Volume 144
Published on April 2025Volume title: Proceedings of the 3rd International Conference on Functional Materials and Civil Engineering
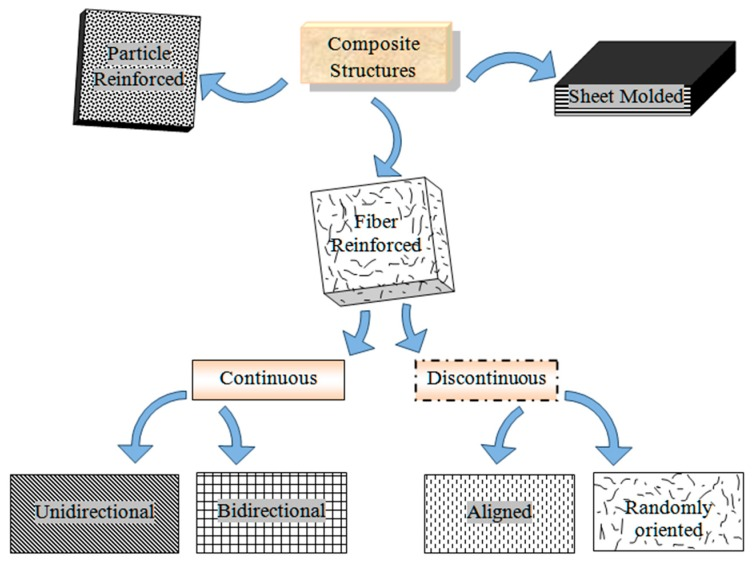
This paper presents a comprehensive review of the research advancements in fiber-reinforced polymer (FRP) composites. FRP composites, owing to their distinctive mechanical, lightweight, and corrosion-resistant properties, have emerged as essential materials across diverse fields. Structurally, they are categorized into micro-, meso-, and macro-structures, with fiber types, matrix polymers, and interface interactions being the decisive factors influencing their performance. Notably, recent years have witnessed remarkable progress. In civil engineering, the addition of specific substances has effectively augmented the properties of concrete. MXene, a two-dimensional nanomaterial, has demonstrated substantial potential in FRP composites. Its incorporation has led to enhanced mechanical strength, improved stability under harsh environmental conditions, and elevated electrical conductivity and thermal stability. Interface engineering plays a pivotal role in optimizing the performance of these composites. Furthermore, there is an increasing trend towards the development of multifunctional composites. In conclusion, this field is undergoing rapid evolution, and FRP composites are set to assume an increasingly significant position in future engineering applications.
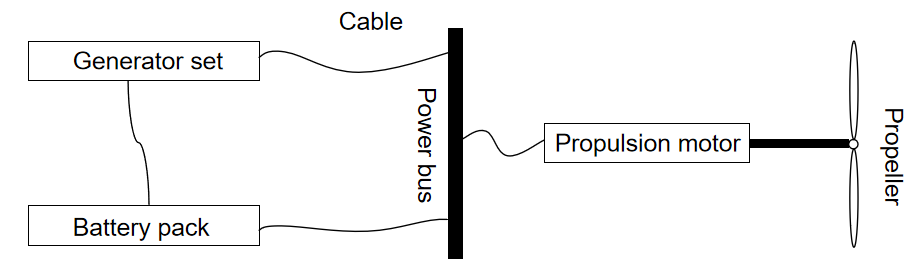
This paper explores contemporary trends and developments in the field of green shipping, with particular emphasis on the use of various types of fuel cells in hybrid-powered ships. The focus is on the thermal shock resistance of solid oxide fuel cells (SOFCs) and their specific application in hybrid ship propulsion systems. The effects of thermal shock on SOFCs are thoroughly examined, and a comprehensive summary of the various proposed and implemented solutions to mitigate these effects is provided. It is shown that the thermal shock resistance of SOFCs can be significantly improved through optimized design, which, in turn, extends the overall lifespan of the fuel cells. Enhancing the reliability of SOFCs in marine environments is critically dependent on appropriate material selection and structural optimization. This paper serves as an important reference for the sustainable development of hybrid-powered ships, offering insights into how the performance of SOFCs can be effectively managed and improved under the challenging conditions of the marine environment.
