Volume 51
Published on November 2024Volume title: Proceedings of CONF-MPCS 2024 Workshop: Quantum Machine Learning: Bridging Quantum Physics and Computational Simulations
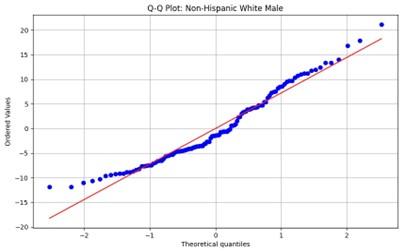
The rate of obesity across the world has seen a steady increase in the past several decades. This disease can lead to significant harm associated with one’s body functioning and mental health. The potential causes of obesity incorporate social, environmental, and personal factors. This article attempts to cover the correlation between obesity and income level using a linear regression model. Samples were extracted from the National Health and Nutrition Examination Survey about 545 individuals from 2017 to 2020. The dataset was subdivided into four cohorts, which include non-Hispanic White male, non-Hispanic White female, non-Hispanic Black male, and non-Hispanic Black female, respectively, to avoid variables that might confound with the explanatory variable. The study found out that the correlation for White male, White female, and Black female can be either positive, negative, or non-existent, and positive on the other hand for the Black male cohort. However, the study concluded that the linear regression model is ineffective for the topic of analysis as the metrics indicate. The incompatibility of the model can be attributed to the fact that other variables that can possibly confound with the explanatory variable are neglected and not filtered out.
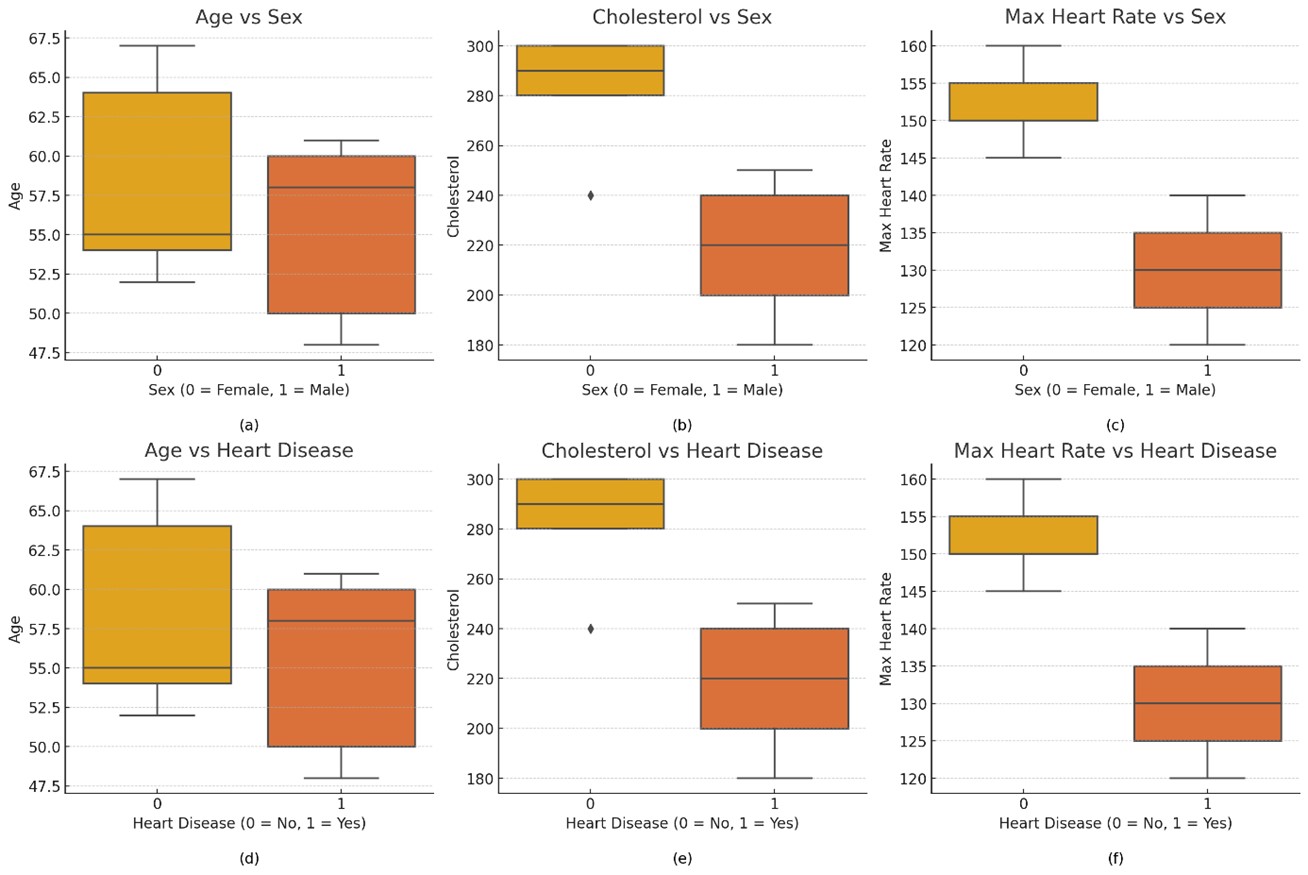
Heart disease remains a global health threat, making rapid identification crucial. Using the Heart Disease Dataset from Kaggle, this research employs a Random Forest model to analyze 13 clinical variables from 1,025 samples. To improve accuracy and address class imbalance, the dataset was split into training and test sets, utilizing methods such as Z-scores, SMOTE, and feature selection. The Random Forest model, which combines multiple decision trees, achieved high performance with an accuracy of 98.54%, identifying key predictors such as chest pain type, maximum heart rate, and thalassemia. Compared to a single decision tree, the