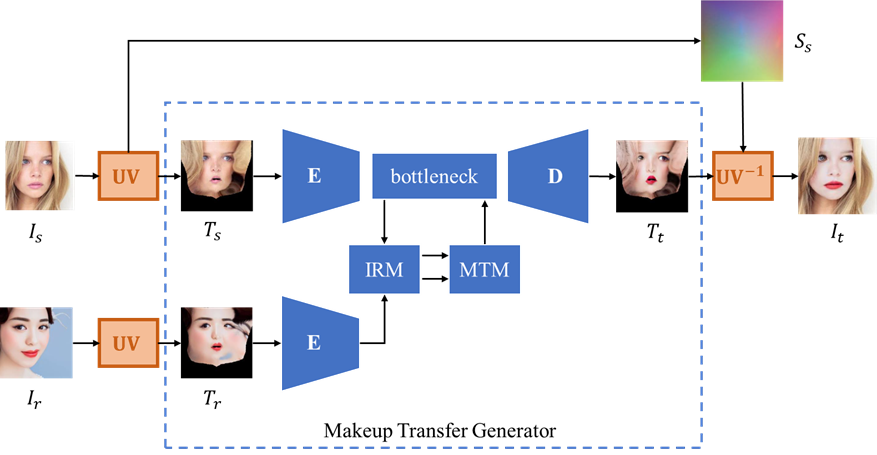
Recently, makeup transfer task has been widely explored with the development of deep learning. However, existing methods have shortcomings in more complex lighting situations in the real world because they do not consider the interference of lighting factors on facial features. To solve the above problem, we propose a local highlight and shadow adaptively repairing GAN for illumination-robust makeup transfer. We first map the 2D face images to UV representations and perform makeup transfer in the UV texture space, which explicitly removes the spatial misalignment to achieve pose and expression invariant makeup transfer. Furthermore, we take advantage of the face symmetry in the UV texture space to design an illumination repair module. It can adaptively repair the features affected by asymmetric local highlight and shadow based on a process of flipping and multi-layer attention fusion. In addition, the multi-layer attention maps are obtained by a pre-trained illumination classification network and hence have the ability to indicate local highlight and shadow areas. Comprehensive experiment results demonstrate the consistent effectiveness and clear advantages of our method, which significantly improve the robustness against local light effects and generate natural transfer results.
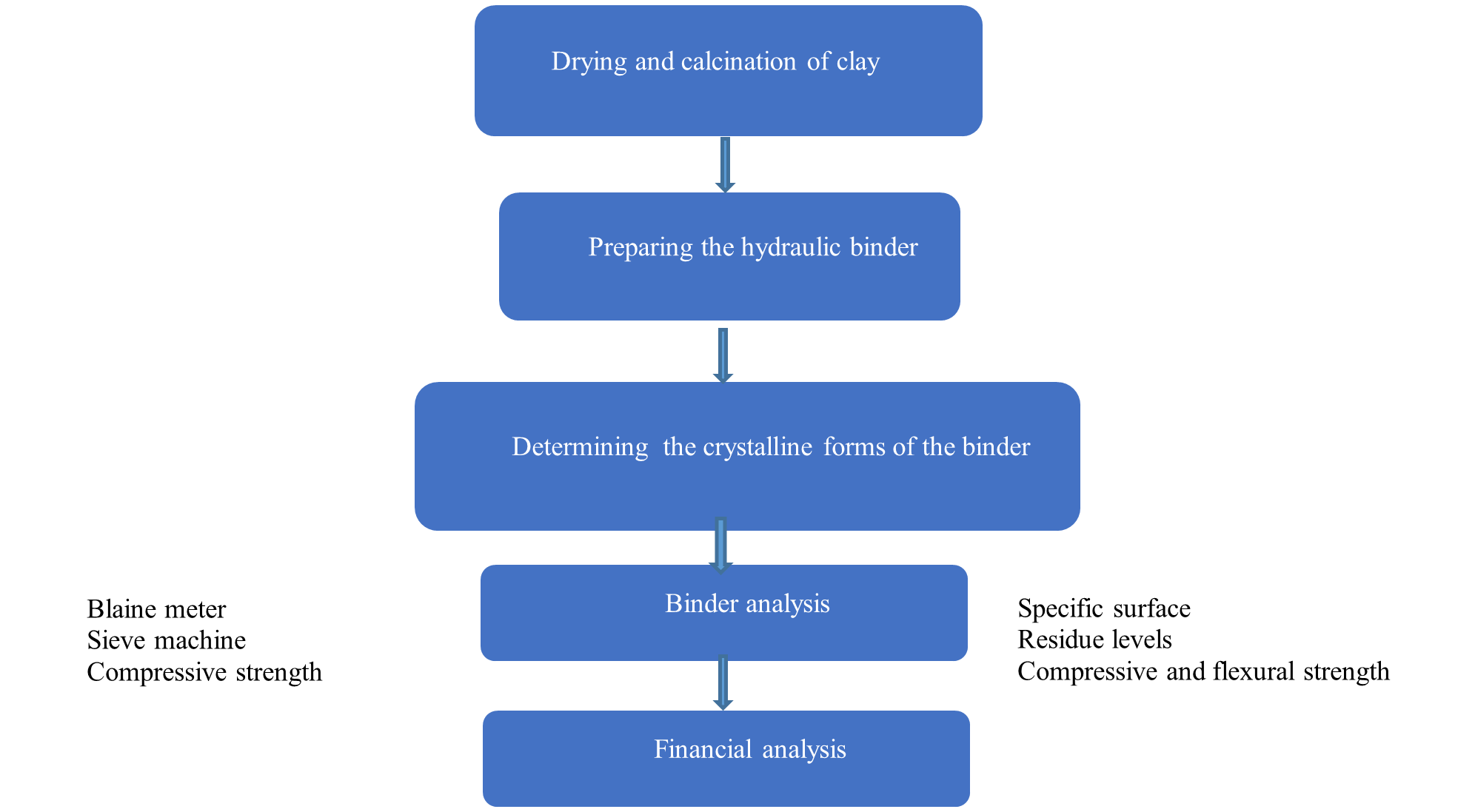
