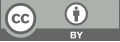
Stock Price Prediction Based on Linear Regression
- 1 School of Mathematics and Statistics, Huazhong University of Science and Technology, Wuhan, Hubei, 430074, China
* Author to whom correspondence should be addressed.
Abstract
Stock market price prediction is one of the core subjects in the financial field. With the increase in market complexity and volatility, accurate stock price prediction has important decision-making significance for investors, financial analysts, and related institutions. This paper aims to use a linear regression model to analyze the historical data of Samsung Electronics stock, and then predict its future stock price trend. The research begins with a pre-processing of the original data set, including data cleaning and feature engineering, to remove noise data and select valuable feature variables. Then, a linear regression model was used to build a stock price prediction model, and the prediction effect of the model was evaluated using mean square error (MSE) to measure the accuracy of the forecast results. To further verify the validity of the model, this paper also makes a comparative analysis of the actual stock price and the predicted stock price and reveals the performance of the model in practical application through error analysis. The results show that the linear regression model can reflect the trend change in stock price to a certain extent. However, its prediction accuracy is still affected by many factors, suggesting that more complex models or technologies should be introduced for further optimization in the future.
Keywords
Stock forecasting, linear regression, stock price, data analysis, mean square error
[1]. Li, X., & Xia, J. (2023). Research on stock price regression prediction based on machine learning algorithm. Science and Technology Information, 21(14), 227-231.
[2]. Wang, L., He, Y., & Jiao, D. (2023). Prediction of stock price fluctuation trend based on logistic regression model: A case study of Bank of Guiyang. China Management Information Technology, 26(4), 156-158.
[3]. Ma, G., & Tang, Y. (2023). Prediction of stock trend in the agriculture, forestry, animal husbandry, and fishery industry based on machine learning. Investment and Cooperation, (6), 47-49.
[4]. Zhang, X., & Chen, L. (2022). Design and implementation of hot stock analysis and recommendation system based on linear regression. Modern Information Technology, 6(22), 16-21.
[5]. Zhao, Y., Tang, Y., & Jiang, Z. (2023). Study on the change of human body weight step length based on linear regression method. Journal of Liaoning Police University, 25(4), 57-62.
[6]. He, X., & Duan, F. (2022). Case analysis of linear regression based on Python. Microcomputer Applications, 38(11), 35-37.
[7]. Poon, S. H., & Chan, K. C. (2008). Forecasting stock returns: A comparison of linear and non-linear models. Journal of Financial and Quantitative Analysis, 43(3), 673-698.
[8]. Tsai, C. F., & Chen, M. Y. (2015). A survey of stock market prediction with big data. Proceedings of the 10th International Conference on Machine Learning and Computing, 125-130.
[9]. Zhang, Y., & Zhou, W. (2017). Stock market prediction using linear regression and LSTM: A hybrid model. Proceedings of the 2017 International Conference on Computational Intelligence and Applications, 85-92.
[10]. Gupta, A., & Gupta, V. (2020). Stock price prediction using linear regression model with multiple features. International Journal of Computer Applications, 175(4), 23-29.
[11]. Huang, S., & Chen, M. (2016). Predicting stock market trends using multiple linear regression models. Expert Systems with Applications, 46, 50-58.
Cite this article
Zhao,Y. (2025). Stock Price Prediction Based on Linear Regression. Theoretical and Natural Science,84,85-90.
Data availability
The datasets used and/or analyzed during the current study will be available from the authors upon reasonable request.
Disclaimer/Publisher's Note
The statements, opinions and data contained in all publications are solely those of the individual author(s) and contributor(s) and not of EWA Publishing and/or the editor(s). EWA Publishing and/or the editor(s) disclaim responsibility for any injury to people or property resulting from any ideas, methods, instructions or products referred to in the content.
About volume
Volume title: Proceedings of the 4th International Conference on Computing Innovation and Applied Physics
© 2024 by the author(s). Licensee EWA Publishing, Oxford, UK. This article is an open access article distributed under the terms and
conditions of the Creative Commons Attribution (CC BY) license. Authors who
publish this series agree to the following terms:
1. Authors retain copyright and grant the series right of first publication with the work simultaneously licensed under a Creative Commons
Attribution License that allows others to share the work with an acknowledgment of the work's authorship and initial publication in this
series.
2. Authors are able to enter into separate, additional contractual arrangements for the non-exclusive distribution of the series's published
version of the work (e.g., post it to an institutional repository or publish it in a book), with an acknowledgment of its initial
publication in this series.
3. Authors are permitted and encouraged to post their work online (e.g., in institutional repositories or on their website) prior to and
during the submission process, as it can lead to productive exchanges, as well as earlier and greater citation of published work (See
Open access policy for details).