Volume 82
Published on November 2024Volume title: Proceedings of the 2nd International Conference on Machine Learning and Automation
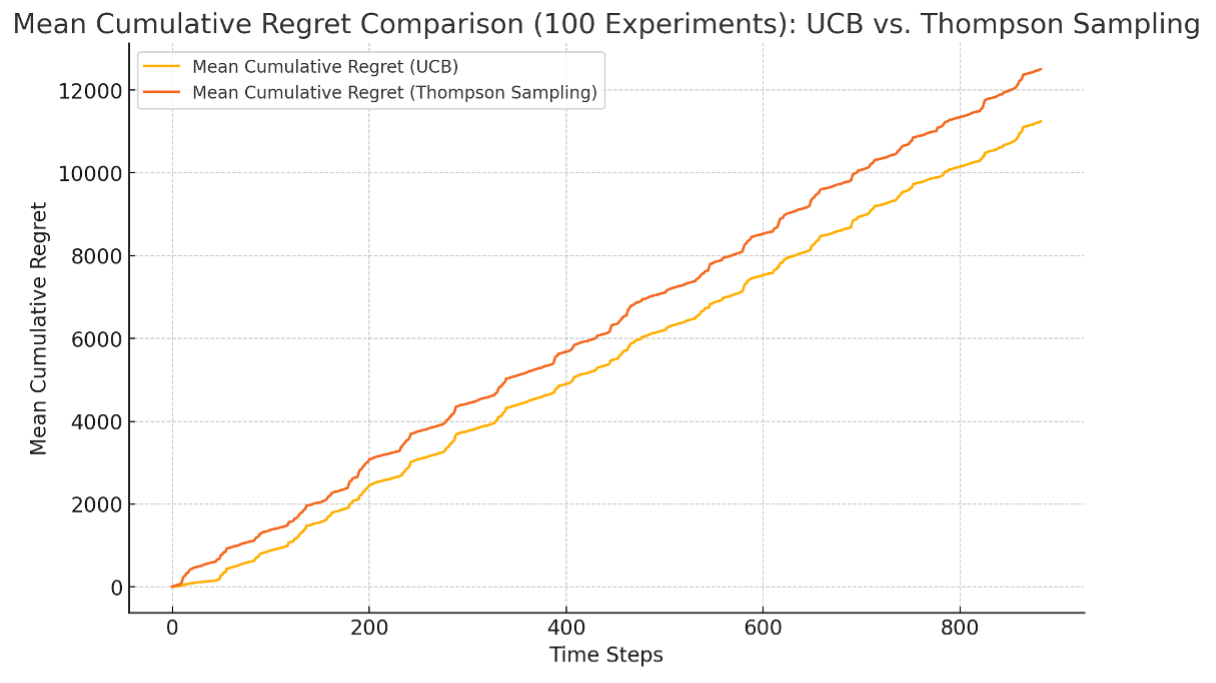
This study explores the application of multi-armed bandit (MAB) algorithms in dynamic pricing of crops, with a focus on evaluating the adaptive upper confidence bound (asUCB) and Thompson Sampling (TS) algorithms. Through simulation experiments on historical data, the study analyzed the performance of these algorithms in fitting actual market price trends and responding to future price fluctuations. The results indicate that the asUCB algorithm performed particularly well in both the training dataset and simulation tests, demonstrating low mean squared error (MSE) and minimal cumulative regret, reflecting its rapid convergence and stable pricing capabilities. In contrast, although the TS algorithm initially performed slightly less effectively, it demonstrated unique advantages in dealing with market volatility due to its strong adaptability. This study demonstrates the potential application of MAB algorithms in dynamic pricing, providing valuable insights for pricing strategies in the agricultural product market.
The Electric Vehicle market is growing fast, creating new challenges for sales predictions and market dynamics. Traditional forecasting methods like linear regression and expert judgment struggle with the market's non-linear and complex nature. To improve accuracy, new models such as machine learning and Adaptive Optimized Grey Models are being applied to predict EV sales, which is discussed in this review paper. These models dynamically adjust parameters and account for external factors, offering better precision. AOGMs, in particular, utilize data preprocessing and buffering to minimize external disturbances and enhance prediction smoothness. Techniques like the Particle Swarm Optimization help these models more effectively capture the EV sales trends. Additionally, adaptive grey models are not only essential for forecasting sales but also for identifying market risks like overcapacity or supply shortages. These advanced methods provide a more robust framework for understanding and anticipating changes in the rapidly evolving the EV market.