The formulation of a calcined clay-based hydraulic binder is an innovative solution for reducing the carbon footprint in the construction industry. This binder is made from calcined clay, a natural and abundant material that does not require high-temperature firing in the 400°C to 950°C range. In our study, we worked respectively under the following temperatures: 550°C, 650°C, 750°C and 850°C, unlike traditional binders whose clinker is fired at a temperature of around 1450°C. The blaine tests showed that the binder had been well ground, resulting in a much finer binder. We then obtained various blaines varying between 5000 and 8000 Cm2/g, unlike the blaine of the traditional binder, which is 3800 Cm2/g; the residue rate is very high compared with that of the traditional binder, due to the grinding carried out in a laboratory ball mill. The results of resistance tests at 30% gave 34.8, 48.8, 36.5 and 46 respectively; those at 40% gave 42.7, 45.4, 44.4 and 44.8, while the resistance of traditional binder gave 42.5. In other words, we obtained strengths comparable to those of the traditional binder. In addition, it was found that the manufacture of calcined clay-based hydraulic binders reduces CO2 emissions compared with traditional binders, which emit very high levels of CO2, making the production of calcined clay-based binders a more environmentally-friendly option for the cement industry. The financial assessment carried out at the end of this study shows that the production of a calcined clay-based binder is more economical than that of a traditional binder (Portland cement).
