Volume 95
Published on March 2025Volume title: Proceedings of the 2nd International Conference on Applied Physics and Mathematical Modeling
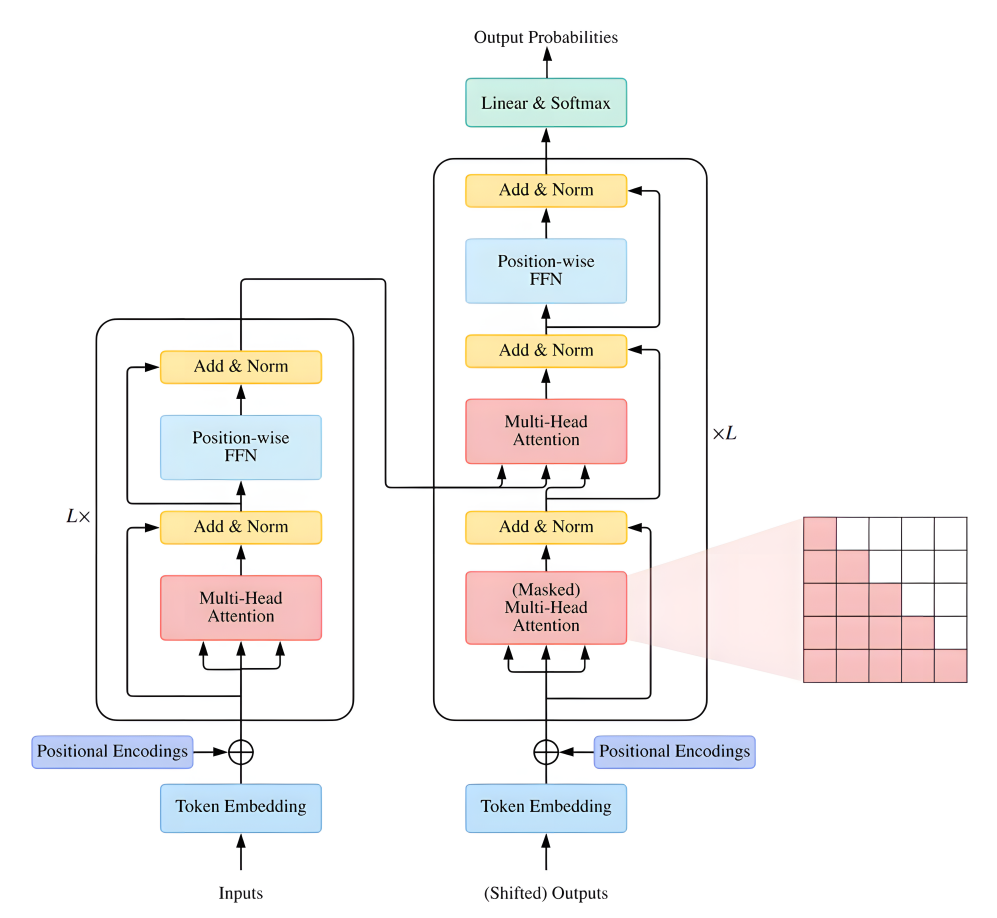
This article optimizes the transformer model based on swarm intelligence optimization algorithm to enhance the predictive ability of users' virtual reality (VR) experience. By analyzing the accuracy and loss value changes of the training set, we found that the model showed significant improvement during the training process. Specifically, the accuracy of the training set gradually increased from 62.74% to 86.94% and stabilized; At the same time, the loss value also decreased from 0.71 to 0.37, showing a good convergence trend. This indicates that the model effectively captures important features in the data during the learning process, thereby improving its predictive performance. Further analyzing the confusion matrix of the training set, we can see that 552 VR immersive predictions are correct, while 92 have errors. Among them, 55 instances that should have been predicted as Level 1 immersion were mistakenly classified as Level 2 immersion, while 37 instances that should have been Level 2 immersion were incorrectly predicted as Level 1. This reflects the confusion of the model in certain categories, despite achieving an overall accuracy of 85.69%. On the test set, 230 predicted results were correct, while 45 were not. Among them, 29 instances that should have been level 1 immersion were misclassified as level 2, and 16 instances that should have been level 2 immersion were misclassified as level 1, resulting in an accuracy rate of 83.63% on the test set. In addition, by outputting the ROC curve of the test set with an AUC value of 0.829, it further proves that the model has good classification performance. In summary, this study significantly improved the accuracy of transformer models in predicting VR experiences through swarm intelligence optimization algorithms. This achievement not only validates the effectiveness of swarm intelligence technology in complex data processing, but also provides new ideas and methods for future related fields. With the development of virtual reality technology, accurate prediction of user experience will help improve product design and service quality, thereby promoting the development of the entire industry.
