Volume 87
Published on August 2024Volume title: Proceedings of the 6th International Conference on Computing and Data Science
This paper systematically builds a theoretical framework for enhancing data integrity and privacy protection by analyzing the fundamentals of blockchain technology and its inherent characteristics, such as decentralization, tamperability, and cryptographic algorithms. The study empirically examines the transparency and anonymity balance mechanism of blockchain in handling sensitive data using case studies and simulation experiments, and at the same time, designs smart contracts to automatically implement data protection strategies for different types of data security threats.
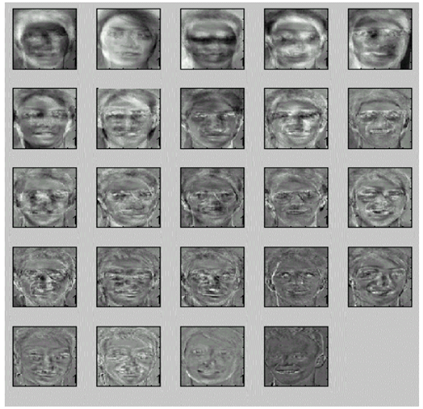
This paper analyses the main technologies for face recognition, a critical biometric tool for identity verification and security across various sectors. A comprehensive overview of traditional and modern facial recognition technologies will be provided, examining their key features such as age, pose, and illumination. The study discusses the evolution and current state of facial recognition, highlighting significant advancements and applications in recent years. The objective is to offer a detailed understanding of how these technologies function and their implications for security and identity verification.
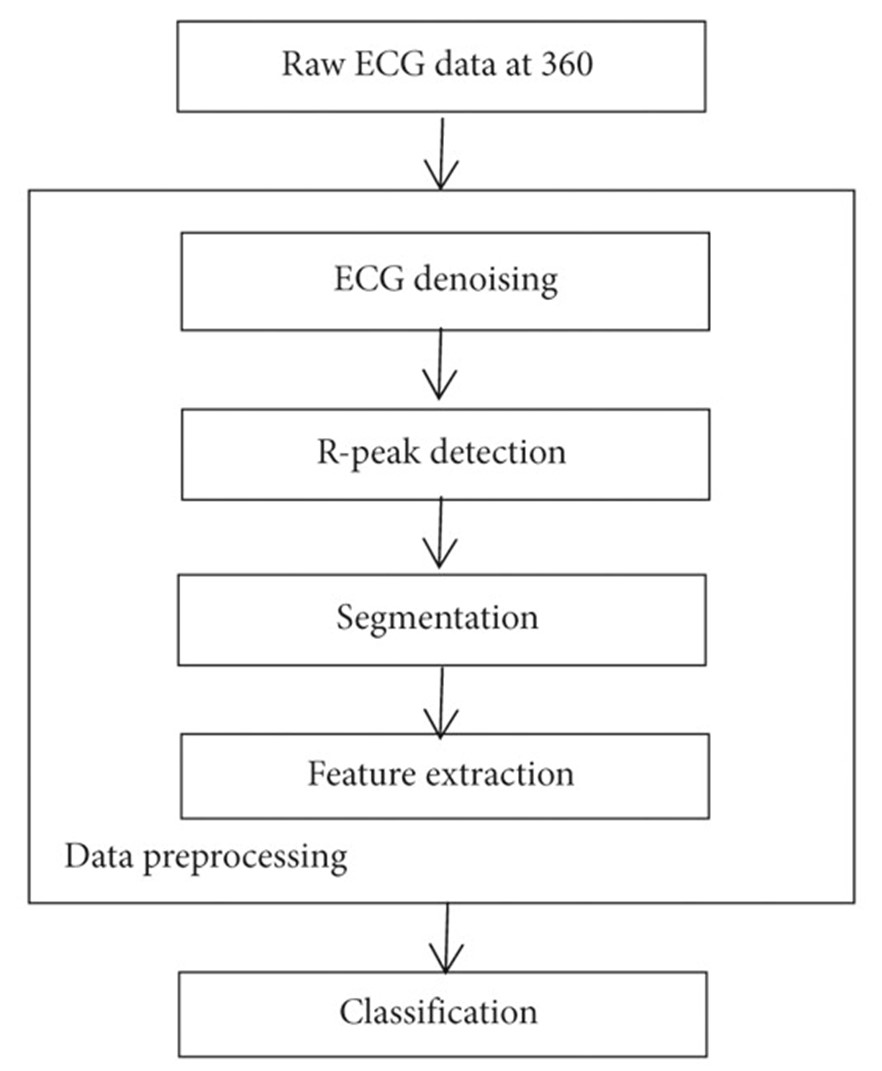
Heart is the most important part of the human body, diseases that are related to heart cause a huge thread to human health. In this paper, methods that applied Apache Spark to heart disease related works would be shown and discussed in order to classify these methods and make a conclusion about the innovations and shortcomings of these works. These works are defined into two categories: the ones that adopted traditional machine learning method and the ones that used deep learning methods. By classifying these works into two types, commonalities and similar innovative approaches in the same category of methods can be better observed and summarized, facilitating a clearer comparison of the similarities and differences in the innovative focuses among similar yet distinct methods. By doing so, conclusions were made to show that apart from enhancing operational efficiency and reliability of models for diagnosing and treating heart diseases, current research utilizing Apache Spark in this field also identifies areas for improvement such as expanding sample data representation, speeding up data processing, and addressing concept drift issues with proposed solutions. By addressing these challenges, researchers aim to optimize existing methods using Apache Spark and advanced data analytics techniques to combat heart diseases.
