Volume 118
Published on December 2024Volume title: Proceedings of the 3rd International Conference on Financial Technology and Business Analysis
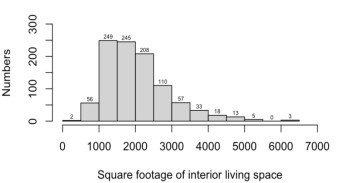
Variations in housing prices exert a profound impact on economic policies and personal financial decisions. This study aims to delve into the factors that affect housing prices and construct a predictive model using machine learning techniques. Machine learning enables computers to acquire knowledge from data and make predictions or decisions. This study forcasts house prices by analyzing the characteristics of data from Seattle, Washington using machine learning multiple linear regression, polynomial regression, and K-nearest neighbors regression (KNN). The findings of this investigation demonstrate that the polynomial regression model is the most accurate in predicting housing prices and can capture nonlinear relationships in the data more effectively than multiple linear regression and K-nearest neighbors regression. The main factors affecting housing prices are interior living space and the construction and design of buildings. These insights hold potential for enhancing government policies, facilitating effective land use decision-making by planners, and enabling investors to make more informed choices.
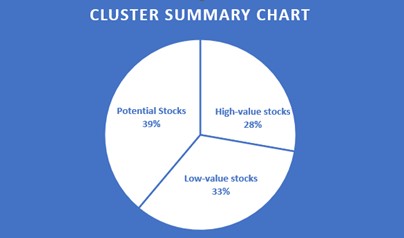
This paper selects the financial statement data of 18 listed companies in the field of education and selects 9 financial indicators from the four aspects of solvency, profitability, development ability and operation ability that can reflect the comprehensive strength of listed companies. Finally, the stocks of 18 listed companies were divided into three categories: high-value stocks, potential stocks and low-value stocks according to the comprehensive strength of the companies. The results show that: (1) From the comparison between the rankings of the selected 18 stocks combined with the traditional methods by combining principal component analysis and entropy weight method, it can be seen that for the comprehensive multi-index evaluation analysis in the past five years, the ranking agreement between the two methods can reach 80% or more. Due to the unstable fluctuations of the stock market, the consistency between the analysis results and investment recommendations obtained in this paper and the actual investment situation of the stock market can also exceed 60%. (2) From the results of cluster analysis, Chuanzhi Education, Entrepreneurship Dark Horse, Guoxin Culture, China Hi-Tech and Action Education are all high-value stocks in the education category, while Meijim, Qinshang Co., Ltd., and Kaiwen Education are potential education stocks.
Innovation is also playing an increasingly important role in the process of transitioning to high-quality economic development. Therefore, it is imperative that enterprises, as micro-entrepreneurs improving the quality of economic growth, pay attention to the enhancement of their R&D capabilities. So how can positive ESG performance by firms alleviate financing constraints and thus promote R&D investment? Therefore, this paper investigates the impact of ESG performance and financing constraints on R&D investment, which is of great significance in promoting enterprises to pay attention to ESG management, alleviating financing constraints, and promoting the enhancement of R&D capability. The results of this study show that corporate ESG performance has a positive effect on R&D investment; corporate ESG performance has a negative effect on financing constraints; financing constraints play a partly intermediary role in the process of corporate ESG performance affecting R&D investment.