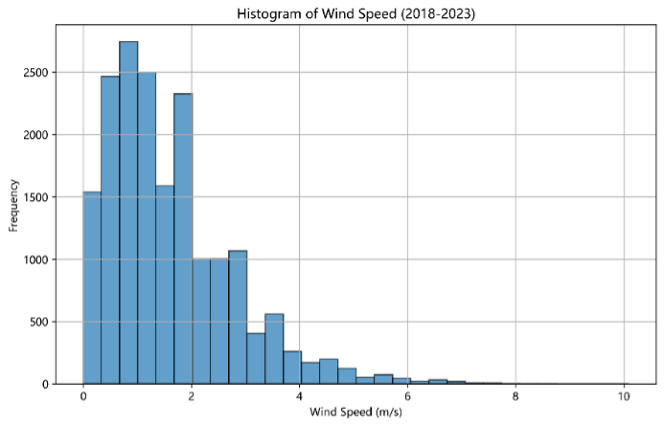
Wind speed variations have a significant impact on the aerodynamic characteristics of F1 racing cars and the race outcomes. To enhance the accuracy and stability of wind speed forecasting, this paper proposes a method based on multi-scale decomposition and time series neural network models. Initially, wind speed data collected from F1 Grand Prix events between 2018 and 2023 are subjected to Empirical Mode Decomposition (EMD), which decomposes the complex nonlinear and non-stationary time series data into several Intrinsic Mode Functions (IMFs) with different time scales. Subsequently, a Long Short-Term Memory network (LSTM) is utilized to predict each IMF component, and the predicted results of all IMFs are reconstructed into the overall wind speed forecast. Experimental results demonstrate that the method based on multi-scale decomposition and time series neural network models significantly outperforms traditional models such as Random Forest, Gradient Boosting Decision Tree (GBDT), and single LSTM models in terms of forecasting accuracy and stability. This study offers a novel perspective and approach for wind speed prediction in F1 Grand Prix events, with important theoretical significance and practical application value.
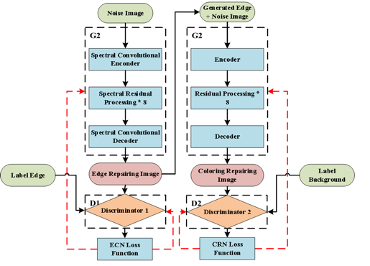
Aiming at the problem of snowflake occlusion and haze veil effect in the collected images by the vehicle-mounted camera for the influence of snowflakes and haze. The decoupling and double-supervised weather elimination network was proposed based on the sequential reconstruction of high-frequency and low-frequency components in background images. Decoupling the desnow and dehaze assignment to two sub-networks sequentially, the network filters the high-frequency feature vectors in the image background region by the eigenvalues of the convolution kernel in the spatial domain, and then the second subnetwork performs image coloring and edge fine-tuning tasks based on edge context features obtained by generative adversarial networks. The algorithm has tested on the SRRS-6000 dataset, which verified the effectiveness in the significant performance on noise removal. The Peak Signal to Noise Ratio (PSNR) and Structural Similarity (SSIM) has reached 33.29dB/0.94, 32.8dB/0.9316, 30.13dB/0.93, 25.88dB/0.82 on Snow100K-S、Snow100K-M、Snow100K-L、I&O-Haze dataset, respectively. Experiments have shown that the decoupling and double supervised method have efficient snowflake and haze removal performance in image denoising tasks, and the adaptability of unmanned assistance systems under complex weather conditions has been enhanced.
