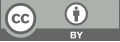
TSGAN: Individual treatment effect estimation for multi-intervention with continuous dosage
- 1 Sichuan University
- 2 Sichuan University
- 3 Shanghai Pinghe School
- 4 The University of Melbourne Grattan Street
- 5 University of Central Oklahoma
- 6 University of Central Oklahoma
* Author to whom correspondence should be addressed.
Abstract
In recent years, causal inference has achieved great results in recommendation systems, causal chain analysis, and individual treatment effects. The individual treatment effect (ITE), also known as the complier average treatment effect (CATE), is the focus of research in the medical, economic, and political fields. Its purpose is to solve the problem that it is impossible to predict an intervention's impact on individuals when interventions’ effects vary due to individual differences. Today's research focuses on estimating the counter-fact, that is, predicting the difference in treatment effect between individuals receiving one treatment and receiving another treatment. However, the above study was limited to two interventions and did not consider the issue of therapeutic dose. In this paper, a method combining both the idea of matching that prevalent in traditional ITE estimation, and a generative adversarial neural network (GAN) is proposed to achieve individual effect estimation under multi-intervention with continuous dosage intervention. This paper first proposes the idea of treatment effect space (TES), and proposes a neural network based on GAN, uses an improved discriminator, which takes a different approach from common GAN, using multiple discriminators in parallel structure to achieve discrimination of true samples from treatment space. The model was tested and validated under semi-simulated data.
Keywords
Individual Treatment Effect, Casual Inference, GAN
[1]. PAUL R. ROSENBAUM, DONALD B. RUBIN, The central role of the propensity score in observational studies for causal effects, Biometrika, Volume 70, Issue 1, April 1983, Pages 41–55, https://doi.org/10.1093/biomet/70.1.41
[2]. Bica, Ioana & Jordon, James & Schaar, Mihaela. (2020). Estimating the Effects of Continuous-valued Interventions using Generative Adversarial Networks.
[3]. Austin, Peter C. An introduction to propensity score methods for reducing the effects of confounding in observational studies. Multivariate behavioral research, 46(3):399–424, 2011.
[4]. Funk,MicheleJonsson,Westreich,Daniel,Wiesen,Chris,Sturmer,Til, Brookhart, M Alan, and Davidian, Marie. Dou- ̈ bly robust estimation of causal effects. American journal of epidemiology, 173(7):761–767, 2011.
[5]. Pearl, Judea. Causality. Cambridge university press, 2009.
[6]. Rubin, Donald B. Causal inference using potential outcomes. Journal of the American Statistical Association, 2011.
[7]. Morgan, S. & Winship, C. (2015).Counterfactuals and Causal Inference: Methods and principles for social research. Cambridge: Cambridge University Press. Page 142.
[8]. Fredrik D. Johansson, Uri Shalit, and David Sontag. 2016. Learning representations for counterfactual inference. In Proceedings of the 33rd International Conference on International Conference on Machine Learning - Volume 48 (ICML'16). JMLR.org, 3020–3029.
[9]. Liuyi Yao, Sheng Li, Yaliang Li, Mengdi Huai, Jing Gao, and Aidong Zhang. 2018. Representation learning for treatment effect estimation from observational data. In Proceedings of the 32nd International Conference on Neural Information Processing Systems (NIPS'18). Curran Associates Inc., Red Hook, NY, USA, 2638–2648.
[10]. Shi, Claudia & Blei, David & Veitch, Victor. (2019). Adapting Neural Networks for the Estimation of Treatment Effects.
[11]. Uri Shalit, Fredrik D. Johansson, and David Sontag. 2017. Estimating individual treatment effect: generalization bounds and algorithms. In Proceedings of the 34th International Conference on Machine Learning - Volume 70 (ICML'17). JMLR.org, 3076–3085.
[12]. Yoon, Jinsung et al. “GANITE: Estimation of Individualized Treatment Effects using Generative Adversarial Nets.” ICLR (2018).
[13]. Nair, Naveen & Gurumoorthy, Karthik & Mandalapu, Dinesh. (2022). Individual Treatment Effect Estimation Through Controlled Neural Network Training in Two Stages.
[14]. Patrick Schwab, Lorenz Linhardt, Stefan Bauer, Joachim M Buhmann, and Walter Karlen. Learning counterfactual representations for estimating individual dose-response curves. arXiv, preprint arXiv:1902.00981, 2019.
Cite this article
Lu,H.;Zhang,X.;Bu,E.;Zhu,R.;Jiang,Y.;Tian,P. (2023). TSGAN: Individual treatment effect estimation for multi-intervention with continuous dosage. Theoretical and Natural Science,18,174-181.
Data availability
The datasets used and/or analyzed during the current study will be available from the authors upon reasonable request.
Disclaimer/Publisher's Note
The statements, opinions and data contained in all publications are solely those of the individual author(s) and contributor(s) and not of EWA Publishing and/or the editor(s). EWA Publishing and/or the editor(s) disclaim responsibility for any injury to people or property resulting from any ideas, methods, instructions or products referred to in the content.
About volume
Volume title: Proceedings of the 2nd International Conference on Computing Innovation and Applied Physics
© 2024 by the author(s). Licensee EWA Publishing, Oxford, UK. This article is an open access article distributed under the terms and
conditions of the Creative Commons Attribution (CC BY) license. Authors who
publish this series agree to the following terms:
1. Authors retain copyright and grant the series right of first publication with the work simultaneously licensed under a Creative Commons
Attribution License that allows others to share the work with an acknowledgment of the work's authorship and initial publication in this
series.
2. Authors are able to enter into separate, additional contractual arrangements for the non-exclusive distribution of the series's published
version of the work (e.g., post it to an institutional repository or publish it in a book), with an acknowledgment of its initial
publication in this series.
3. Authors are permitted and encouraged to post their work online (e.g., in institutional repositories or on their website) prior to and
during the submission process, as it can lead to productive exchanges, as well as earlier and greater citation of published work (See
Open access policy for details).