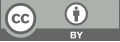
Boosting Extreme Weather Prediction by Fine-tuning a Pre-Trained Large Model: A Study on GraphCast
- 1 Shanghai High School International Division, Shanghai, China
* Author to whom correspondence should be addressed.
Abstract
Large-scale deep learning weather prediction models are revolutionizing the field of weather forecasting. GraphCast is the current state-of-the-art model, but its training is not explicitly designed for predicting extreme weather events. However, extreme weather prediction is more critical because it directly impacts public safety, potentially saving a lot of lives and resources. This paper improves large-scale weather models such as GraphCast by introducing an uncertainty estimation module to differentiate the importance of extreme weather data. We hypothesize and demonstrate that regions with higher uncertainty are more prone to cause prediction errors. By fine-tuning large-scale weather prediction models such as GraphCast with our uncertainty-aware weighting method, we enhance extreme weather forecasting in extreme cases where predictions were previously poor. Our approach provides a pathway for more accurate extreme weather forecasts and a pipeline for future model fine-tuning efforts.
Keywords
Weather Forecasting, Deep Learning, Extreme Weather, GraphCast, Large Model
[1]. https://www.cbsnews.com/news/climate-change-extreme-weather-events-deaths/
[2]. United Nations. “Climate Action and Synergies | Department of Economic and Social Affairs.” Sdgs.un.org, 2023, sdgs.un.org/topics/climate-action-synergies.
[3]. https://www.microsoft.com/en-us/research/blog/introducing-aurora-the-first-large-scale-foundation-model-of-the-atmosphere/?msockid=151aa37cc04a60e0180bb207c130615e
[4]. Rasp, S., Dueben, P. D., Scher, S., Weyn, J. A., Mouatadid, S., & Thuerey, N. (2020). WeatherBench: a benchmark data set for data‐driven weather forecasting. Journal of Advances in Modeling Earth Systems, 12(11), e2020MS002203.
[5]. Lam, R., Sanchez-Gonzalez, A., Willson, M., Wirnsberger, P., Fortunato, M., Alet, F., ... & Battaglia, P. (2023). Learning skillful medium-range global weather forecasting. Science, 382(6677), 1416-1421.
[6]. Conti, S. (2024). Artificial intelligence for weather forecasting. Nature Reviews Electrical Engineering, 1(1), 8-8.
[7]. Lopez-Gomez, I., McGovern, A., Agrawal, S., & Hickey, J. (2023). Global extreme heat forecasting using neural weather models. Artificial Intelligence for the Earth Systems, 2(1).
[8]. Bi, K., Xie, L., Zhang, H., Chen, X., Gu, X., & Tian, Q. (2023). Accurate medium-range global weather forecasting with 3D neural networks. Nature, 619(7970), 533-538.
[9]. Keisler, R. (2022). Forecasting global weather with graph neural networks. arXiv preprint arXiv:2202.07575.
[10]. Zhong, X., Chen, L., Liu, J., Lin, C., Qi, Y., & Li, H. (2024). FuXi-Extreme: Improving extreme rainfall and wind forecasts with diffusion model. Science China Earth Sciences, 1-13.
[11]. Cohen, T. S., Geiger, M., Köhler, J., & Welling, M. (2018, February). Spherical CNNs. In International Conference on Learning Representations.
[12]. Kochkov, D., Yuval, J., Langmore, I., Norgaard, P., Smith, J., Mooers, G., ... & Hoyer, S. (2024). Neural general circulation models for weather and climate. Nature, 632(8027), 1060-1066.
[13]. Zhao, J., Lu, C. T., & Kou, Y. (2003, November). Detecting region outliers in meteorological data. In Proceedings of the 11th ACM international symposium on Advances in geographic information systems (pp. 49-55).
[14]. Porto, F., Ferro, M., Ogasawara, E., Moeda, T., de Barros, C. D. T., Silva, A. C., ... & Bezerra, E. (2022). Machine learning approaches to extreme weather events forecast in urban areas: Challenges and initial results. Supercomputing Frontiers and Innovations, 9(1), 49-73.
[15]. Annau, N. J.; Cannon, A. J.; and Monahan, A. H. 2023. Algorithmic hallucinations of near-surface winds: Statistical downscaling with generative adversarial networks to convection permitting scales. Artificial Intelligence for the Earth Systems, 2(4): e230015.
[16]. Morozov, V.; Galliamov, A.; Lukashevich, A.; Kurdukova, A.; and Maximov, Y. 2023. CMIP X-MOS: Improving Climate Models with Extreme Model Output Statistics. arXiv preprint arXiv:2311.03370.
[17]. Lopez-Gomez, I.; McGovern, A.; Agrawal, S.; and Hickey, J. 2023. Global extreme heat forecasting using neural weather models. Artificial Intelligence for the Earth Systems, 2(1): e220035.
[18]. Wang, H.-W.; Peng, Z.-R.; Wang, D.; Meng, Y.; Wu, T.; Sun, W.; and Lu, Q.-C. 2020. Evaluation and prediction of transportation resilience under extreme weather events: A diffusion graph convolutional approach. Transportation research part C: emerging technologies, 115: 102619.
[19]. Ni, Z. 2023. Kunyu: A High-Performing Global Weather Model Beyond Regression Losses. arXiv preprint arXiv:2312.08264.
[20]. Berard, H.; Gidel, G.; Almahairi, A.; Vincent, P.; and LacosteJulien, S. 2019. A closer look at the optimization landscapes of generative adversarial networks. arXiv preprint arXiv:1906.04848.
[21]. Ding, N., Qin, Y., Yang, G., Wei, F., Yang, Z., Su, Y., ... & Sun, M. (2023). Parameter-efficient fine-tuning of large-scale pre-trained language models. Nature Machine Intelligence, 5(3), 220-235.
Cite this article
Zhan,H. (2025). Boosting Extreme Weather Prediction by Fine-tuning a Pre-Trained Large Model: A Study on GraphCast. Theoretical and Natural Science,100,83-94.
Data availability
The datasets used and/or analyzed during the current study will be available from the authors upon reasonable request.
Disclaimer/Publisher's Note
The statements, opinions and data contained in all publications are solely those of the individual author(s) and contributor(s) and not of EWA Publishing and/or the editor(s). EWA Publishing and/or the editor(s) disclaim responsibility for any injury to people or property resulting from any ideas, methods, instructions or products referred to in the content.
About volume
Volume title: Proceedings of the 3rd International Conference on Mathematical Physics and Computational Simulation
© 2024 by the author(s). Licensee EWA Publishing, Oxford, UK. This article is an open access article distributed under the terms and
conditions of the Creative Commons Attribution (CC BY) license. Authors who
publish this series agree to the following terms:
1. Authors retain copyright and grant the series right of first publication with the work simultaneously licensed under a Creative Commons
Attribution License that allows others to share the work with an acknowledgment of the work's authorship and initial publication in this
series.
2. Authors are able to enter into separate, additional contractual arrangements for the non-exclusive distribution of the series's published
version of the work (e.g., post it to an institutional repository or publish it in a book), with an acknowledgment of its initial
publication in this series.
3. Authors are permitted and encouraged to post their work online (e.g., in institutional repositories or on their website) prior to and
during the submission process, as it can lead to productive exchanges, as well as earlier and greater citation of published work (See
Open access policy for details).