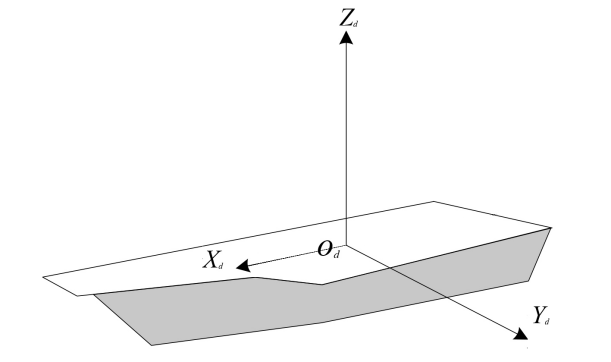
The coordinate systems for ship positioning and navigation are crucial as they enable ships to determine optimal routes, ensure navigational safety, and accurately locate their positions to avoid collisions. Additionally, these systems facilitate analyzing the impact of oceanic environmental factors on ship navigation, improving operational efficiency, and supporting marine-related operations. This paper reviews commonly used coordinate systems in ship positioning and navigation, including geodetic coordinate systems, body-fixed coordinate systems, transverse geodetic coordinate systems, grid coordinate systems, and pseudo-Earth coordinate systems. Their definitions, mutual conversion relationships, algorithms, and applications in ship navigation are analyzed, highlighting their respective advantages and disadvantages. In practical applications, the selection of appropriate coordinate systems and schemes must consider geophysical factors and the accuracy of navigation equipment. Future research on ship positioning and navigation coordinate systems will focus on the development of novel high-precision coordinate systems, efficient conversion algorithms, data fusion technologies, error source analysis and modeling, as well as the establishment of international standards and collaborations.
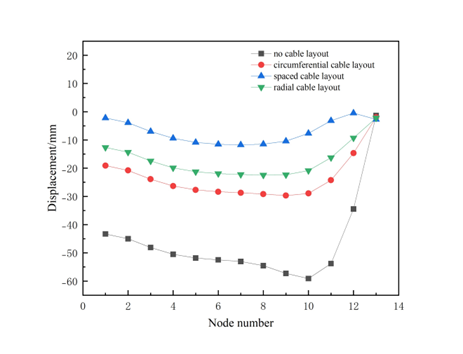
With the growth of energy demand, storage tanks are developing towards larger capacities. During this process, the stability of latticed tank tops is facing severe challenges. Based on the dome structure formed by prestressed cables combined with single-layer spherical latticed shells used in modern architectural structures, this paper applies it to the storage tanks in the petroleum industry, forming a new type of storage tank latticed tank top structure composed of prestressed cables combined with the single-layer latticed shell of the tank top, aiming to improve the stability of the tank dome. Static and stability analyses of the storage tank latticed tank top structure are carried out from aspects such as different cable layout methods, different magnitudes and positions of prestress. The results show that the storage tank latticed tank top with prestressed cables has better performance than the traditional single-layer latticed tank top without prestressed cables in terms of displacement, internal force distribution of members, and ultimate bearing capacity under the same working load. At the same time, the influence laws of prestress magnitude and position on the storage tank latticed tank top structure are obtained, and reasonable suggestions are given.