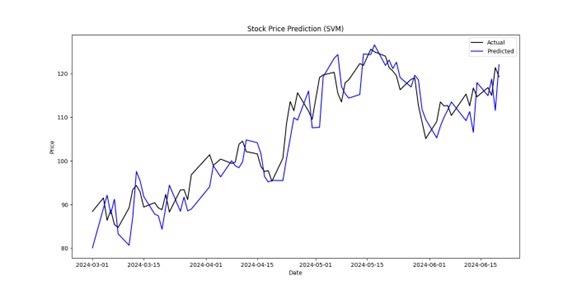
Stock price prediction has always been an important topic in financial research. Accurate price prediction can not only help investors make informed investment decisions but also enhance market stability and reduce systemic risk. In recent years, with advancements in computing technology and data science, machine learning methods have been increasingly applied in the financial field. Compared to traditional methods, machine learning methods can better handle high-dimensional, nonlinear, and large datasets, thus demonstrating higher prediction accuracy and applicability in stock price prediction.This paper reviews relevant literature and selects five models for empirical research: Support Vector Machine (SVM), Long Short-Term Memory network (LSTM), LightGBM, a combination of LSTM and LightGBM, and Convolutional Neural Network (CNN). The effectiveness of these models in predicting the stock price of Meituan-W (3690) was analyzed and compared in detail. The experimental results show that the LightGBM model performs best in terms of Mean Squared Error (MSE), Mean Absolute Error (MAE), and Root Mean Squared Error (RMSE), proving its significant advantages in handling large-scale, high-dimensional, and nonlinear data. By comparing the prediction results of different models, this paper explores the strengths and weaknesses of each model and their feasibility and effectiveness in practical applications. Machine learning methods have significant potential in stock price prediction, model selection needs to comprehensively consider data characteristics, computational resources, and practical application scenarios.
In light of the nation's increasingly pressing aging problem, achieving sustainable development of China's pension insurance fund is a key objective of the country's present social security system reform. The first subject addressed in this paper is the connection between aging and the sustainability of the pension insurance fund. An econometric model can be used to illustrate how the aging population will reduce the basic pension insurance fund's balance rate. This will have an additional effect on the fund's income and expenditure balance, causing a payment gap and hindering the fund's capacity to develop sustainably. As such, a review of the national pension insurance program is carried out. From the perspectives of policy concerns and improvement ideas, we argue for the soundness and areas for development in the national pooling policy of pension insurance funds. The sustainability of pension insurance funds is the ultimate goal, and it also helps with the difficulties brought on by shifting demographics and the pressure on pension insurance fund contributions.
Urban digital transformation has become important to promote social progress and economic growth. At present, rural development is seriously lagging, and it is of great significance to explore the impact of urban digital transformation on rural revitalization to promote the coordinated development of urban and rural areas and rural revitalization. This study empirically investigates the impact of urban digital transformation on rural revitalization based on municipal panel data from 2009-2021. The study shows that urban digital transformation significantly drives rural revitalization, and e-commerce plays a mediating role in it. The improvement of green economy efficiency, industrial agglomeration, and human capital level will enhance the impact of urban digital transformation on rural revitalization. From the results of heterogeneity analysis, the effect is more obvious in the eastern region. This study also proposes policy suggestions from three perspectives: to promote urban digital transformation, to focus on key elements such as e-commerce, and to develop regional synergies, which provide a reference for promoting rural revitalization in the context of urban digital transformation.
The emergence of the asset-light operational model provides a new direction for corporate transformation, enabling companies to improve market adaptability and overall operational flexibility by controlling the input of fixed and other heavy assets. This model has garnered considerable attention from enterprises; however, research on the impact and transmission mechanisms of this model on corporate development remains insufficient. In light of this, this paper uses A-share listed companies in China from 2012 to 2022 as the sample and empirically examines the impact of the asset-light operational model on corporate performance, while identifying the transmission mechanism through the lens of supply chain resilience. The study finds a significant positive correlation between the asset-light model and corporate performance. The mechanism test shows that the asset-light model promotes corporate performance growth by enhancing supply chain resilience, establishing a deduction pathway where the asset-light model drives corporate performance improvement. This paper enriches the research on the impact mechanisms of the asset-light model on corporate performance from a new perspective and provides a fresh direction for enterprises to improve performance through transformation in asset operation models.
This paper takes financial sharing-corporate governance level-firm performance as the research framework, based on the data of A-share listed companies from 2005 to 2023.It applies the double-difference bivariate fixed model and mediation effect model to explore the relationship between financial sharing, corporate governance level and firm performance.The paper further analyses the heterogeneity of the nature of equity. The results of the study show that the establishment of financial sharing centre by the company significantly enhances the performance of the company. The effect is more significant in non-state-owned firms compared to state-owned firms. Corporate governance plays a partial mediating role in it.