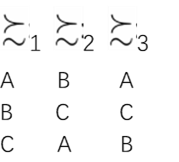
In China, patients are given the opportunity to know background information about their doctors, and patients choose their doctors to see. Due to the shortage of medical resources and the uncertainty of the quality of medical services, as well as some external factors, patients will prefer to choose well-known doctors in the hope of getting a better medical experience. In order to match the preferred healthcare resources, they may choose to lie to increase the chance of visiting the doctor. This study adopts a combination of theoretical modeling and algorithmic simulation. Through theoretical analysis, a framework model of doctor-patient matching is established. Doctors with different skills and experience, who select patients according to their conditions and their own preferences in the matching process, as well as patients with different conditions and preferences, who want to receive treatment by matching to a suitable doctor, are clarified. The Deferred Acceptance algorithm is written and operated to simulate the matching process where patients apply to doctors and doctors are screened based on their priorities. Analyze and evaluate the performance of Strategy-Proofness and Pareto Efficiency in matching by iterating the algorithm. In this case, the DA algorithm establishes a stable match between the patient and the physician despite the possibility that the patient may deceive his/her preferences. However, patient behavior may affect the efficiency and fairness of the matching process, highlighting the importance of transparency and integrity of the doctor-patient matching system.
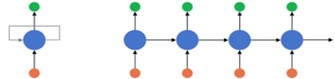
This paper explores the application of machine learning in financial time series analysis, focusing on predicting trends in financial enterprise stocks and economic data. It begins by distinguishing stocks from stocks and elucidates risk management strategies in the stock market. Traditional statistical methods such as ARIMA and exponential smoothing are discussed in terms of their advantages and limitations in economic forecasting. Subsequently, the effectiveness of machine learning techniques, particularly LSTM and CNN-BiLSTM hybrid models, in financial market prediction is detailed, highlighting their capability to capture nonlinear patterns in dynamic markets. Finally, the paper outlines prospects for machine learning in financial forecasting, laying a theoretical foundation and methodological framework for achieving more precise and reliable economic predictions.
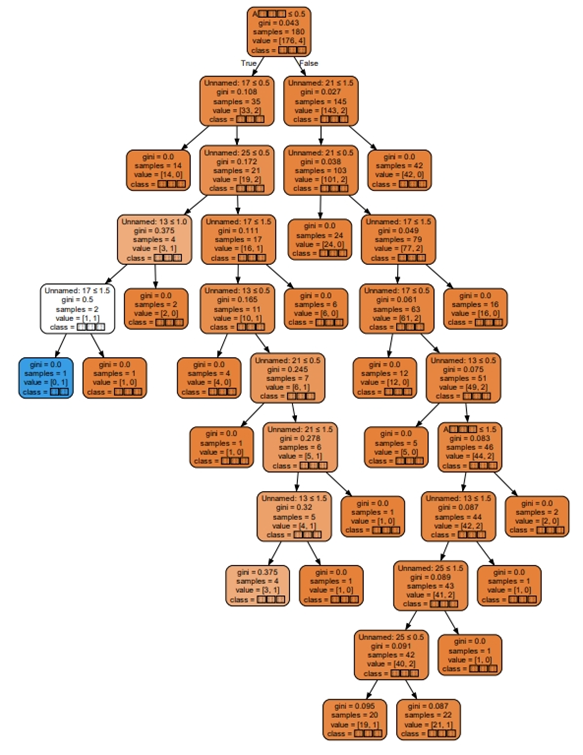
With the development of AI technology, generative AI has gradually entered the life of the public, for example, the explosion of CHAT-GPT has allowed more people to see the huge potential and obvious advantages of generative AI. However, in the process of generative AI operation, events that violate social responsibility and ethics often occur, which makes the research on the scientific and technological ethics of generative AI more urgent. In the past literature and research, many industry experts have analysed the impact of generative AI on specific industries, but everyone is or will be a user of generative AI, so we should pay attention to the study of the people's scientific and technological ethical issues of generative AI after putting aside the industry background, so this paper collects primary data by means of questionnaire surveys to find out the public's awareness of generative AI and their perception of generative AI. and attitudes towards generative AI, and using the decision tree C4.5 algorithm with Python as the tool, it is used to respond to people's awareness of generative AI and the public's perception of the relationship between the various factors of the ethical issues of
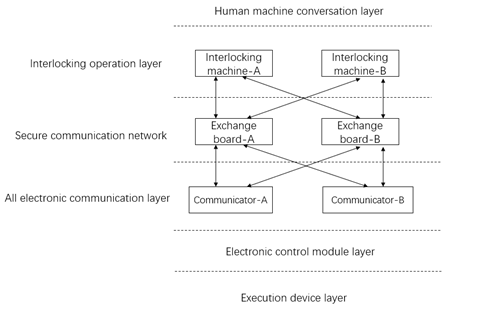
Communication security in fully electronic interlocking systems is one of the key factors ensuring the safe and reliable operation of the entire system. EN50159 is an important standard in the European railway communication sector, aimed at ensuring the safety, reliability, and efficiency of railway transportation. According to the communication security standards of EN50159, there are six types of security risks in closed communication: data duplication, deletion, insertion, misordering, delay, and corruption. This paper analyzes and explains the aspects of communication security that need to be considered based on the safety communication standards of China's railway signaling system and the signal safety standards in EN50159, focusing primarily on communication security and reliability.