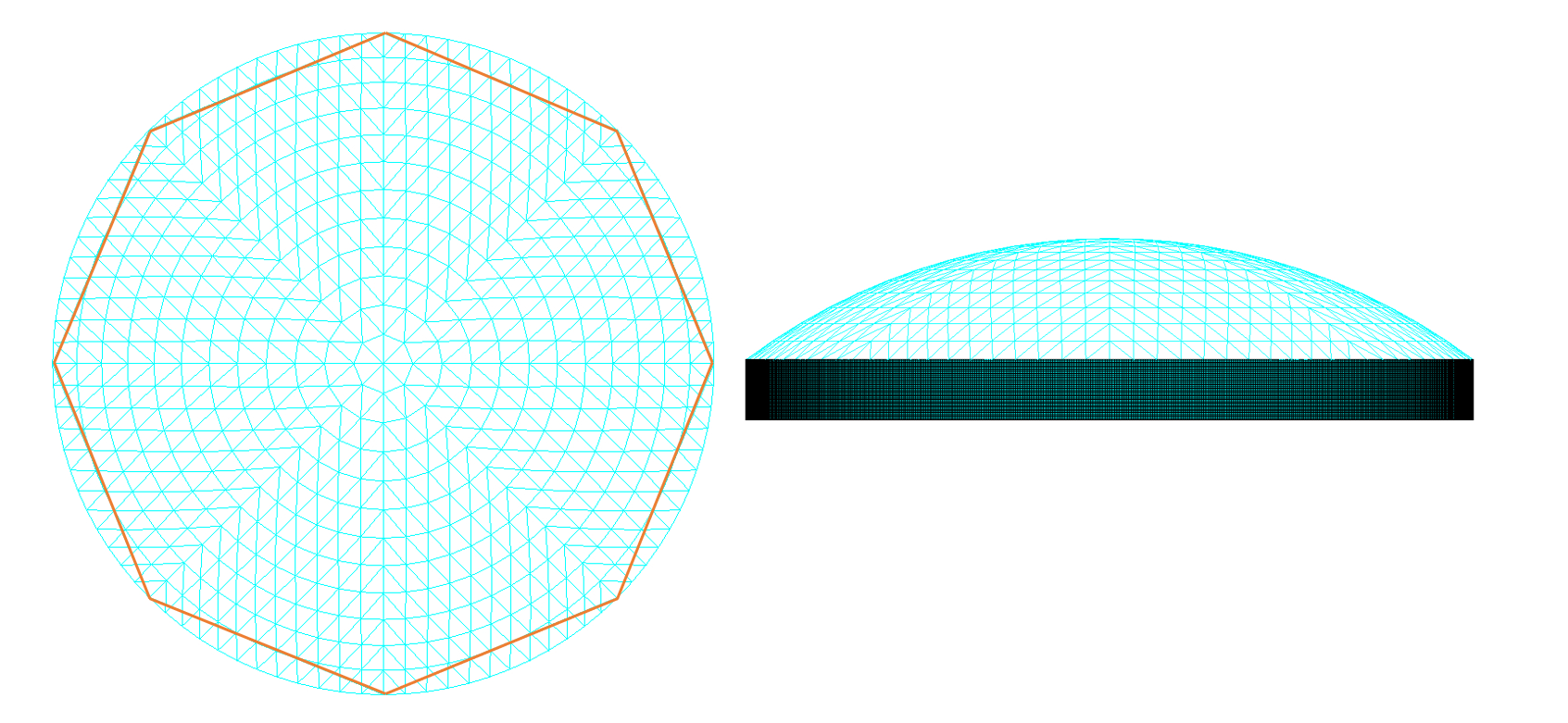
With the increase in the size of storage tanks, the larger spans of the tanks place higher demands on the safety of the shell structure. This study improves the traditional single-layer spherical shell structure used in oil tanks by adding prestressed cables to the original Kiewitt reticulated shell, creating a new type of prestressed shell structure. The prestressed cables help balance the internal forces in the shell members, significantly improving its overall ability to withstand earthquakes. This paper carefully examines how the prestressed shell affects the earthquake resistance of the Kiewitt reticulated shell, considering the support provided by the lower tank to the upper shell. The earthquake performance of the shell is analyzed using a time-based method, and the study explores how different levels of prestress affect the shell's response to earthquakes. Additionally, the paper compares how the shell behaves under steady and dynamic loads, focusing on how the prestressed shell deforms during strong earthquakes.
