Volume 19
Published on October 2023Volume title: Proceedings of the 5th International Conference on Computing and Data Science
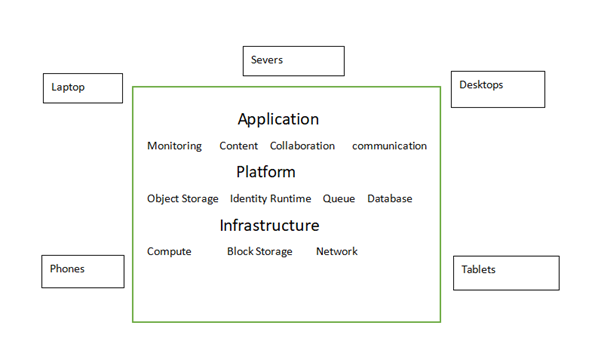
5G mobile communication network and cloud computing are the technological products and focus of today's era. Compared to 5G, 5G has seen a huge increase in peak speeds to 10-20Gbit/s, air interface latency as low as 1ms and much more. Cloud computing uploads data to the cloud so that users can access it more easily. They bring great convenience and high working efficiency to people's life. The use of cloud computing in 5G could make more efficient.5G, as a combination of new technology and cloud computing, will become a much larger market. This paper mainly describes the theoretical basis of 5G mobile communication network and cloud computing, the application of cloud computing in 5G (including automatic driving technology, surgery mobile communication network) and the current dilemma and the improvement needed. It aims to further promote the combination of 5G mobile communication network and cloud computing.
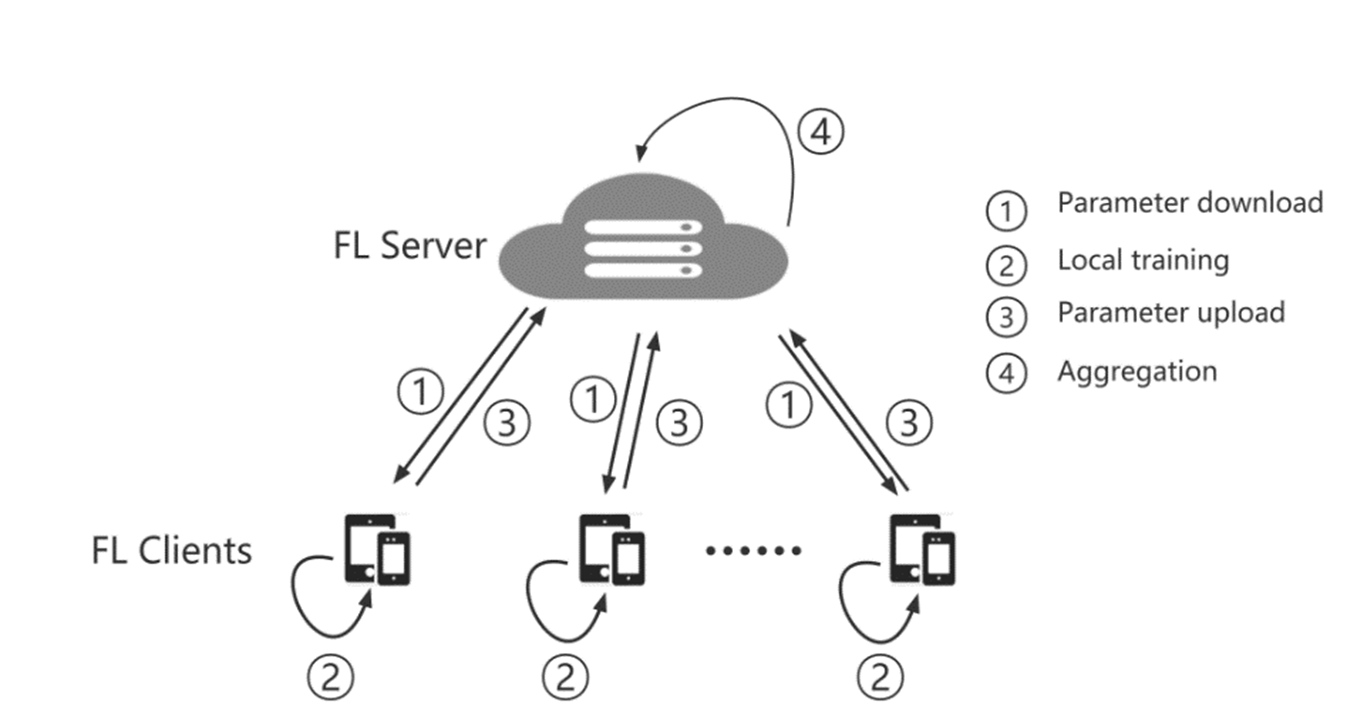
Federated learning allows you to train machine learning models without sharing your local data. Due to the No-iid problem, this paper is based on the Moon algorithm, which can have excellent performance in datasets of images with models that use deep learning and outperforms FedAvg, FedProx, and other algorithms, with the goal to decrease communication costs while enhancing efficiency more effectively. This study optimizes its gradient descent technique based on Moon's algorithm by utilizing Adaptive Gradient (AdaGrad) optimizer and combining with knowledge distillation to improve Moon's algorithm in order to better reduce communication costs and improve efficiency. That is, it reduces the loss and improves the accuracy faster and better in local training. In this paper, we experimentally show that the optimized moon can better solve the communication cost and improve the accuracy rate.