Random Forest model reduces overfitting, improves generalization, and increases predictive accuracy. Factors like cholesterol levels, resting blood pressure, and exercise-induced angina were also considered. By averaging results from multiple trees, the model offers reliable and stable predictions, highlighting its potential in clinical settings for early detection and personalized treatment strategies. This study aims to assist healthcare providers in better allocating resources, planning preventive measures, and tailoring treatment plans to individual patients.
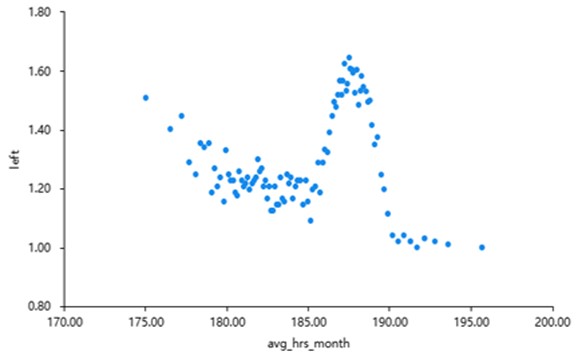
Nowadays, the enterprise employee turnover rate has become an important factor in measuring enterprise knowledge loss, human resource management, enterprise comprehensive competitiveness, and enterprise expenditure. Therefore, as much as possible may affect the enterprise employee turnover data and analyze the influence on employee turnover degree can maintain stable staff and increase its comprehensive competitiveness in the market economy system. Employee mobility is not only affected by corporate and macro environmental factors, such as corporate culture, incentive system, employee relations, economic environment, but also related to employees' factors, such as career development planning, job satisfaction, salary, and burnout. Research on employee mobility and its impression factors should be carried out from multiple aspects and perspectives. This paper refers to the relevant literature on the impression factors of employee mobility in the past, combines the basic data that may affect the employee turnover rate to analyze the Impact of employees' indicators on employee mobility, pre-processes the data, and presents the visual image of the data. And then the paper uses multicollinearity diagnosis to exclude the collinearity influencing variables and conduct a preliminary correlation analysis of the variables. Finally, this text establishes a regression analysis model of the related variables, obtains seven variables related to employee mobility, and gives reasonable suggestions for the enterprise research employee turnover rate.