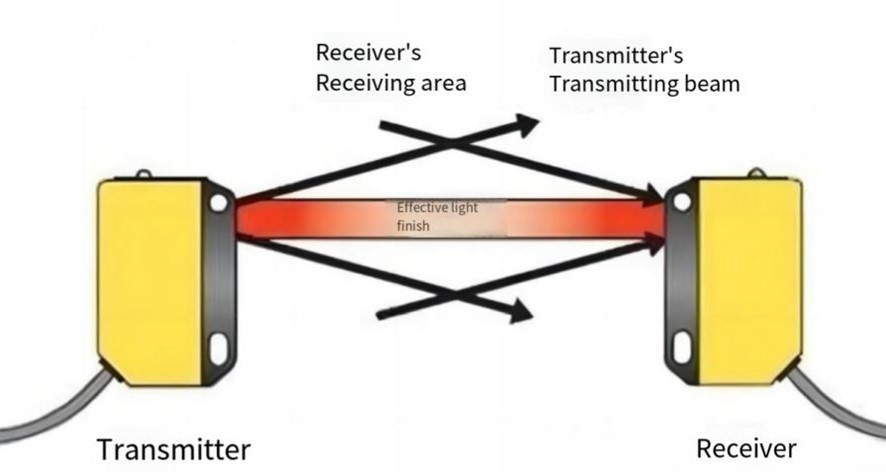
In order to meet the increasing demand for electronic products in contemporary society, relevant manufacturers have been continuously improving related technologies. While electronic products are convenient, they are plagued by issues such as rapid power consumption, susceptibility to damage, and inconvenience in portability. Therefore, many countries have developed foldable screen smartphones, computers, and other electronic products. In China, major brands such as OPPO and Huawei have released foldable screen smartphones, effectively addressing issues like portability. In this article, a background study of flexible tactile sensors is first conducted to ensure a thorough understanding of their applications. Secondly, based on the working principle of flexible tactile sensors and their different categories, the current research status is analyzed. The classification can be further refined according to the information transmission mechanism and application scenarios. In the former classification, detailed principal introductions are provided for each type of sensor. In the latter classification, based on the existing environment, detailed analyses of the production methods and characteristics are carried out in multiple fields such as tablets and smartphones, laying the groundwork for subsequent discussions. Furthermore, from the perspectives of preparation, characteristics, and power supply, I identify the existing problems and shortcomings that need improvement today, providing detailed explanations. Finally, relevant solutions are proposed for the aforementioned issues, and prospects for the extensive application and bright future of flexible tactile sensors are discussed, including in the fields of biointerfaces, cellular domains, and future mechanical domains.
With the ever-increasing volume of data being generated and shared across various platforms, the challenge of maintaining privacy while extracting value from this data has become paramount. This paper delves into the realm of Privacy-Preserving Data Analysis (PPDA), examining its current landscape and the pivotal techniques shaping it. Using datasets from diverse domains, we evaluated four leading PPDA techniques—Differential Privacy, Homomorphic Encryption, Secure Multi-Party Computation (SMPC), and Data Obfuscation—to discern their efficacy and trade-offs in terms of data utility and privacy breach risk. Our findings underscore the strengths and constraints of each method, guiding researchers and practitioners in choosing the optimal approach for specific scenarios. As data continues to be an invaluable asset in the digital age, the tools and techniques to analyze it privately will play a critical role in shaping future data-driven decision-making processes.
The evolution of software architecture has witnessed the transition from monolithic to microservices, offering enhanced scalability, maintainability, and flexibility. With the rise of Microservices Architecture (MA), containerization has emerged as a pivotal technology to encapsulate microservices in isolated environments, ensuring consistent deployment. This paper delves into the intricate relationship between Microservices Architecture and containerization, focusing on the benefits, challenges, and practical implications of integrating both. Through a comprehensive experimental setup simulating an e-commerce platform, we quantitatively evaluate the performance metrics of a containerized microservices system versus a traditional monolithic setup. Our findings accentuate the performance gains achieved through MA and containerization, while also shedding light on areas that demand caution and further research. The insights presented serve as a beacon for organizations aiming to transition to or optimize their microservices and containerization practices.