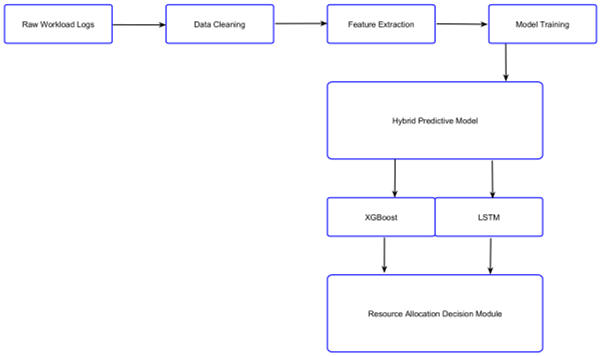
This paper proposes an innovative AI-driven approach for efficient resource allocation in cloud computing environments using predictive analytics. The study addresses the critical challenge of optimizing resource utilization while maintaining high quality of service in dynamic cloud infrastructures. A hybrid predictive model combining XGBoost and LSTM networks is developed to forecast workload patterns across various time horizons. The model leverages historical data from a large-scale cloud environment, encompassing 1000 servers and over 52 million data points. A dynamic resource scaling algorithm is introduced, which integrates the predictive model outputs with real-time system state information to make proactive allocation decisions. The proposed framework incorporates advanced techniques such as workload consolidation, resource oversubscription, and elastic resource pools to maximize utilization efficiency. Experimental results demonstrate significant improvements in key performance indicators, including increasing resource utilization from 65% to 83%, reducing SLA violation rates from 2.5% to 0.8%, and enhancing energy efficiency, with PUE improving from 1.4 to 1.18. Comparative analysis shows that the proposed model outperforms existing prediction accuracy and resource allocation efficiency methods. The study contributes to the field by presenting a comprehensive, AI-driven solution that addresses the complexities of modern cloud environments and paves the way for more intelligent and autonomous cloud resource management systems.
In the rapidly advancing field of AI and ML, this paper explores their pivotal role in transforming cybersecurity. Highlighting the integration of sophisticated techniques like deep learning for intrusion detection and reinforcement learning for adaptive threat modeling, it emphasizes the shift towards AI-driven cybersecurity solutions. The study meticulously analyzes supervised and unsupervised learning's impact on threat detection accuracy and the dynamic capabilities of neural networks in real-time threat identification. It reveals how these methodologies enhance digital defenses against complex cyber threats, underscoring the theoretical underpinnings and practical applications of AI and ML in cybersecurity. The paper also discusses the challenges and future directions, contributing significant insights into the evolving landscape of cybersecurity technologies. This comprehensive research background sets the stage for understanding the unique contributions and potential of AI and ML in strengthening cybersecurity measures.
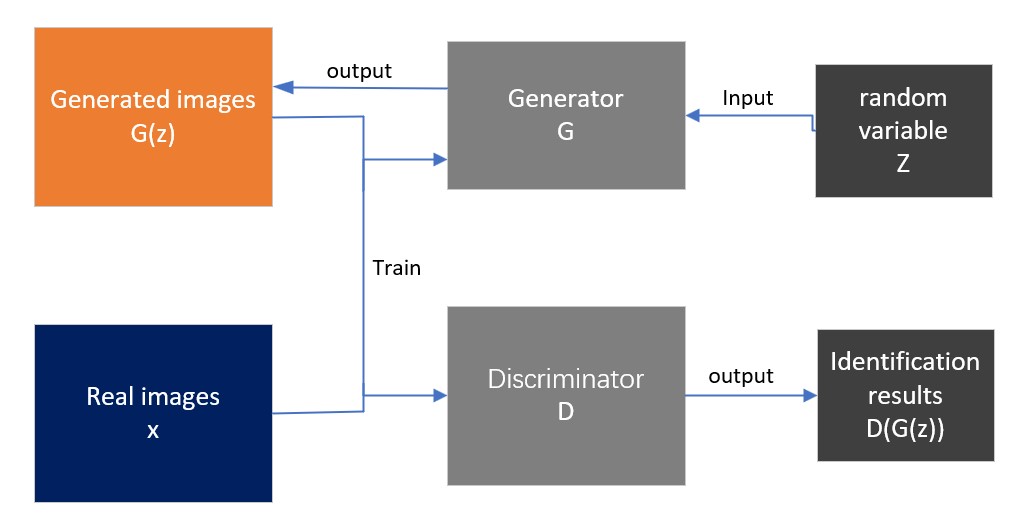
With the rapid development of machine learning, the field of image style transfer has received widespread attention, and scientists have gradually realized that this is an important field in computer vision. Image style transfer refers to the conversion of an image from one visual style to another and preserving the content. The technology of changing image style has a wide range of applications in various fields from art to virtual reality. Although current learning models have achieved some results in the study of image style transfer, they still face challenges in improving resolution and complex style transfer. This paper mainly introduces the background and characteristics of existing learning models that can achieve image style transfer. It introduces the principles, characteristics and limitations of GANs. In the basis of introduction, this paper also analyses the differences and different characteristics of GANs and summarizes the advantages and disadvantages of GANs. At the same time, this paper predicts future research directions, continuing to optimize these models, reducing training costs, and exploring hybrid methods to further advance image style transfer technology. These developments not only strengthen the theoretical research of image style transfer and GANs, but also have broad application potential in fields such as art, design and medical imaging.