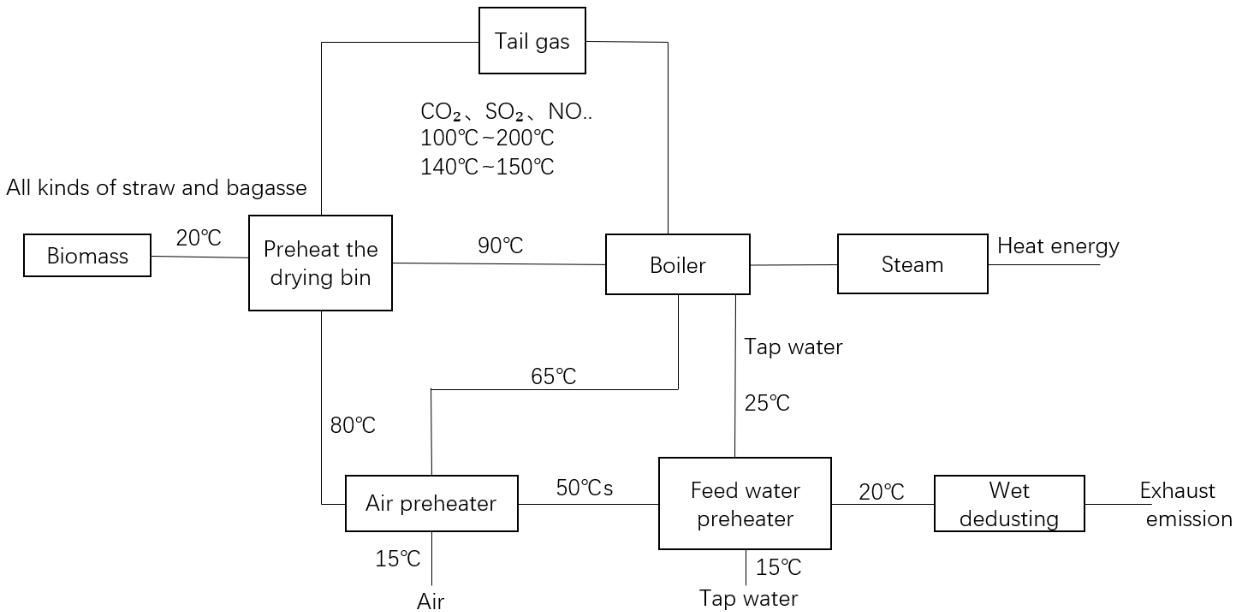
Biomass waste energy has the advantages of large quantity, low cost, and carbon neutrality, and has broad prospects for energy utilization. As the only renewable energy that can be directly transformed into fuel, biomass plays an important role in alleviating energy demand and achieving environmentally friendly energy use. It is also an important way for China to achieve the goal of "carbon neutrality". This paper focuses on the latest technical process and future development trends of biomass waste energy technology. This paper also introduces the related technologies of energy utilization, and analyzes the process of biomass liquefaction and gasification, and provides technical references for the energy utilization of biomass waste.
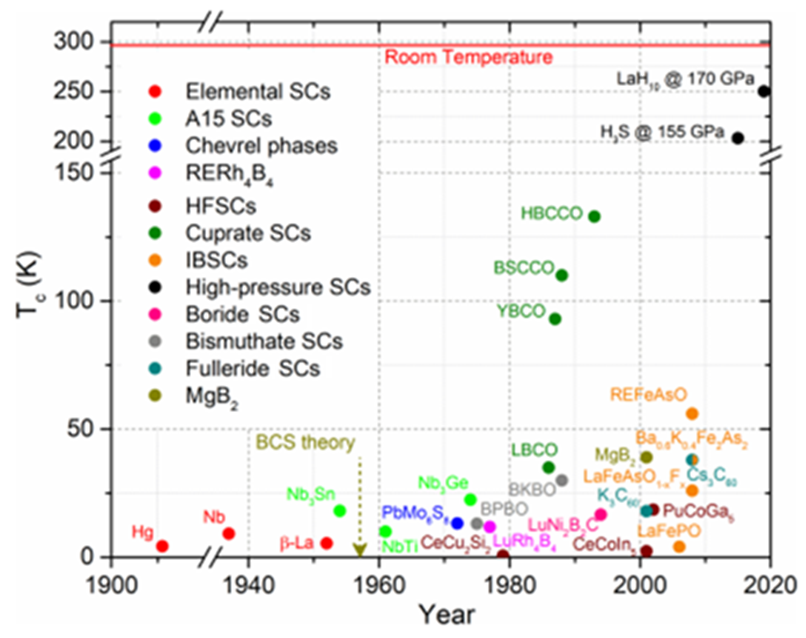
Superconductivity was discovered 113 years ago in 1911. Due to the unique properties of superconducting materials, such as zero resistivity and perfect diamagnetism, they play a critical role in energy, healthcare, and other industries. As scientific research continues to advance, various types of superconducting materials, including iron-based superconducting materials, have attracted increasing attention from scientists. This paper provides an overview of the research progress and the significant development potential of various iron-based superconducting materials (primarily the 11, 122 and 1111 systems), with the aim of offering guidance and suggestions to researchers.