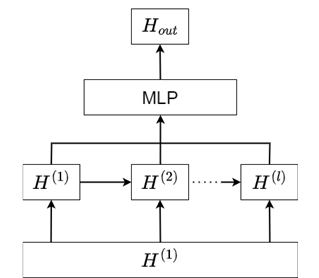
In order to address the issues of predefined adjacency matrices inadequately representing information in road networks, insufficiently capturing spatial dependencies of traffic networks, and the potential problem of excessive smoothing or neglecting initial node information as the layers of graph convolutional neural networks increase, thus affecting traffic prediction performance, this paper proposes a prediction model based on Adaptive Multi-channel Graph Convolutional Neural Networks (AMGCN). The model utilizes an adaptive adjacency matrix to automatically learn implicit graph structures from data, introduces a mixed skip propagation graph convolutional neural network model, which retains the original node states and selectively acquires outputs of convolutional layers, thus avoiding the loss of node initial states and comprehensively capturing spatial correlations of traffic flow. Finally, the output is fed into Long Short-Term Memory networks to capture temporal correlations. Comparative experiments on two real datasets validate the effectiveness of the proposed model.
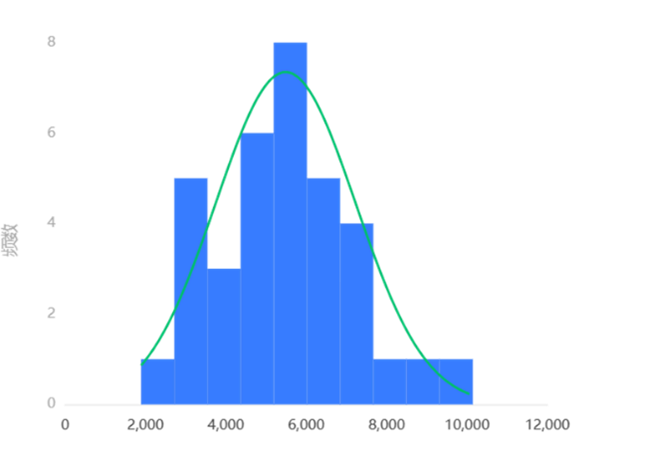
This paper addresses the issues of replenishment and pricing of vegetable commodities in fresh food supermarkets. By analyzing sales data and loss rate data, mathematical models are established to solve the following problems. Spearman correlation analysis and cluster analysis methods are employed. Firstly, data preprocessing is conducted by excluding returned vegetable products from a certain supermarket’s distribution, and then integrating sales transaction details and wholesale price-related data. Secondly, monthly data is used to succinctly present the sales distribution patterns of various categories and individual items of vegetables through line charts. Finally, the models of Spearman correlation analysis and cluster analysis are respectively applied to illustrate the relationship between the sales volume of 6 major categories of vegetables and 251 individual items. Linear regression models, time series analysis, and XG-Boost regression analysis models are used. This paper requires replenishment plans to be made by category, analyzing the relationship between the total sales volume and cost-plus pricing of various vegetable categories. Firstly, a linear regression model is applied to fit historical sales volume and cost-plus pricing, resulting in a linear functional relationship, indicating that only the sales volume of tomatoes is negatively correlated with cost-plus pricing. Based on historical sales data, the daily replenishment total for each vegetable category within the next week is predicted. Predictive models and time series analysis are employed. Meanwhile, for pricing strategies, the XGBoost algorithm is utilized to provide reasonable pricing strategies. Based on the optimal solution, replenishment plans and pricing strategies for the next week are formulated to ensure the maximization of sales revenue objectives.