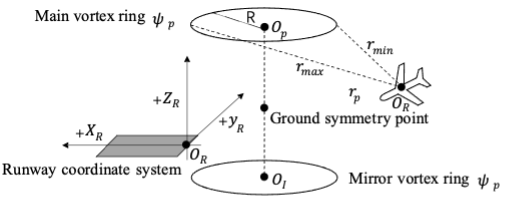
To address the impact of low-altitude wind shear on aircraft flight safety, this paper establishes a real-time low-altitude headwind shear model based on the vortex ring method and an aircraft dynamic model incorporating disturbance wind parameters. The study investigates the effects of wind shear on aircraft and proposes both longitudinal and lateral escape strategies. In the simulation study, vertical and horizontal wind vector fields of wind shear were first simulated, and wind shear vector diagrams were plotted. Then, using the B747-100 aircraft as the research object, a longitudinal escape numerical simulation was conducted at 80 seconds by setting relevant parameters, followed by a lateral escape simulation at 45 seconds. The results indicate that low-altitude wind shear can lead to a decrease in aircraft airspeed, significant changes in pitch angle, and a reduction in flight altitude, thereby increasing the risk of a crash. Additionally, when encountering wind shear, implementing a longitudinal escape strategy (maintaining a 15° pitch angle and full thrust) can effectively recover flight altitude. The lateral escape strategy also successfully allows the aircraft to escape wind shear. This research provides theoretical foundations and technical support for enhancing aviation flight safety.

Gastrointestinal (GI) tract disorders, ranging from benign polyps to aggressive forms of cancer, pose significant health challenges globally. Early detection and precise classification of these conditions are crucial for effective treatment and improving patient survival rates. This study employs the Hyper-Kvasir dataset, a comprehensive collection of endoscopic images, to develop deep learning models that harness the power of the YOLO (You Only Look Once) architecture for real-time detection and classification of GI abnormalities. The focus is on overcoming inherent challenges such as class imbalance and limited annotated data availability. Advanced machine learning strategies, including data augmentation and semi-supervised learning, are utilized to enhance the model's performance. Our experiments demonstrate notable improvements in the detection of pre-cancerous lesions and other GI abnormalities, confirming the potential of integrating AI into endoscopic practices to support clinicians, reduce diagnostic errors, and contribute to more accurate and timely diagnoses. The implications of these findings are significant, offering a pathway to more reliable diagnostic processes and ultimately, better patient management in gastroenterology.
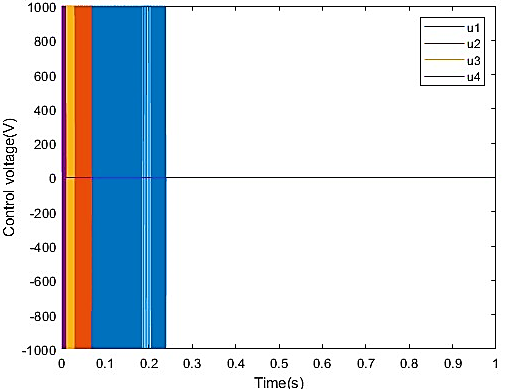
This paper studies the active vibration control of membrane structure by piezoelectric actuator. Firstly, the nonlinear vibration equation is established for membrane structure and the vibration equation is supplemented on the basis of the system equation established by Liu Xiang et al., and then the multi-mode dynamic model is obtained by Galerkin decomposition for the four-degree-of-freedom modes. After that, the Sliding Mode Controller is designed to suppress the large initial displacement vibration of the membrane structure and the continuous external excitation of the membrane structure. The stability of the controller is proved by using the Lyaplov stability theory and the effectiveness of the controller is verified by numerical simulation. In addition, the control effect of synovial control is compared with that of negaive velocity feedback control, which proves that synovial control has better robustness.
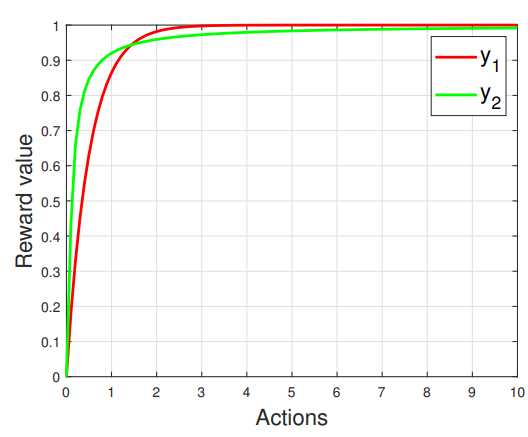
The fifth generation of wireless communication technology (5G), has revolutionized the digital landscape with its ultra-fast speeds, massive connectivity, and reduced latency; Beyond 5G (B5G), represents the evolutionary steps towards sixth-generation networks which aims to build upon 5G’s capabilities by integrating advanced technologies like ultra-dense network, edge computing, and enhanced spectral efficiency. This paper investigates the application of two distinct exponential reward functions in reinforcement learning algorithms for power allocation in ultra-dense networked base stations. The primary objective is to maximize the overall network capacity and spectral efficiency. The performance of the proposed reinforcement learning algorithms is compared with the traditional water-filling algorithm, as well as against the other to highlight the differences in learning outcomes resulting from the choice of reward functions. The results show that the exponential function model with reciprocal exponent is superior to the previous two in spectral efficiency and convergence speed and provide valuable insights into the effectiveness of using reinforcement learning for complex resource allocation problems in modern communication networks.
