Volume 93
Published on November 2024Volume title: Proceedings of the 2nd International Conference on Machine Learning and Automation
Autonomous driving is gradually becoming one of the major directions in the development of automotive technology nowadays. Environmental detection technology is indispensable for existing intelligent vehicles, especially when such cars are used in daily life, where many complex road environments cannot be helped by environmental detection technology. Environmental detection technology cannot be divorced from hardware and software support. This study will discuss the different sensors used in autonomous driving environment detection technology, to gain a deeper understanding of the characteristics, functions and applications of these sensors, and to discuss the advantages and limitations of each sensor. Then a comparison of the three widely used sensors in the world is conducted, in terms of the detection range and angle, the accuracy of road detection, and the stability of the detection. The three sensors are a camera, millimeter wave radar and laser radar. A more suitable and stable one from these three sensors will be chosen for in-depth consideration. On this basis, new ideas for the future development of existing sensors are provided, while the direction of improvement of existing sensors is summarized based on the results of existing analyses.
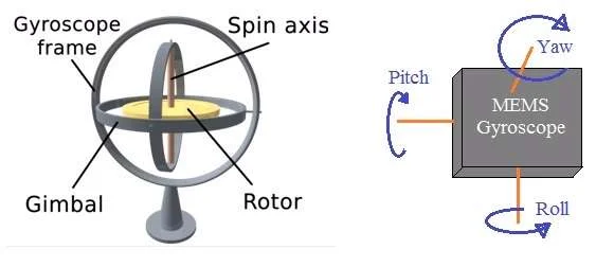
A sensor is a device that receives signals and responds to the signals or stimuli. The output of the sensors can be the voltage or current. The input of the sensors is very complex. For different types of sensors, there are different types of input. All sensors are categorized based on their applications and principles. In this article, there are mainly two major categories of sensors in the field of engineering included. One is the localization. The localization mainly includes visual sensors, infrared sensors, lidar and ultrasonic sensors. This paper discusses the application scenarios as well as the advantages and disadvantages of each kind of sensor. The SLAM control algorithm for robotics based on sensors will also be addressed. Another focus of this paper is robot control. It only includes a major type of sensor which is the inertial sensor. For this part, the article mainly discusses the applications and advantages & disadvantages of the gyroscope sensor. Also, the prevailing robotics control algorithm- PID control is illustrated. In a more complex environment in engineering, a single sensor may not meet the requirements of the engineers. So, the combination use of the sensors appears. This article also explores the use of machine learning for intelligent sensor design.
This paper examines the perception systems used in industrial Automated Guided Vehicles (AGVs), focusing on traditional and advanced sensor solutions. Traditional perception methods, such as track-based and magnetic tape guidance, offer reliability but are limited in flexibility. In contrast, radar, vision, and LiDAR sensors provide enhanced perception capabilities, enabling AGVs to navigate safely and efficiently in complex industrial environments. The study explores various sensors, including visible light, infrared, ultrasonic, LiDAR, magnetic strip sensors, Inertial Measurement Units (IMUs), tactile sensors, Ultrawideband (UWB) sensors, thermal sensors, and millimeter-wave (mmWave) sensors, highlighting their principles, advantages, and limitations. The integration of these sensors supports robust navigation and operational efficiency in diverse settings. The methodology involves reviewing existing literature and analyzing current technologies used in industrial AGVs. Results indicate that while traditional solutions are reliable, advanced sensor technologies significantly enhance AGV performance. The paper concludes that the future of AGV perception systems lies in the integration of advanced sensors with artificial intelligence and machine learning algorithms, promoting intelligent and adaptive industrial automation. Additionally, it underscores the necessity of developing robust sensor fusion techniques to harness the full potential of these advanced sensors.
This paper compares three Simultaneous Localization and Mapping (SLAM) algorithms. SLAM algorithms are the core technology for autonomous navigation and environmental perception of mobile robots. SLAM algorithms are used by mobile robots to perceive the surrounding environment, build up an environment map and position themselves in real-time in an unknown environment. This article first systematically reviews the basic principles of each algorithm based on experiments and studies that have been completed by previous researchers and illustrates their respective unique mechanisms for processing sensor data, map construction, and localization. Subsequently, this paper analyzes the performance differences and characteristics of the three algorithms in practical applications in terms of robustness in complex environments, consumption of computing resources, and accuracy for generated maps. Finally, based on the advantages and disadvantages of each analyzed algorithm, this article summarizes the most suitable and unsuitable usage scenarios of different algorithms in specific situations. Moreover, this article puts forward specific algorithm selection suggestions for different scenarios to help engineers and researchers make more appropriate decisions in actual projects.