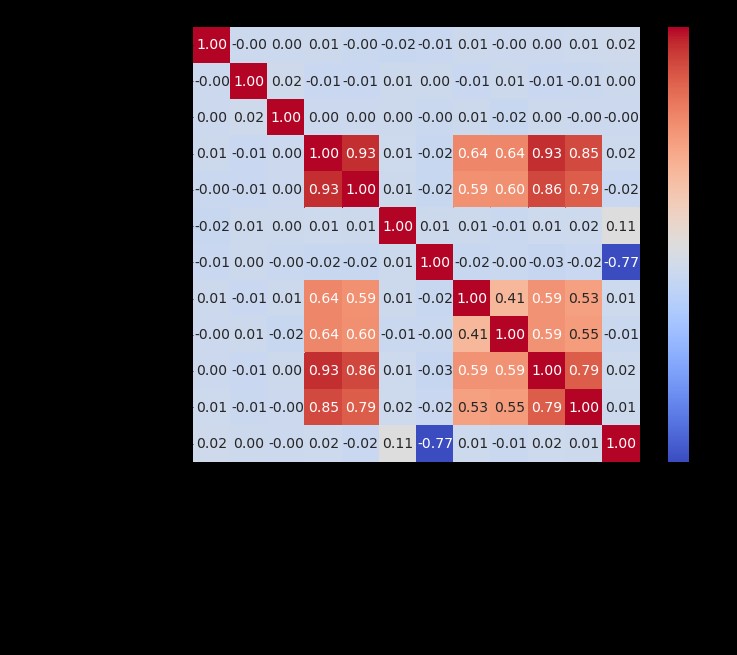
This paper discusses an improved XGBoost model based on four-vector optimization algorithm to improve the accuracy of loan approval prediction. Through the analysis of correlation heat maps, we found that there were significant positive and negative correlations among some variables, which laid the foundation for subsequent machine learning analysis. Based on this, we compare the traditional machine learning algorithm with the improved model to evaluate its performance in loan approval forecasting. In the confusion matrix analysis of the training set, the improved XGBoost model demonstrated excellent performance, with all loan approval predictions being correct with 100% accuracy. However, the performance in the test set was slightly different, with 1,182 projects receiving correct loan approval predictions and 99 projects forecasting errors. Of these, 26 projects that should have been predicted to be "unapproved" were incorrectly labeled as "approved," while 73 projects that should have been predicted to be "approved" were incorrectly labeled as "unapproved." These results suggest that we still need to pay attention to the misjudgment of the model in practical application. By synthesizing all model evaluation indicators, we found that the improved XGBoost model based on the four-vector optimization algorithm has a higher accuracy in loan approval prediction than the traditional XGBoost model, with an increase of 1.5%. In addition, the other evaluation indicators also show a trend of significantly better than the traditional model. This study shows that the four-vector optimization algorithm can effectively improve the application effect of XGBoost model in the field of loan approval, and provide more accurate data support and decision-making basis for the financial industry. In the future, we will continue to explore the potential and application prospects of this algorithm in other fields.
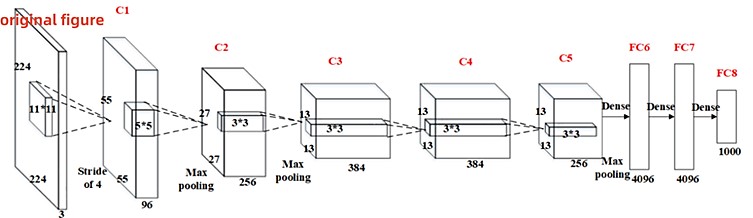
Facial recognition technology allows users to achieve efficient and accurate identity verification through facial features alone, without the need to physically touch the device. Face recognition technology can be applied in a variety of scenarios such as unlocking mobile devices, online payment, attendance management and public identity verification. Nonetheless, face recognition algorithms still have some limitations in the face of massive data and complex application scenarios. Therefore, there is an urgent need for more advanced technical means to overcome the shortcomings of traditional algorithms such as low recognition rate and poor adaptability. As deep learning methods have become more popular, especially the emergence of Convolutional Neural Networks (CNN) and Transformer Networks, face recognition has been revolutionized. This paper firstly describes the principles of convolutional neural network model and Transformer model. Secondly, the applications of CNNs and Transformer networks at different stages of development in the domain of face recognition are reviewed. Then, this paper combs through the deep learning models applied to face recognition methods and evaluates their characteristics, innovativeness, usefulness and portability respectively. Finally, this paper summarizes the challenges faced by deep learning in the domain of face recognition as well as the trend of face recognition technology development in the future, aiming to point out the research focus research direction for the subsequent research.