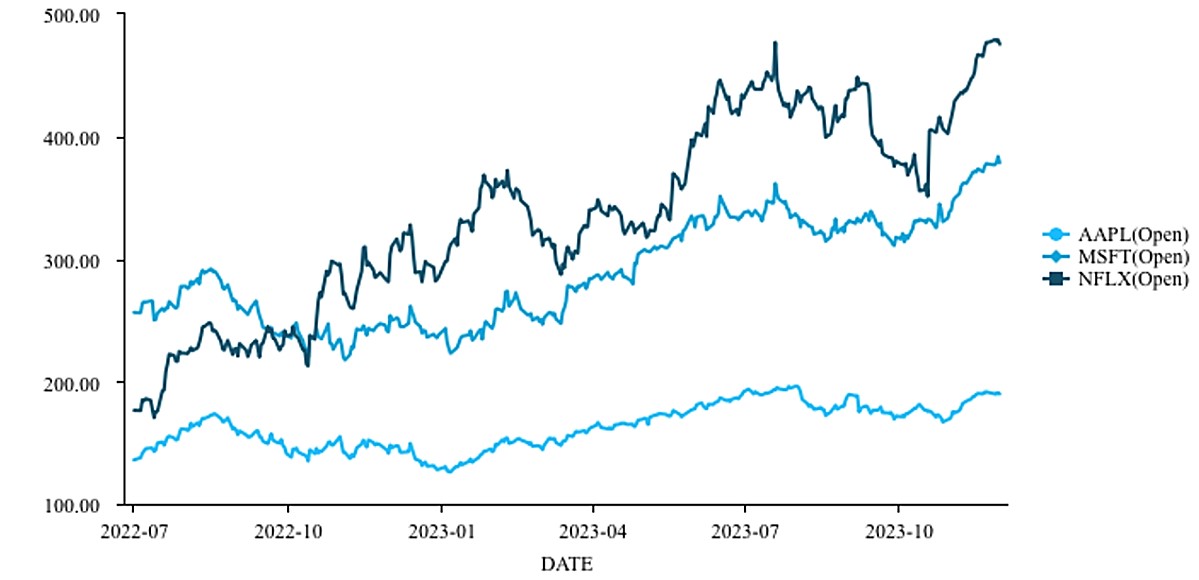
Stock market has been and will always be the central of the financial market. It is also a leading indicator of the economy react before the business cycle. Thus, predicted the trend of the stock market should be considered by market participants, policymakers and academicians. Simultaneously, technology stock market is gradually dominating the market. Under such a background, this paper will forecast the trend of the technology stock market based on a few technology companies, which are highly weighted in the technology industry, via the Autoregressive Integrated Moving Average (ARIMA) model. This study has successfully predicted the price of the technology stock market, which the predicted outcome is a close match to the actual price. The trend of the technology market analyzed by the ARIMA model with first order difference parameters, is going upward. The result represents American business cycle is undergoing a transition from contraction phase to recovery phase to some extent.