In the past, scholars mainly studied the relationship between corporate financialization and digital transformation of the entire economy, without specifically analyzing individual industries. At present, Chinese construction enterprises have encountered development bottlenecks and further research is needed. Against the backdrop of the significant trend of financialization in Chinese enterprises and the new strategic deployment proposed by the Chinese government in 2023, the task of digital transformation for construction enterprises is urgent. This article chooses to use panel data of Chinese construction industry listed companies from 2012 to 2022 for empirical testing to investigate the relationship between financialization and digital transformation of construction enterprises. Research has found that: (1) Corporate financialization can make positive effect on digital transformation activities of construction enterprises; (2) Financialization of construction enterprises can alleviate financing constraints; (3) Financialization of construction enterprises weakens the inhibitory effect of financing constraints on enterprise digitization.
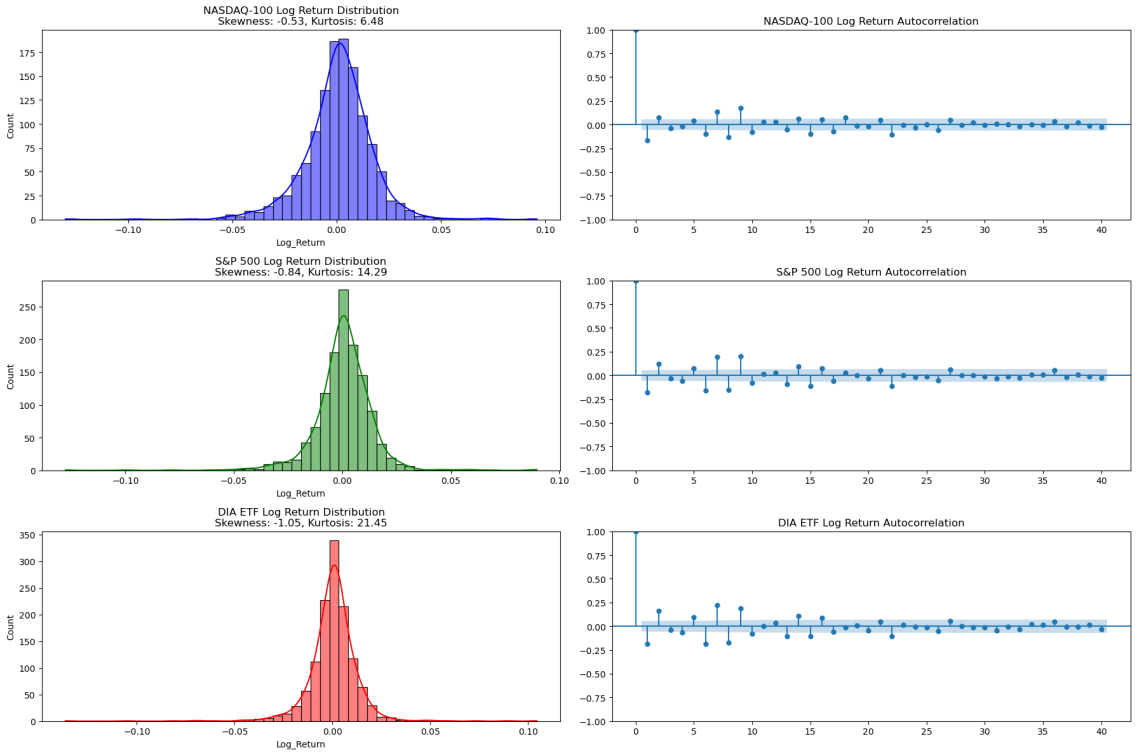
The main objective of this study is to increase the accuracy of option pricing of the Black-Scholes model by integrating GARCH models and analyzing the effectiveness of this method across different industries. This study focuses on the three major U.S. stock indices needed to achieve this goal and derive the results. By applying the GARCH model to options data from these indices, this study shows that the GARCH model significantly enhances the pricing accuracy for SPX and DJIA options while not yielding similar improvements for NDX options. This discrepancy highlights the GARCH model's effectiveness in more stable, less volatile industries like industrials and manufacturing but its reduced utility in the technology sector, where stock price fluctuations are more unpredictable. Finally, This study recommends that investors use the GARCH model in industries such as manufacturing and suggests that future research adopt more accurate and advanced models to enhance option pricing in highly volatile sectors, providing investors with better tools and methodologies.