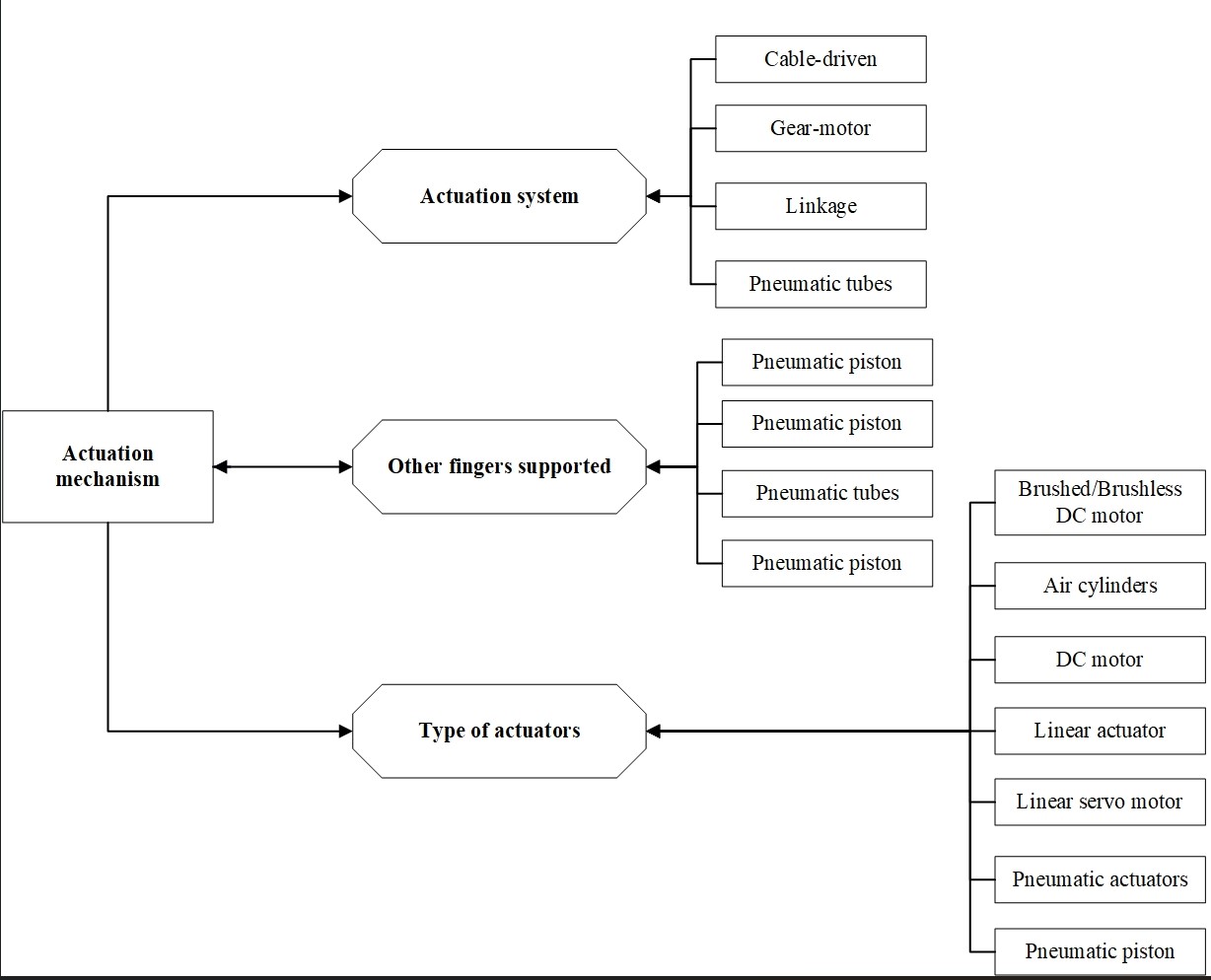
Stroke patients experience impaired hand function which significantly impacts their daily activities. Robot-assisted training is essential for restoring motor function, with hand exoskeletons serving as assistive devices to support, amplify, replace, or counteract body movements. Designing an exoskeleton that accurately mimics the natural movement of the thumb and provides adequate support is a key challenge in rehabilitation. This paper designs a retrieval model for hand rehabilitation exoskeletons from 2009 to 2023, analyzing the hand anatomy and thumb motion range. It discusses various driving mechanisms for hand exoskeletons, including cable, linkage, pneumatic, gear motor, and hybrid systems. While progress has been made in experimental stages, more research is needed for clinical applications. A universal hand exoskeleton actuation system that meets all patient preferences does not exist. Ongoing research on hybrid drive systems aims to enhance hand exoskeletons for better rehabilitation and daily use for patients with hand dysfunction.
