Volume 124
Published on December 2024Volume title: Proceedings of the 8th International Conference on Economic Management and Green Development
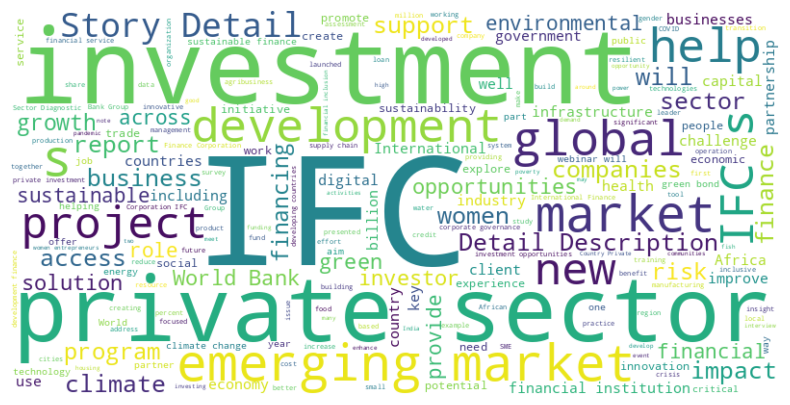
Green finance has gained global significance as governments and financial institutions emphasize sustainable investment. Understanding the sentiment of green finance reports can provide valuable insights into public perception, investor sentiment, and policy reception. This study uses three different models — FinBERT, GPT-3.5 Turbo, and GPT-4o -- to perform sentiment analysis on over 1000 reports obtained from the International Finance Corporation (IFC) website. To assess the accuracy of the models, this paper manually labeled the sentiment of the reports into three categories: Positive, Negative, and Neutral. We compared the models’ outputs using standard metrics such as F1-score, Accuracy, Precision, and Recall. The findings indicate that GPT-3.5 Turbo outperforms the other models in terms of accuracy. GPT-4o shows superior performance compared to Finbert which trained on financial texts in extracting sentiment from general text. Even though FinBERT and GPT-4 have stronger financial text processing capabilities, GPT-3.5 Turbo can often capture the true intent and sentiment of the text more quickly and clearly, especially when trained on a relatively small text corpus. Its generalization and speed make it efficient for less complex financial tasks.
In this paper, the Nasdaq 100 Index (ETF) is selected as a representative of the technology sector, and the dominant factors affecting the performance of stock returns in the technology market are explored through data processing and regressions of its monthly returns from 2014 to 2023.In this study, the Nasdaq 100 Index (ETF) is used as a proxy for the technology sector. The study investigates the dominant factors affecting the performance of the technology market stock returns. This study adds 10 extended factors to the Fama-French three-factor model,with the aim of finding the variables which strongly impact on NDX returns. Each factor was tested and screened through multiple time series regression, multiple covariance analysis, and stepwise regression. Finally, the Fama-French two-factor extended model with the largest R-squared value and the strongest explanatory power for NDX returns was constructed. Its independent variables include market returns and premiums, SMB, HML, interest rate changes, and industrial production index changes. Compared to the original Fama-French three-factor model (R-squared 0.871), the reconstructed Fama two-factor extended model has a better fitting which has an R-squared of 0.885, which illustrates the effect of the newly added factors on the technology market stock portfolio and provides an empirical evidence on that the new model is an improvement for the technology market stock portfolio.
This paper aims to address the current development status and problems of new energy vehicle companies, taking Tesla as an example. The research question is to understand the challenges of low brand awareness and inadequate crisis public relations handling faced by Tesla in emerging markets and second- and third-tier cities. This is important to the academic field because it provides insights into the development and challenges of the new energy vehicle industry. This study adopts a case analysis method, taking Tesla as an example to study the current development status of new energy companies and some related problems, and proposes some countermeasures and suggestions for the problems found. The results show that in some emerging markets and smaller cities, Tesla has limited brand awareness due to less promotion compared with traditional car brands and consumers' trust in traditional brands. In terms of crisis public relations, Tesla's response is sometimes not timely or transparent enough. The solutions proposed include holding more events and developing personalized marketing strategies in these areas, as well as establishing a rapid response mechanism and strengthening communication with consumers and regulators. The impact of this work is to provide Tesla with practical suggestions to improve its brand image and competitiveness, and also provide valuable lessons for other new energy vehicle companies to deal with similar problems.