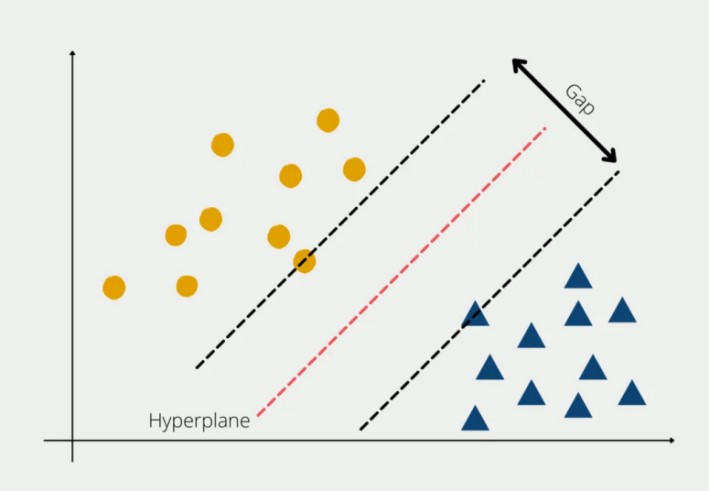
Quantum Support Vector Machines (QSVMs) combine the fundamental principles of quantum computing and classical Support Vector Machines (SVMs) to improve machine learning performance. In this paper, the author will further explore QSVM. Firstly, introduce the basics of classical SVM, including hyperplane, margin, support vector, and kernel methods. Then, introduce the basic theories of quantum computing, including quantum bits, entanglement, quantum states, superposition, and some related quantum algorithms. Focuses on the concept of QSVM, quantum kernel methods, and how SVM runs on a quantum computer. Key topics include quantum state preparation, measurement, and output interpretation. Theoretical advantages of QSVMs, such as faster computation speed, stronger performance to process high-dimensional data, and kernal computation. In addition, the author discussed the implementation of QSVM, quantum algorithms, quantum gradient descent, and optimization techniques for SVM training. The article also discusses practical issues such as error mitigation and quantum hardware requirements. The purpose of this paper is to show the advantages of QSVM by comparing SVM and QSVM.
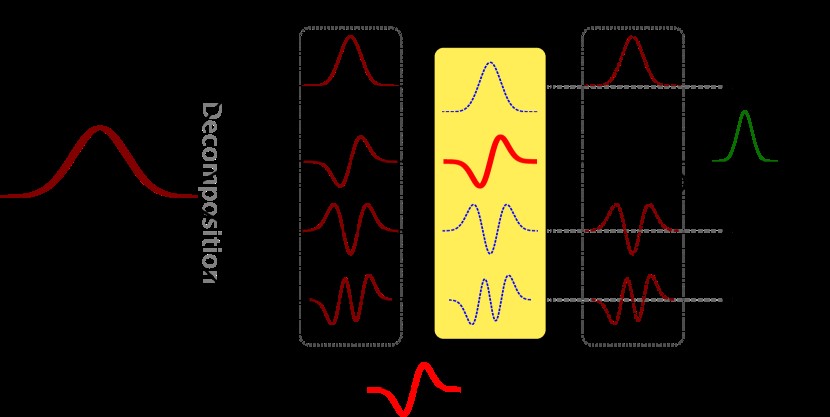
This article delves into the fundamental aspects of qubits in quantum computation, emphasizing their coordination, mathematical representation through the Bloch Sphere, and the unique advantages and challenges of superconducting, trapped ion, and photonic qubits. It highlights the transformative potential of quantum algorithms in solving complex problems in cryptography and information security, while addressing key sources of quantum errors such as decoherence and quantum noise. The study discusses advanced error correction methods and underscores the necessity of improving qubit coherence, stability, scalability, and integration for the practical application and commercialization of quantum computers. Ongoing research and collaboration are deemed essential for overcoming technical challenges and unlocking the full potential of quantum computation across various industries.