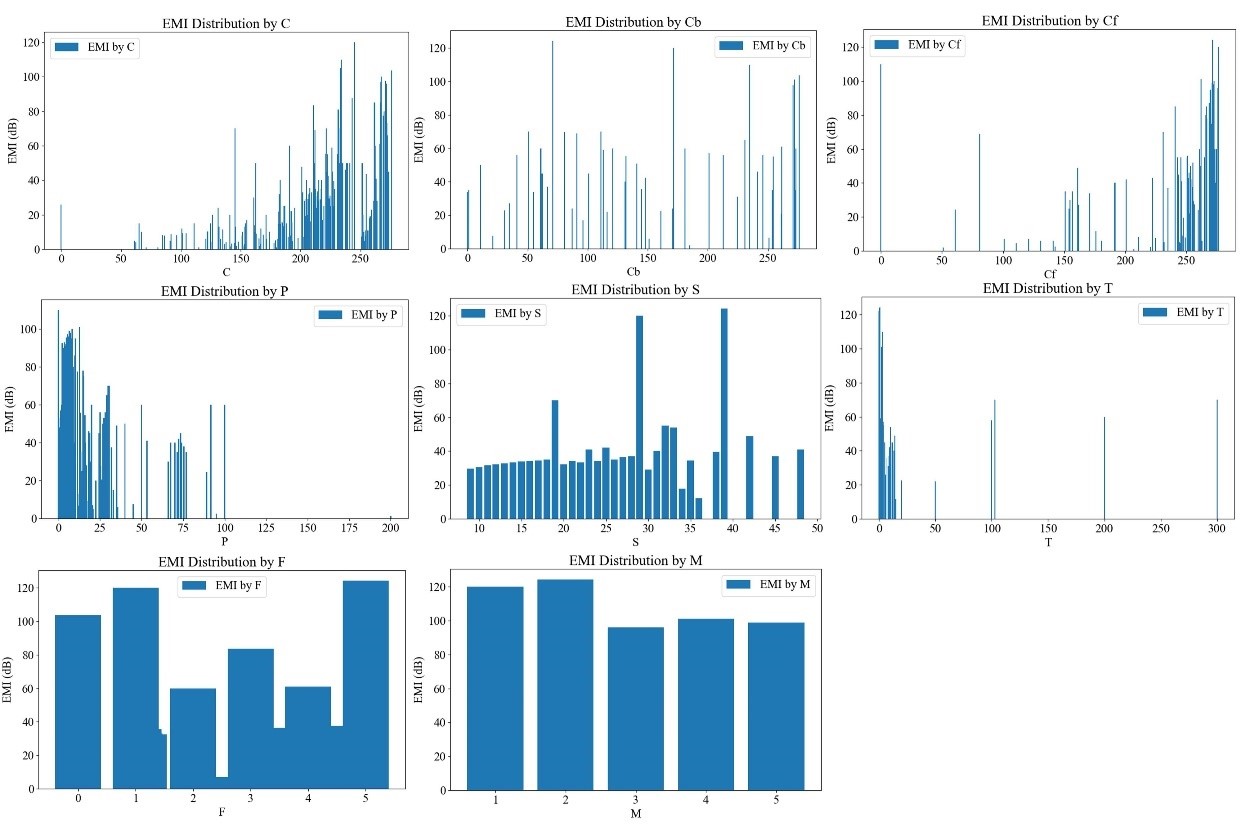
The compilation and preprocessing of electromagnetic shielding material databases using machine learning techniques are pivotal in contemporary materials science and engineering. These materials play a crucial role in diverse applications such as electronics, telecommunications, aerospace, and automotive industries, necessitating effective attenuation of electromagnetic interference (EMI). This paper underscores the significance of comprehensive databases in organizing material properties systematically, facilitating the identification of novel materials with enhanced shielding effectiveness. It explores the role of machine learning algorithms in predictive modeling and data analysis, expediting the screening process of candidate materials and uncovering hidden correlations within complex datasets. However, the efficacy of machine learning techniques relies heavily on the quality of input data and preprocessing steps. Thus, this paper discusses methodologies for collecting material data, challenges in data curation and integration, and common preprocessing techniques such as data cleaning, feature extraction, and normalization. The integration of electromagnetic shielding material databases with machine learning preprocessing holds great promise for FeCo-based alloy laser cladding manufacturing and design, leading to innovative solutions for electromagnetic interference mitigation across various technological domains.