Research about the Fama-French (F-F) model has long attracted significant academic attention. This study explores the application and extension of the F-F model within the healthcare sector. Using the Vanguard Health Care ETF (VHT) as a representative of the healthcare sector, this research examines its monthly returns over the period from 2014 to 2024. A comparison between the Fama-French three-factor (FF3) and five-factor models (FF5) reveals that the FF5 model is more suitable for the healthcare industry, the impact on ETF returns increased by 3.82%. To identify a model better suited for the healthcare sector, this study extends the FF5 model by incorporating an additional 11 factors. Through stepwise regression and significance testing, the final model with the highest adjusted R² value and strongest explanatory power for ETF returns was constructed. The independent variables in this Extension model include market excess returns (Rm-Rf), HML, CMA, the momentum factor (MOM), inflation rate (INFR), employment change rate (EMP), and the PPI for medical laboratories (PPILAB). Compared to the original FF5 model, which had an adj R² of 0.6980, the Adj R² of the Extension model increased to 0.7359, demonstrating a better fit for the healthcare industry. As a result, with the help of this study, investors will be better equipped to use the F-F Model and make informed investments in the healthcare sector.
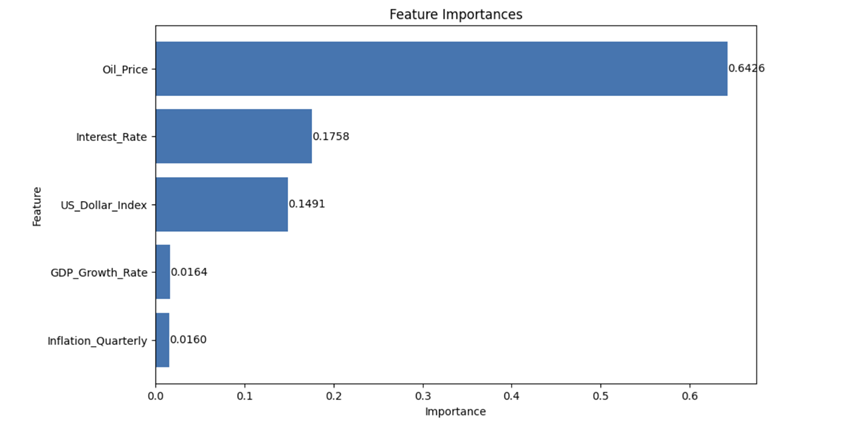
Nowadays, investing in gold as a hedge against macroeconomic factors risk has been brought into the spotlight. It is essential for investors to predict gold prices before making their hedging decisions. After a thorough study of past precursors, the machine learning method, especially the gradient boosting technique, has been proven to be a powerful method for predicting gold prices. This study tests macroeconomic factors as well as oil prices and uses them as independent variables to predict gold prices. By using advanced machine learning technique: gradient boosting, this study achieves finding the best four factors model. The research shows that oil prices, the U.S. dollar index, interest rate, and GDP growth are significant factors in predicting gold prices. Among all the significant factors, oil price is the most important one. The explicit reasons of this result is because that transportation cost and operation cost of gold mining is largely influenced by oil price. This study lays the foundations for investors to make insightful decisions.