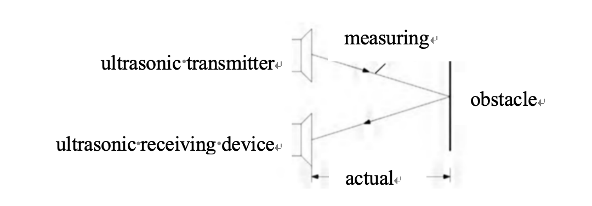
With the progress of science and technology, more and more cars have the function of automatic driving. After reviewing a series of articles, it is found that there are still many problems in the research on automatic driving. These include problems with sensors, cameras, navigation systems, signals and so on. The primary focus of this article is the automobile's sensor system, which includes body-sensing sensors, radar sensors, vision sensors, and GPS systems. However, there will be numerous issues if these sensors are used alone. It can be seen that many researchers have done the application of multiple sensors and achieved good results. Thus, in order to enable the widespread adoption of automated driving in the future, it is advised to integrate two or three sensors and conduct testing through real-world applications. This can not only reduce the occurrence of accidents but also promote the broader development of autonomous driving.
As society develops, people are paying more attention to disabilities. Visually impaired people as a group, are one of the largest populations of disabilities. Nowadays, scientists are finding approaches to give them more convenience. Robotic guide dogs, which aid the visually impaired to travel, becoming a standout topic in society. As an emerging technology in recent years, artificial intelligence (AI) gives technical support to the study of robotic guide dogs. By examining a number of current robotic guide dog models, this paper will demonstrate how AI can be used to improve robotic guide dogs in a number of ways. The research shows that AI plays an important role in the operation of the robotic guide dog. This research will also point out some directions for future studies.
Vertical takeoff and landing (VTOL) technology is garnering significant attention in the aviation industry due to its distinct benefits and wide range of potential applications. A long runway is not necessary when using VTOL technology, which allows aircraft to take off and land vertically straight from the ground. Air mobility in cities, military activities, and emergency response are just a few of the areas where this technology is beneficial. This essay will provide an overview of the development, application, and future of vertical takeoff and landing technology. The technology’s operating principles, advantages and limitations will also be explored. Overall, VTOL technology offers a tremendous leap in aircraft technology, with numerous possible uses. Even though there are still difficulties, more research and development will probably result in improvements and broader use.
This paper provides a comprehensive review of advancements in Positioning and Navigation, focusing on the integration of key technologies to enhance operational efficiency and sustainability. The study examines the application of sensors in robotic systems, with particular emphasis on visual Simultaneous Localization and Mapping (SLAM) and LiDAR SLAM technologies. Visual SLAM, which uses monocular or binocular cameras to capture environmental images for navigation and mapping, offers cost-effective solutions but faces challenges such as sensitivity to lighting conditions and high computational demands. LiDAR SLAM, utilizing laser pulses to create precise 3D maps, complements visual SLAM by improving accuracy and robustness. The review also covers the integration of these technologies with deep learning and other advanced algorithms, highlighting their potential to enhance robotic performance and address practical limitations. The paper identifies future research directions aimed at advancing these technologies for more effective and sustainable practices.
As mobile robots are widely used in daily life, industrial manufacturing and the military, their ability to autonomous navigation in unmanned platforms and a wide range of environments is increasingly demanding. Therefore, the selection of sensors is a necessary process to improve the efficiency of navigation. The paper will introduce the principles and advantages of monocular vision, LIDAR and ultrasonic sensors etc. in detail, and then explore the advantages and disadvantages of various algorithms, and finally conclude optimal fusion of sensor solutions. The comparison results present that for the monocular vision, acquiring an image from a single camera setup, then using a YOLOv5 and mosaic technology to form a single image and finally using the improved RRT and the Frenet coordinate system to model the path is an efficient solution. 3D LIDAR technology can use the SLAM framework of graph optimization to create the map for obstacle avoidance and path planning. At last, this paper provides suggestions and optimizations for mobile robot navigation solutions, which are integrating multiple sensors and combining navigation solutions with machine learning.
Unmanned Aerial Vehicles (UAVs) have significantly impacted various industries, with electro-optical (EO) pods playing a crucial role in enhancing their functionality. These pods require advanced stabilization algorithms to maintain a stable line of sight (LOS) in dynamic environments. This paper reviews the evolution of self-stabilization algorithms used in UAV EO pods, from traditional Proportional-Integral-Derivative (PID) controllers to advanced Kalman filters and machine learning techniques. Through a comprehensive analysis, the paper explores the application of these algorithms in different scenarios, highlighting their importance in both military and civilian domains. The findings provide insights into optimizing EO pod performance, ensuring high-quality imaging and precise targeting in various UAV operations.