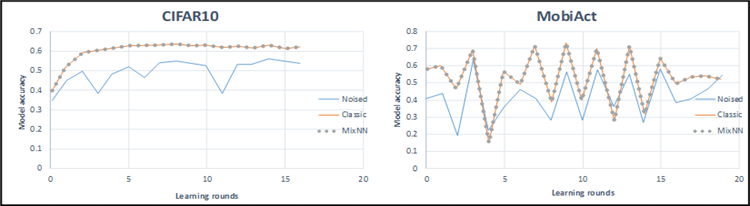
The FedFTG plug-in can effectively solve the problem of knowledge forgetting caused by the server-side direct aggregation model in Federated Learning. But FedFTG runs the risk of compromising customer privacy, as well as additional transmission costs. Therefore, this paper introduces methods to enhance the privacy and communication efficiency of FedFTG, they are: Mixing Neural Network Layers method which can avoid various kinds of inference attack, Practical Secure Aggregation strategy which uses cryptography to encrypt transmitted data; The Federated Dropout model which focuses on reducing the downward communication pressure, and the Deep Gradient Compression method that can substantially compress the gradient. Experimental results show that, MixNN can ensure the privacy protection without affecting the accuracy of the model; Practical Secure Aggregation saves the communication cost when dealing with large data vector size while protecting the privacy; Federated Dropout reduces communication consumption by up to 28×; DGC can compress the gradient by 600× while maintaining the same accuracy. Therefore, if these methods are used in FedFTG, its privacy and communication efficiency will be greatly improved, which will make distributed training more secure and convenient for users, and also make it easier to realize joint learning training on mobile devices.
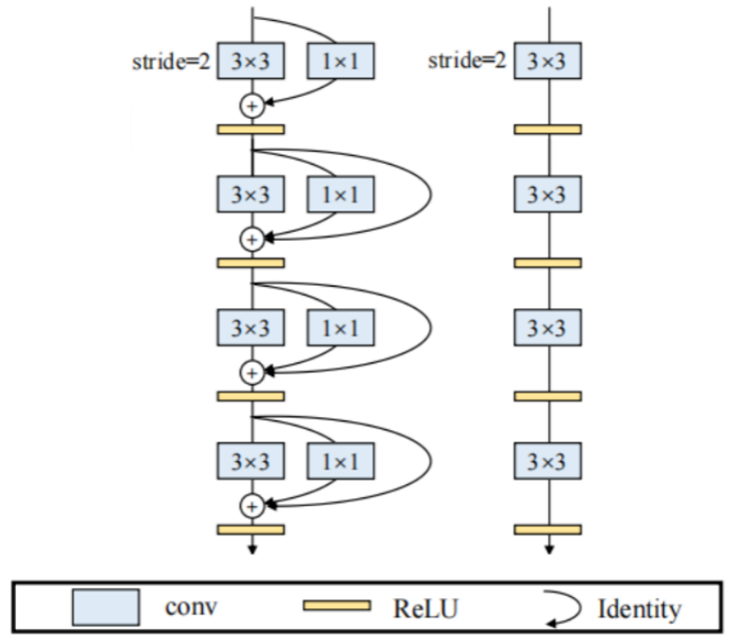
Artificial neural networks have developed rapidly in recent years and play an important role in the academic field. In this paper, the RepVGG artificial neural network model is adjusted by the learning rate algorithm, so as to realize the optimization of the model including but not limited to accuracy. The main optimization strategy is to add the warmup strategy based on the learning rate algorithm of the original model so that the model can obtain good prior information on the data early in the training process, so as to converge quickly in the later training. Through a series of tests and simulations, the RepVGG-A0 model improves the Top1 accuracy by about 2.6% to 68.56% and the Top5 accuracy by about 0.38% to 94.32% on imagesetter dataset within 25 training epochs. The precision and recall are improved to 68.43% and 68.63%, respectively.