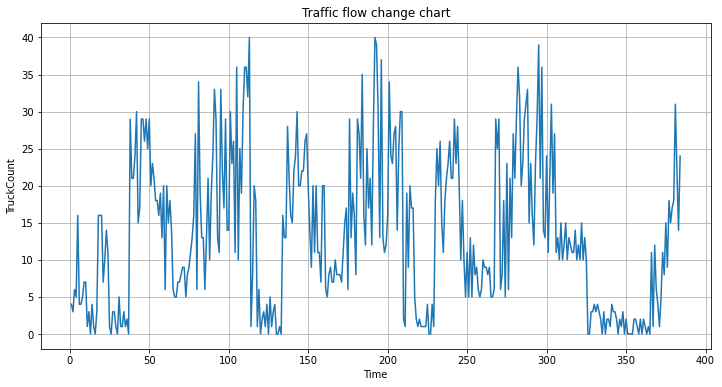
This paper proposes a traffic flow prediction model based on the fusion of time-domain convolutional network (TCN) and long-short-term memory network (LSTM), and verifies its effectiveness through multi-dimensional experiments. To address the complexity of urban traffic flow prediction, the study constructs a TCN-LSTM hybrid model to fuse temporal feature extraction and long and short-term dependency capturing capabilities, and performs prediction validation on three types of traffic flow datasets, namely, cars, bicycles and trucks, respectively. The experimental results show that: in car traffic prediction, the training loss value of the model decreases significantly from the initial 0.8 to less than 0.1, and the R² of the training set and the test set reaches 0.73 and 0.75, respectively, which reflects good convergence and generalisation ability; bicycle traffic prediction shows that the R² of the training set reaches as high as 0.84 but the R² of the test set decreases to 0.31, which shows that there is a certain degree of overfitting phenomenon; truck Truck traffic prediction achieves a balanced performance of R² 0.73 for the training set and R² 0.50 for the test set, which verifies the model's ability to capture heavy vehicle traffic patterns robustly. By comparing the performance differences between TCN, LSTM and their hybrid models through ablation experiments, it is found that TCN-LSTM is superior in key indicators: the mean absolute error (MAE) is 0.11 lower than that of the pure TCN model, the mean squared error (MSE) is in between that of TCN and LSTM, and the relative prediction deviation (RPD) reaches the highest value of the three at 2.15, which is a good proof that the hybrid model combines both multi-scale capturing ability of time-series features and the advantage of accurate modelling of nonlinear relationships.