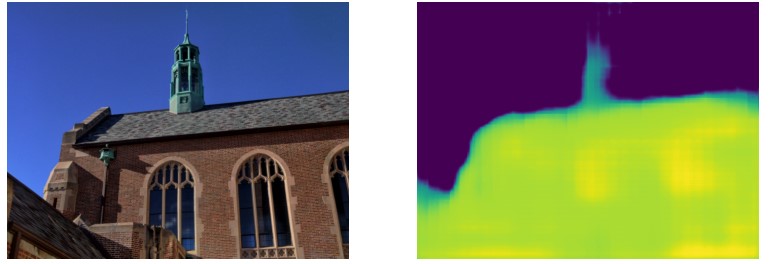
Depth estimation is a key task in computer vision, critical for applications such as autonomous navigation and augmented reality. This paper introduces a novel hybrid neural network that combines ResNet and UNet architectures with a SoftHebbLayer, inspired by Hebbian learning principles, to improve depth estimation from RGB images. The ResNet backbone extracts robust hierarchical features, while the UNet decoder reconstructs fine-grained depth maps. The SoftHebbLayer dynamically adjusts feature connections based on co-activation, enhancing the model’s adaptability to diverse environments. This approach addresses common challenges in depth estimation, including poor generalization and computational inefficiency. We evaluated the model on the DIODE dataset, achieving strong results in Mean Squared Error (MSE), which is 0.0800 and Root Mean Squared Error (RMSE), which is 0.2805, demonstrating improved accuracy in both indoor and outdoor scenes. While the model excels in precision, further refinement is needed to reduce computational overhead and improve performance in challenging environments. This research paves the way for more efficient, adaptable depth estimation models, with potential applications in mobile robotics and real-time edge computing systems.
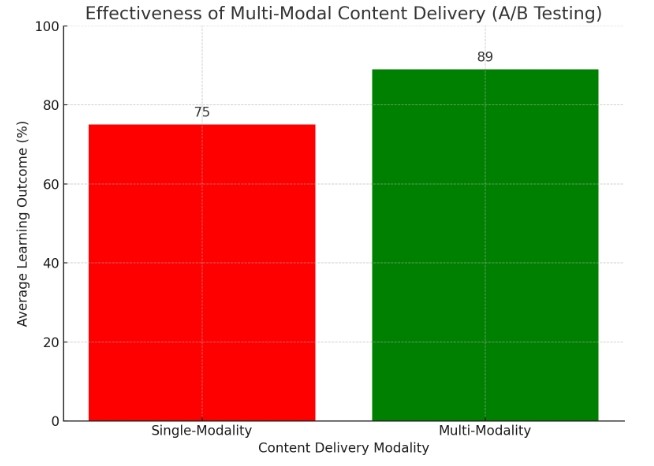
The integration of artificial intelligence (AI) into education has opened doors to personalized learning experiences. This paper introduces the Adaptive Cognitive Enhancement Model (ACEM), a cutting-edge AI-driven framework designed to personalize cognitive development for students. Leveraging advanced machine learning algorithms and quantitative analysis, ACEM adapts educational content and learning strategies to individual cognitive needs. The model encompasses five key components: cognitive profiling, adaptive learning paths, intelligent feedback, motivational strategies, and longitudinal tracking. Through quantitative analysis and mathematical modeling, the paper demonstrates how ACEM significantly enhances learning outcomes compared to traditional education models. The discussion section provides a detailed exploration of each model component, its architecture, and its role in optimizing personalized cognitive development. Furthermore, challenges such as data privacy, scalability, and model interpretability are examined, alongside potential solutions. The conclusion underscores the transformative potential of ACEM in revolutionizing education.