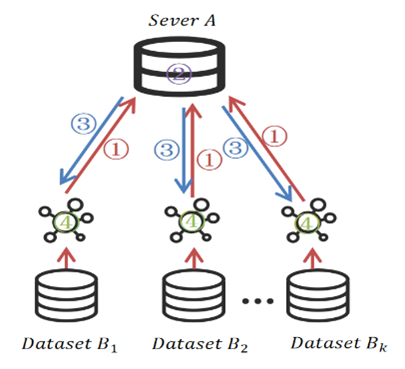
With the development of optical network, modern optical network needs better performance. Because the traditional optical transceiver technology has a delay according to the flow switching transmission configuration, the delay optical network service still adopts the original configuration transmission, so a certain degree of frequency spectrum resources waste and high blocking rate will be caused. The above situation can be improved if the transmission configuration can be deployed in advance based on the predicted traffic. Federated learning is a scheme of distributed training model, which can train the traffic prediction model in distributed way under the premise of ensuring the privacy of client data, which is very suitable for the traffic prediction of optical network terminals. This paper proposes an intelligent optical transceiver technology based on federal learning traffic prediction, applies the federal learning on the traffic prediction of optical communication network terminal, distributed training traffic prediction model, and deploy the optical transceiver early transmission configuration such as modulation format and baud rate parameters, thus to weaken the delay of optical transceiver technology, reduce the network blocking rate and improve the transmission performance of optical network.
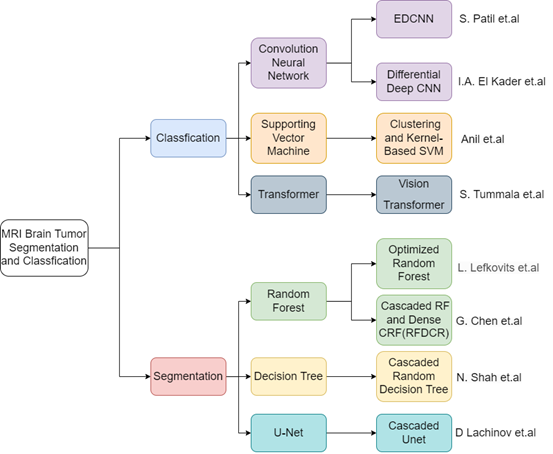
Brain tumors have a high-risk factor and are extremely harmful to the human body. With the development of science and technology in recent years, automatic segmentation has become popular in medical diagnosis because it provides higher accuracy than traditional hand segmentation. At present, more and more people start to study and improve it. Due to the non-invasive nature of MRI, MR images are often used to segment and classify brain tumors. However, limited by the inaccuracy and inoperability of manual segmentation, it is very necessary to have a complete and comprehensive automatic brain tumor segmentation and classification algorithm technology. This article discusses the benefits, drawbacks, and areas of application of several traditional algorithms as well as more modern, improved, and more advanced algorithms. Segmentation methods and classification methods can be used to classify these techniques. Convolutional neural networks (CNN), Support vector machines, and Transformers are examples of classification methods. Random forests, decision trees, and improved U-Net algorithms are examples of segmentation methods. To discuss the capability of classification and segmentation, there are three sections in the area used for segmenting brain tumors with three types, including Tumor Core, Enhance Tumor, and Whole Tumor, which could be abbreviated as TC, ET, and WT. Through the comparative analysis of these methods, useful insights for future research are provided.
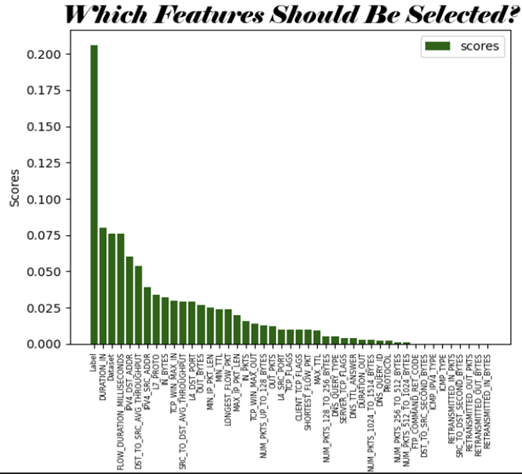
The topic of Intrusion Detection System (IDS) has become a highly debated issue in cybersecurity, generating intense discussions among experts in the field. IDS can be broadly categorized into two types: signature-based and anomaly-based. Signature-based IDS employ a collection of known network attacks to identify the precise attack the network is experiencing, while anomaly-based IDS employ machine learning models to detect anomalies present in the network traffic that could indicate a potential attack. In this study, we concentrate on anomaly-based IDS, evaluating the effectiveness of three supervised learning algorithms - Decision Tree (DT), Naive Bayes (NB), and K-Nearest Neighbor (KNN) - to determine the most suitable algorithm for each dataset based on its source. We conducted tests to evaluate each algorithm's performance and choose the best one for each dataset. Our findings show that anomaly-based IDS is highly effective in enhancing network security, providing valuable insights for organizations looking to improve their security measures.