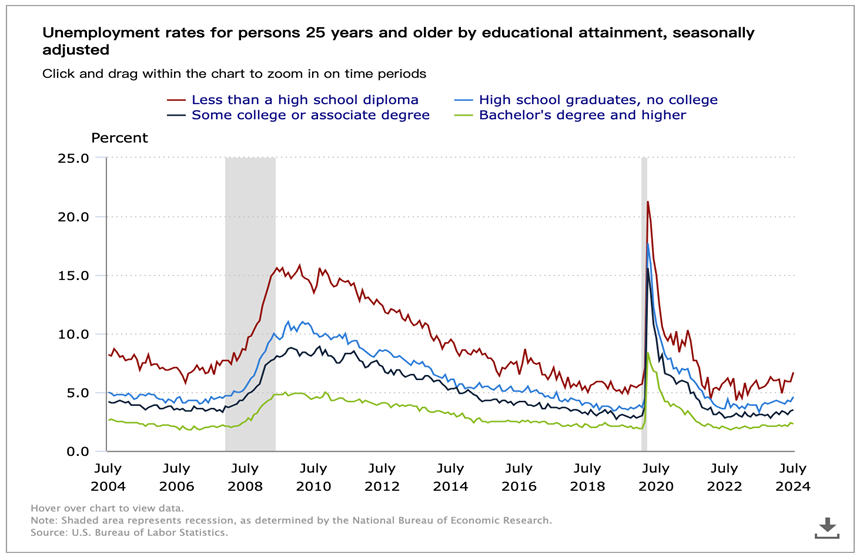
This study investigates U.S. unemployment rates among individuals aged 25 and older, segmented by four distinct levels of educational attainment. Understanding the interplay between education and employment is crucial for effective policy-making and long-term economic planning. To capture these dynamics, a regression model is utilized to derive equations representing the patterns observed in four separate time series, each corresponding to a specific educational group. In addition to this, logarithmic difference analysis is applied to compare these time series, resulting in the creation of three new plots that help further elucidate the relationships between educational attainment and unemployment rates. The analysis of these new plots reveals a narrowing disparity in unemployment rates across various educational levels, suggesting a trend toward convergence. Over time, this indicates a potential reduction in gaps based on educational background. The findings provide valuable insights into the evolving U.S. labor market, highlighting the influence of education on employment outcomes. These insights offer important implications for future policies aimed at reducing unemployment disparities and fostering equitable job opportunities across different educational levels.
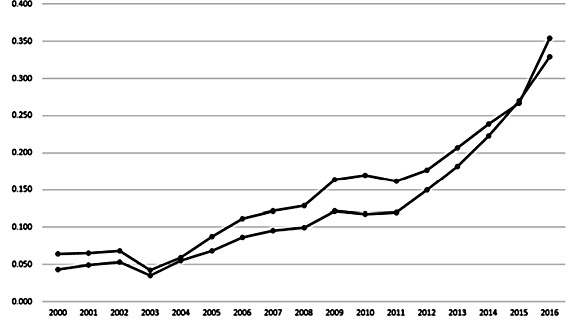
The explosive fire of the black myth Wukong is not only its technical production, but also its stunning location. This paper is going to explore six of these tourist venues and Datong, a city full of tourism consumption potential. The relationship between tourism and urban development will be explored through practical cases. This paper will refer to national policies and discuss related topics of tourism and urban transformation. And