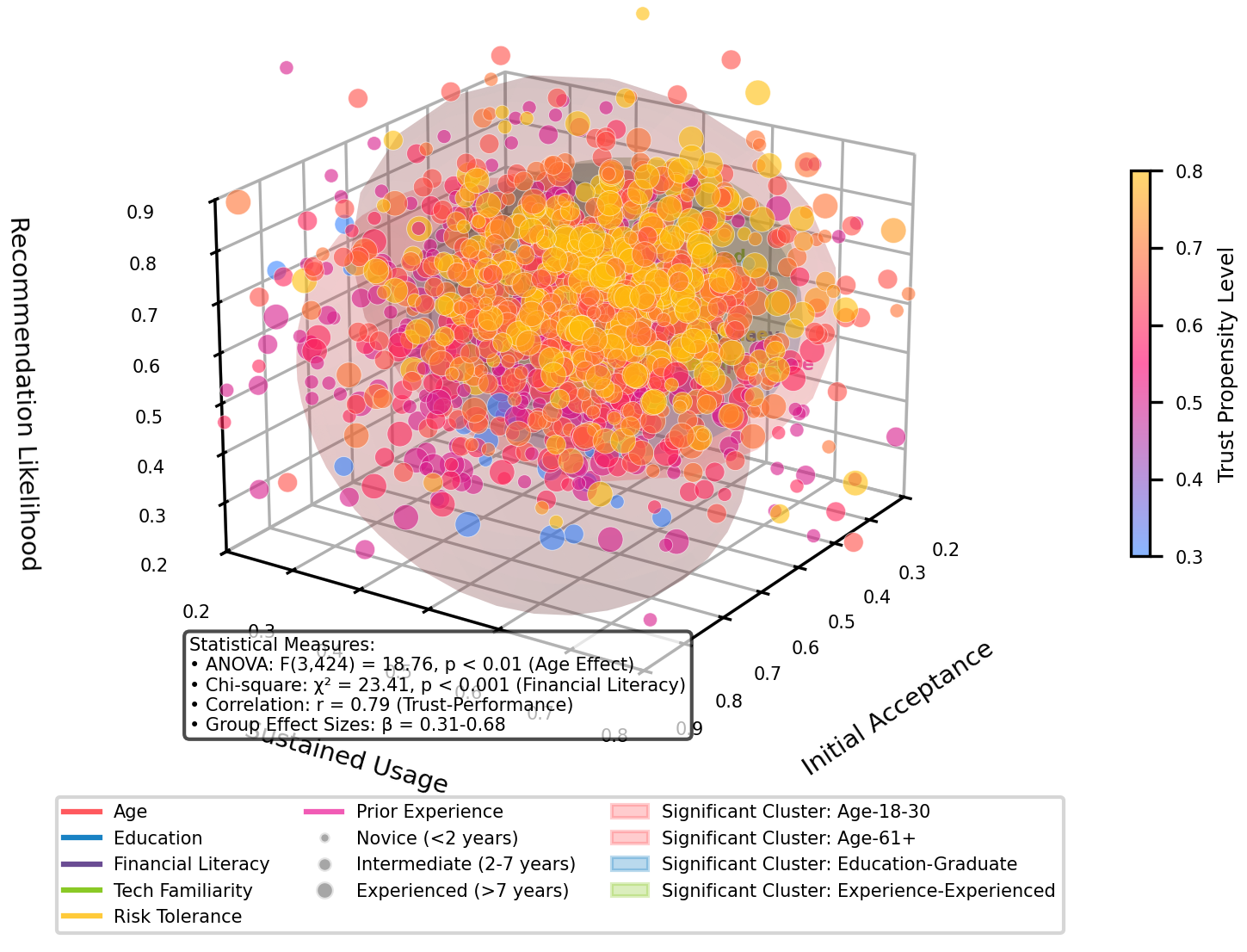
This study examines trust dynamics and decision quality among retail investors interacting with AI financial advisors. Using a convergent parallel mixed methods design incorporating surveys (n=428) and interviews (n=42), we investigate how trust forms, evolves, and influences investment outcomes. Results demonstrate that institutional affiliation (β=0.68, p<0.001) and perceived competence (β=0.57, p<0.001) significantly influence initial trust formation, while system explainability features substantially impact trust sustainability (high-explainability M=4.76 vs. low-explainability M=3.28, p<0.001, d=1.78). Longitudinal analysis reveals non-linear trust trajectories with distinct investor classes showing increasing (58.2%), stable (23.7%), or degrading (18.1%) patterns over nine months. AI-advised portfolios outperformed self-directed investments by 7.2% on risk-adjusted returns (Sharpe ratio: 0.83 vs. 0.65, p<0.01) with significant reductions in overconfidence bias (41.3%) and disposition effect (37.8%). Financial literacy moderates these benefits, with high-literacy investors showing smaller performance differentials between AI-advised and self-directed conditions compared to low-literacy participants. Transparency regarding system limitations demonstrates particularly strong effects on trust calibration, with 76.4% of participants in transparent conditions exhibiting appropriate reliance patterns versus 34.2% in non-disclosure conditions. These findings advance understanding of human-AI interaction in financial contexts and provide implications for designing trustworthy advisory systems that enhance retail investor outcomes.