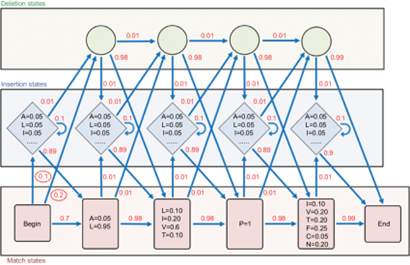
This paper delves into the intricate relationship between machine learning (ML) and data analysis, spotlighting the recent advancements, prevailing challenges, and emerging opportunities that underscore their integration. By conducting an extensive review of scholarly literature and real-world case studies, this article uncovers the synergistic potential of ML and data analysis, emphasizing their combined influence across diverse industries and domains. The exploration is framed around pivotal themes including algorithmic innovations, which are at the heart of ML's ability to transform vast and complex datasets into actionable insights. Moreover, the discussion extends to predictive modeling techniques, a cornerstone of data analysis that leverages historical data to forecast future trends, behaviors, and outcomes. Practical applications are scrutinized to demonstrate how the confluence of ML and data analysis is pioneering solutions in fields as varied as healthcare, where predictive analytics can save lives, to finance, where it is used to navigate market uncertainties. This paper also addresses the barriers to effective integration, such as data privacy concerns and the need for robust data governance frameworks. Through this comprehensive examination, the article sheds light on the rapidly evolving landscape of ML-driven data analysis, offering insights into how these technological advancements are reshaping research methodologies, industry practices, and societal norms.
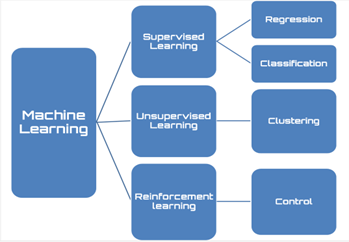
This paper investigates the application of artificial intelligence in the financial sector, analyzing the existing technical, ethical, and legal issues, and proposing corresponding solutions. The research background highlights the widespread use of AI technology in the financial industry and the efficiency and cost benefits it brings. The research focuses on challenges related to data quality, feature engineering, model complexity, real-time capability, computational resources, and data privacy protection. The research method includes literature review and case analysis, revealing the applications of AI technology in stock prediction, risk management, trading strategy optimization, and customer service. The results indicate that effective data cleaning, automated feature engineering, model simplification and regularization techniques, the use of interpretability tools like LIME and SHAP, and the introduction of fairness evaluation standards can significantly enhance AI model performance and transparency. The conclusion points out that these measures can not only solve the current technical and ethical issues of AI in the financial sector but also promote the widespread application and standardization of AI technology in other fields.
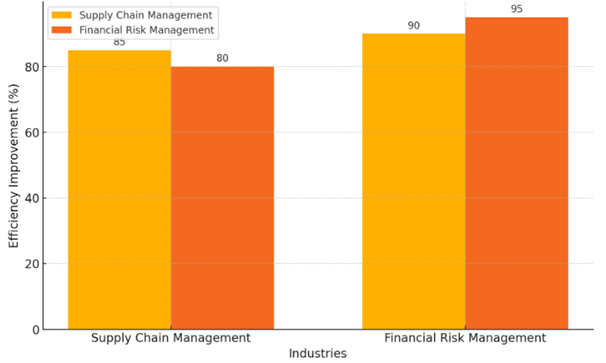
This paper investigates the integration of stochastic processes and reinforcement learning (RL) in strategic decision support systems (SDSS) and personalized advertisement recommendations. Stochastic processes offer a robust framework for modeling uncertainties and predicting future states across various domains, while RL facilitates dynamic optimization through continuous interaction with the environment. The combination of these technologies significantly enhances decision-making accuracy and efficiency, yielding substantial benefits in industries such as financial services, healthcare, logistics, retail, and manufacturing. By leveraging these advanced AI techniques, businesses can develop adaptive strategies that respond to real-time changes and optimize outcomes. This paper delves into the theoretical foundations of stochastic processes and RL, explores their practical implementations, and presents case studies that demonstrate their effectiveness. Furthermore, it addresses the computational complexity and ethical considerations related to these technologies, providing comprehensive insights into their potential and challenges. The findings highlight the transformative impact of integrating stochastic processes and RL in contemporary decision-making frameworks.