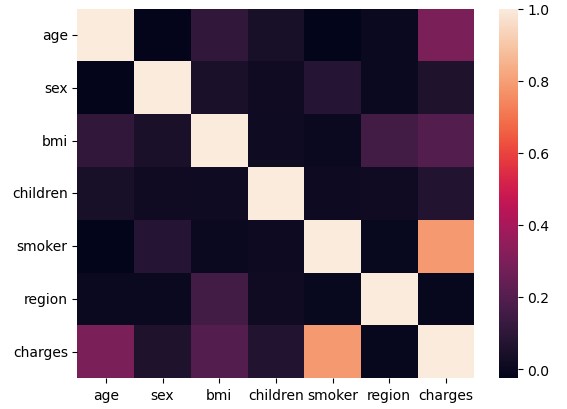
Linear regression method can be performed to predict the outcome from one or many input values. Its versatility allows it to be applied on many datasets that contain correlated values. However, researches on the application of linear regression on medical insurance costs, a highly important part of people’s life, are few. This paper studies an insurance dataset from Kaggle by applying linear regression on it. The author validates the dataset at first and explores the correlation between each individual factor and their corresponding charges to better show how insurance costs differ from person to person with different background. Many figures are also included to help visualize the correlation between factors. In the end, the author creates a multilinear regression model to predict the insurance charges. The R-Squared score of the model and a result table including regression coeffects are also provided to show the accuracy and details of the model.
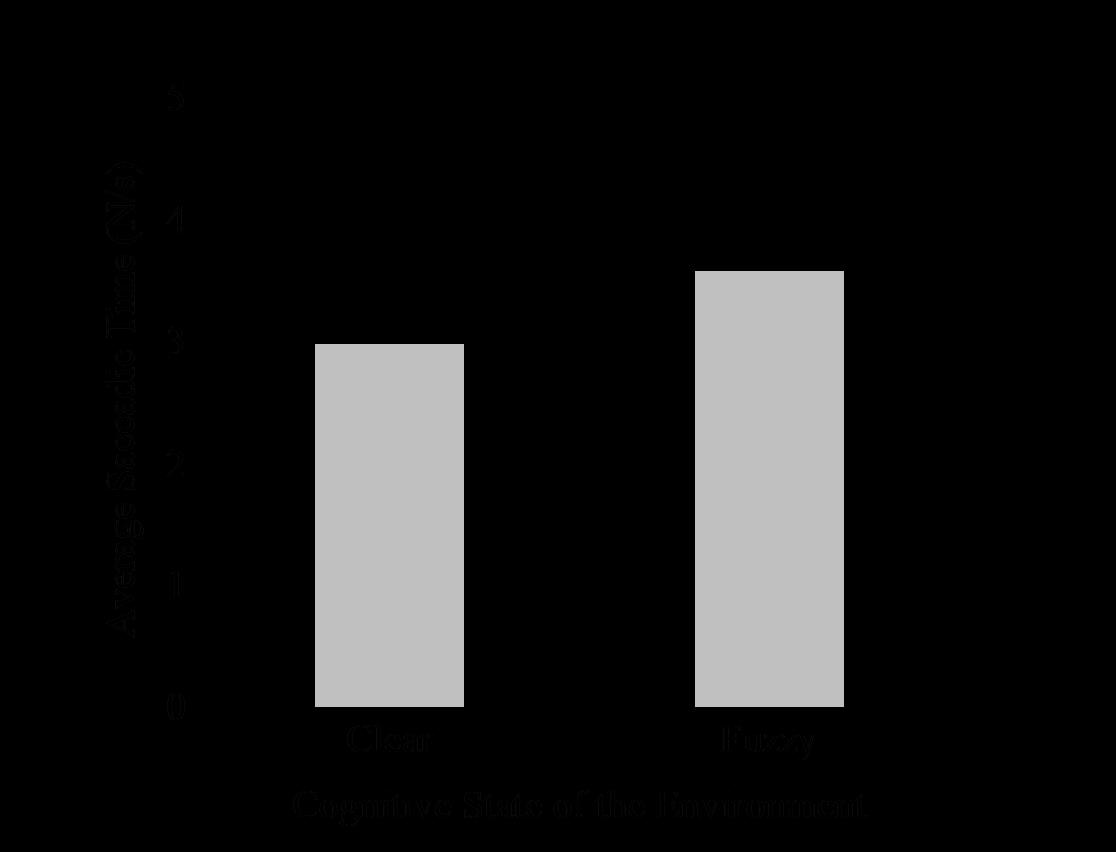
In the recent development of Autonomous Vehicles (AVs), human-machine trust and take-over strategy are important research contents, which is related to whether the driving system can make the most of its performance and ensure driving safety. At the same time, the unique advantages of Multi-Sensor Information Fusion (MSIF) also make it more and more applied to the auxiliary driving system. At present, research on MSIF applications in automated driving mainly focuses on external environment perception and map construction. However, there is little research on the application of MSIF in vehicle Human-Machine Interaction (HMI). Therefore, this paper analyzes the application of MSIF in trust detection and take-over HMI, proposes the advantages of applying MSIF to the HMI of AVs, and points out that the HMI design of intelligent vehicles in the future can enhance the application of MSIF. Dynamic monitoring of human-machine trust and dynamic adjustment of HMI strategy of the intelligent driving system are realized to achieve a better effect and further guarantee driving safety.