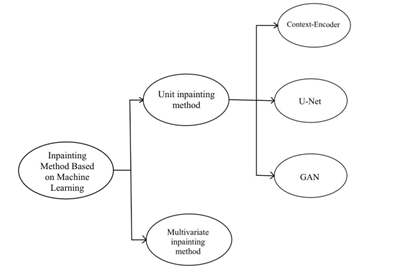
The technique of restoring sections of a picture that have been lost or damaged is known as "image inpainting." In light of recent developments in machine learning, academics have begun investigating the possibility of using deep learning methods to the process of picture inpainting. However, the current body of research does not include a comprehensive review of the many different inpainting methods that are based on machine learning, nor does it compare and contrast these methods. This article provides an overview of some of the most advanced and common machine learning based image restoration techniques that are currently available. These techniques include Multivariate inpainting technology and Unit inpainting technology, such as Context-Encoder Network, Generative Adversarial Network (GAN), and U-Net Network. We examine not just the benefits and drawbacks of each method, but also the ways in which it might be used in a variety of settings. At the conclusion of the piece, we predict that machine learning-based inpainting will continue to gain popularity and application in the years to come.
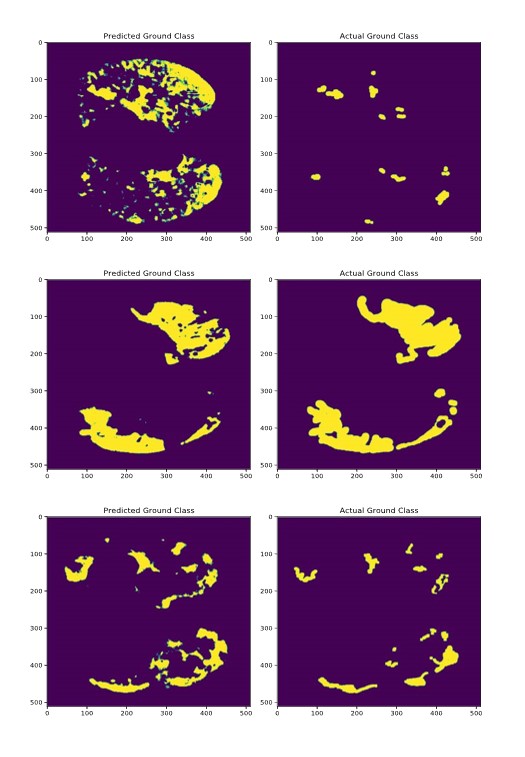
The ongoing COVID-19 pandemic has highlighted the importance of accurate and efficient medical image analysis to aid in the diagnosis and treatment of patients. In particular, the segmentation of COVID-19 medical images has become a critical task to identify regions of interest, such as the infected lung areas, and to track disease progression. Traditional image segmentation methods have been widely used in medical image analysis. However, these methods are often challenged by the complex and diverse nature of COVID-19 images, as well as the limited availability of data. In this paper, we propose a simplified version of the U-Net that eliminates redundant crop operations. This simplification reduces computational complexity and memory usage, and enables the model to learn from larger input images, resulting in better performance. We evaluate the performance of our simplified U-Net model on a public COVID-19 dataset and demonstrate that our model achieves state-of-the-art results while using fewer computational resources.
Minimum spanning tree has many applications in real life. For example, the government needs to build roads between many cities. Therefore, it is necessary to find the plan with the shortest path to save the cost. The problem is essentially generating a minimal spanning tree, and it require a suitable algorithm to find the minimum spanning tree. In this paper, the author analyzes the structure and time complexity of the Prim algorithm, the Kruskal algorithm and the Boruvka algorithm. Through this research, the author finds Prim algorithm is suitable for dense graphs. The Kruskal algorithm can generate the minimum spanning tree in sparse tree. And the Boruvka algorithm is suitable for graphs that have some special characters. Based on the above conclusions, the author gives some suggestions for urban highway network planning.