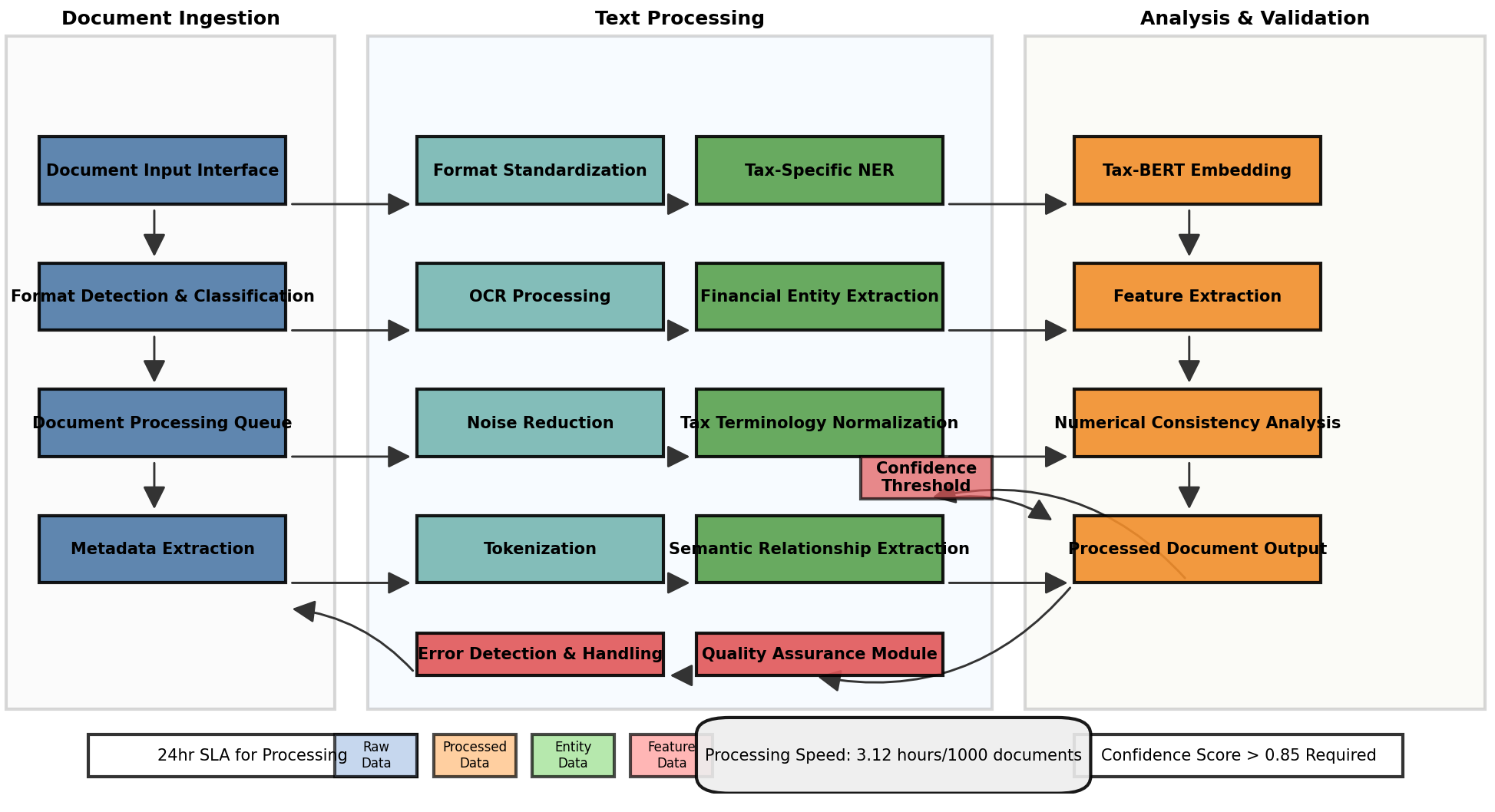
This paper introduces a novel approach to tax fraud detection utilizing natural language processing techniques for identifying anomalies in tax filing documents. The methodology integrates tax-domain specific BERT embeddings with bidirectional LSTM networks to capture contextual relationships within tax documents that traditional numerical analysis might overlook. We present a multi-component ensemble framework that processes both structured and unstructured components of tax filings, extracting semantic relationships between financial entities while maintaining sensitivity to numerical inconsistencies. Using a dataset of 15,000 tax documents with 8.5% labeled anomalies, our approach demonstrates superior performance compared to existing methods, achieving an F1-score of 0.868 and AUC of 0.931—a 7.6% improvement over state-of-the-art techniques. The framework exhibits varying effectiveness across document types, with higher detection accuracy for individual income tax returns (F1-score 0.889) compared to business tax declarations (F1-score 0.818). Performance analysis reveals that semantic relationship features contribute significantly to anomaly detection in business tax documents, while numerical consistency features dominate in individual returns. Despite computational requirements exceeding traditional methods, the enhanced detection capabilities address critical gaps in existing tax fraud detection systems, particularly for sophisticated evasion strategies that manipulate textual elements while maintaining numerical plausibility.