In recent years, unmanned aerial vehicles (UAVs) have seen extensive use in fields such as agriculture, search and rescue, commercial, and military operations, driving the demand for autonomous navigation capabilities. Though GPS is the traditional method used for navigation, it becomes problematic in harsh environments like deserts. The Visual Simultaneous Localization and Mapping technology offers a solution to enhance UAV navigation in complex environments by constructing maps and localizing the UAV simultaneously in real time. This paper presents state-of-the-art visual SLAM technology developed for UAV navigation regarding algorithms like Oriented FAST and Rotated BRIEF SLAM (ORB-SLAM) and Large-Scale Direct Monocular SLAM (LSD-SLAM), whereby their performance is also discussed, with its positives and negatives. In this regard, the latest progress and challenges in applications are reviewed and analyzed through relevant literature from the databases of PubMed, IEEE, and Google Scholar in the past five years. The novelty of this paper lies in the comprehensive evaluation of the application performance of different visual SLAM algorithms in UAV navigation and the proposal of future research directions.
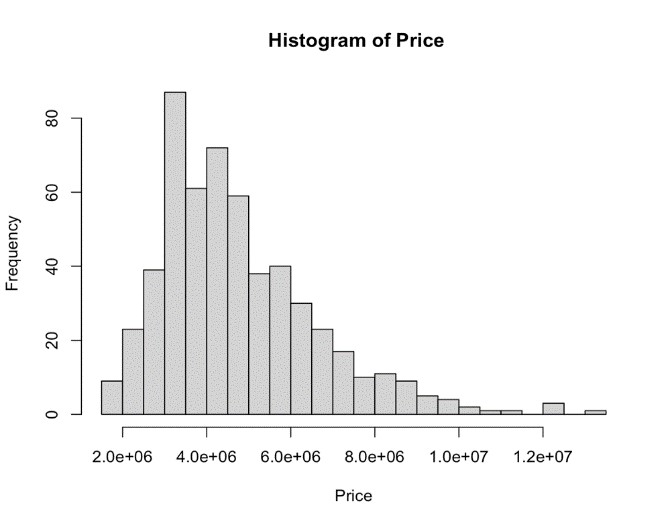
The method is to construct a multiple linear regression model to examine the variables that significantly affect US home prices. The dataset has 13 variables and 545 observations, and it was obtained via Kaggle. This paper also considers comparing the multiple linear regression models and random forest model for predicting house prices and thus concludes which model can most accurately predict house prices. The result indicates that the factors including area, quantity of bedrooms and bathrooms, the existence of a basement, and state of the furnishings, etc. significantly impact housing prices. By comparing Root Mean Squared Error (RMSE) and Mean Absolute Percentage Error (MAPE), the conclusion suggests that the multiple linear regression model marginally surpasses the random forest regarding the accuracy of the predictions, despite the fact that both models function similarly. This research offers practical applications for enhancing real estate market strategies and provides valuable insights into the factors influencing housing prices.