put forward ways to expand the international market. Datong has the most locations for the black myth of Wukong with six. At the same time, in the process of the transformation of Datong from an energy-based economic city, tourism needs to publicize Datong in the process of playing its advantages in promoting economic growth. This paper not only analyzes the benefits in other similar Internet celebrity tourism cities, but also analyzes the new opportunities in the continuous improvement of the transportation network.
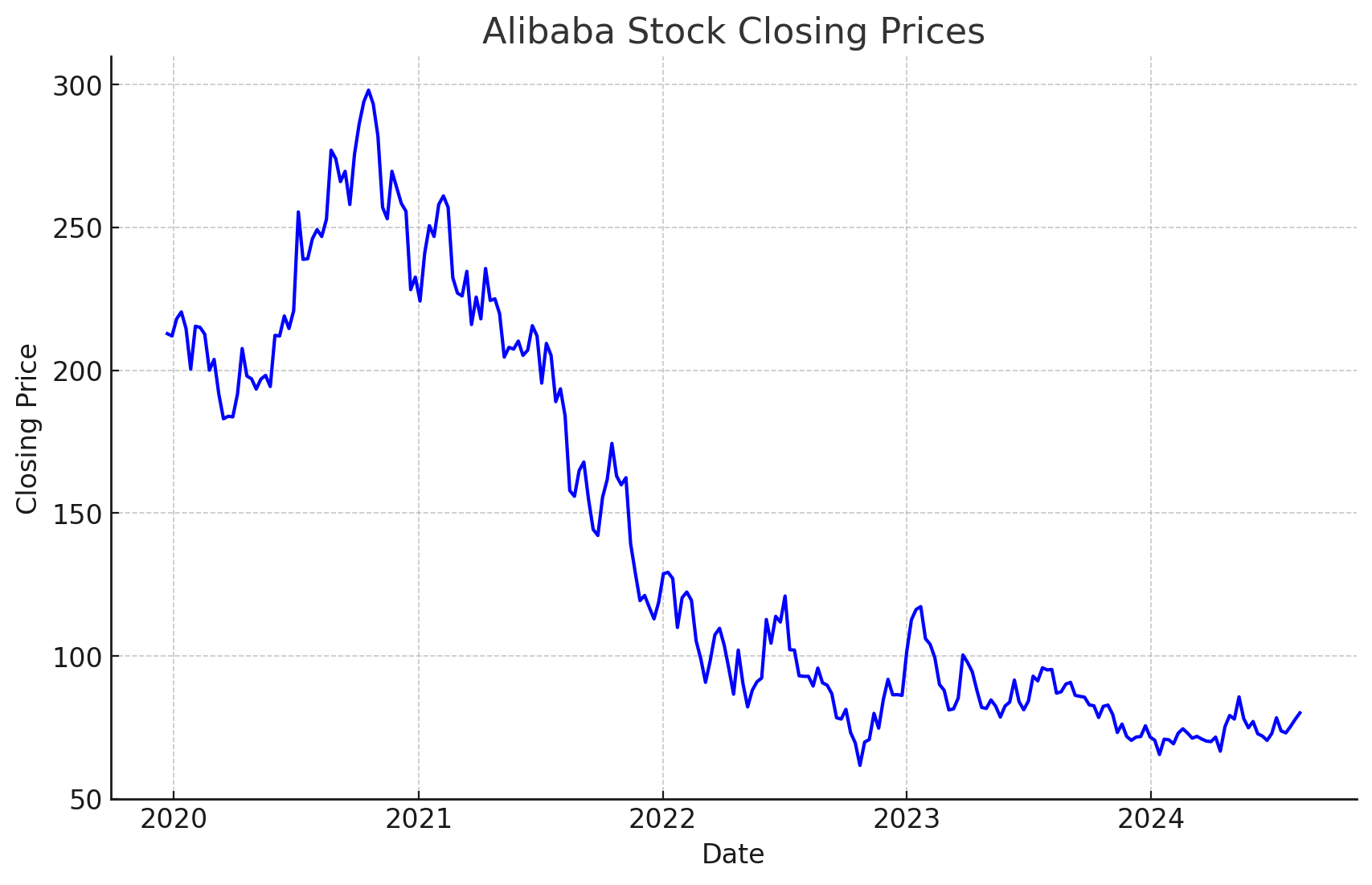
This study investigates how the COVID‐19 pandemic affected the stock value of Alibaba by examining fluctuations in its market performance to and following the outbreak using the ARIMA models. The research utilizes Alibabas closing stock prices from December 2019 to August 2024 to cover both pre-pandemic and post pandemic situations comprehensively. By employing the ARIMA model for analyzing stock performance patterns and projecting market trends the study aims to shed light on the impact of the pandemic, on Alibabas stock value over time. The research results show that Alibabas stock price went through ups and downs along with growth over time; However, the COVID-19 pandemic brought about a lot of unpredictability into the mix, highlighting the necessity for improved forecasting methods that can adapt to sudden market changes effectively. These findings hint at the idea that while the ARIMA model is good at identifying trends in data patterns; it should be combined with techniques to be more prepared for unexpected situations. The study emphasizes how having a diverse investment portfolio is crucial, during times and stresses the importance of keeping up with regulations and making strategic investment choices wisely.
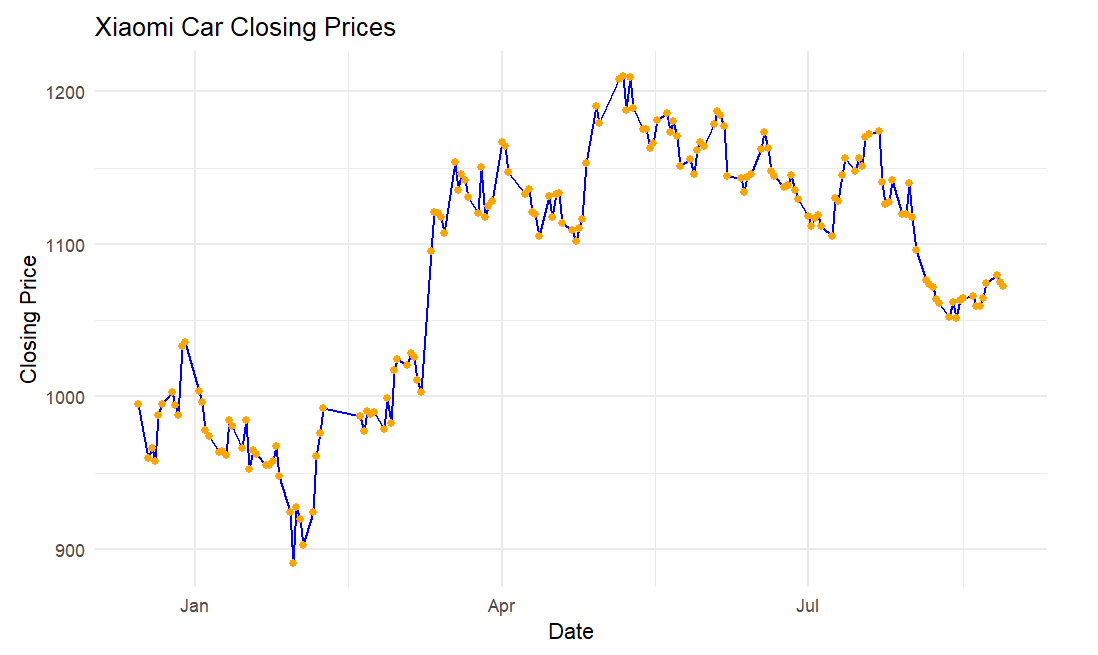
Since Xiaomi Corporation entered the market for electric vehicles, the volatility of its stock price has attracted a lot of attention from investors. This study's objective is to foresee the stock price of Xiaomi Auto by constructing GARCH and ARIMA models in R. By conducting a stationarity test and differencing the previous stock price information of Xiaomi Auto, and then the stock price for the upcoming 30 days is predicted using an ARIMA (0,1,0) model. The findings demonstrate that while the ARIMA model predicts a short-term phase of relatively stable stock price, the widening prediction intervals suggest increasing uncertainty. The construction of a GARCH (1,1) model aims to more accurately represent the fluctuations in the stock price. Predictions from the GARCH model point to higher price volatility in coming 30 days. Based on these model outcomes, this paper proposes short-term and medium- to long-term investment strategies for investors and discusses the limitations of the models as well as potential improvement for future research. This research improves the understanding of stock price trends and volatility in the electric vehicle market. It provides practical insights for better investment decisions. The study also advances financial forecasting methods, making them more applicable to real-world market behavior.
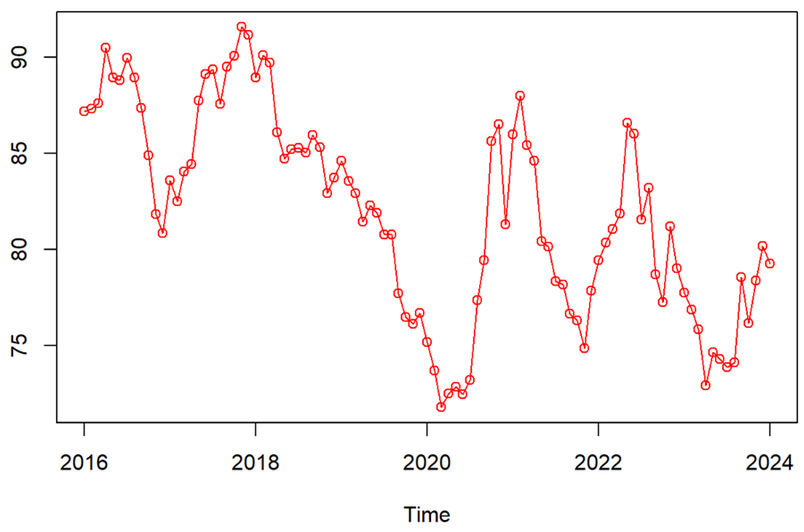
As a key indicator of the global financial market, the relationship between the US 10-year treasury bond and the US dollar index has attracted extensive attention from the academic community. To explore the correlation and future trends between the two. This article collects relevant data, analyzes the relationship between ARIMA and VAR models, and makes future predictions. The research results show that in the future, the dollar index will remain around 80 points and treasury bond will remain around 4 points. But the correlation between them is weak. At the same time, because the trend of the 10-year treasury bonds of the United States is affected by many factors, more factors need to be considered for their future trend. This study provides empirical evidence for the development of the US economy and lays the foundation for future investors' asset allocation, which has important practical significance for the development of financial markets.