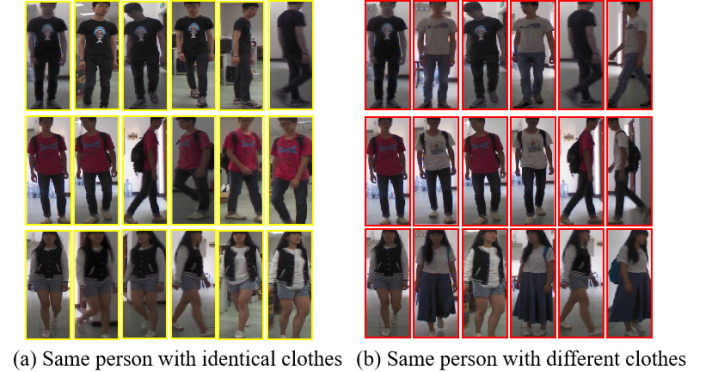
In recent years, person re-identification (ReID) has experienced significant advancements due to its diverse real-world applications. However, traditional benchmarks often assume consistent attire across captured images, failing to reflect the reality of pedestrians frequently changing their clothing. This discrepancy has led to the emergence of the cloth-changing person re-identification (CC-ReID) problem and the development of relevant benchmarks. CC-ReID poses a substantial challenge, as pedestrians’ primary visual cues, particularly their clothing, vary between query and gallery images, while non-attire-related features remain relatively weak. To address this gap and advance research in CC-ReID, this paper introduces a novel task termed Silhouette-Keypoint Fusion Re-Identification (SKF-ReID). This represents a dual-stream framework capable of extracting silhouette and keypoint details within the shape stream, subsequently transferring this data to the ReID stream to enrich appearance features with clothing-independent insights. Additionally, we employ the Maximum Mean Discrepancy (MMD) loss to ensure similarity between shape and appearance features, thereby enhancing the accuracy of cloth-changing person re-identification. Our proposed approach undergoes rigorous evaluation across benchmark cloth-changing person re-identification datasets, showcasing cutting-edge performance.
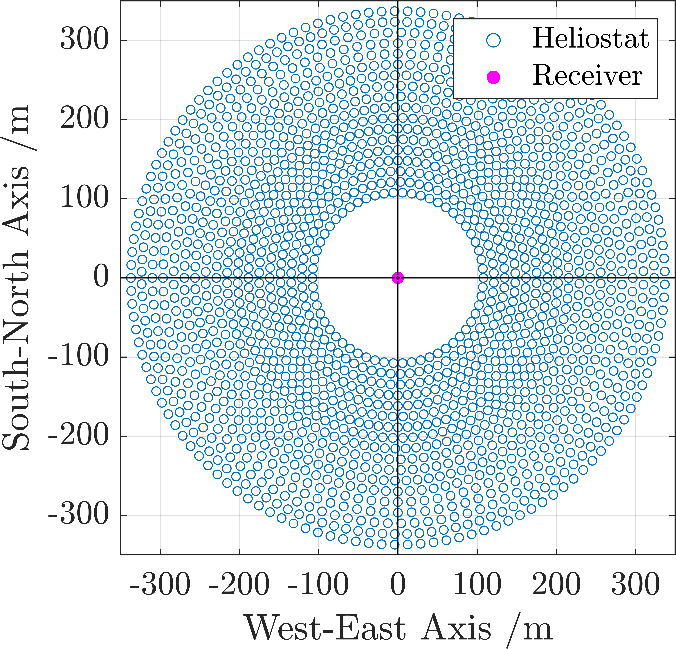
This study presents a novel computational approach to enhance the efficiency of calculating shading and blocking in heliostat fields for solar tower power plants. Traditional methods for determining shading and blocking efficiency often involve substantial computational overhead due to the need to evaluate numerous heliostats within a field. To address this, our proposed method focuses on predicting potential heliostats that may cause shading or blocking, thereby significantly reducing the computation time and resource consumption. Through experimental validation, we demonstrate that our method maintains high computational accuracy and reliability while improving computational efficiency. This approach is critical for optimizing heliostat field layouts, ultimately leading to improved efficiency and reduced costs in solar tower power stations.
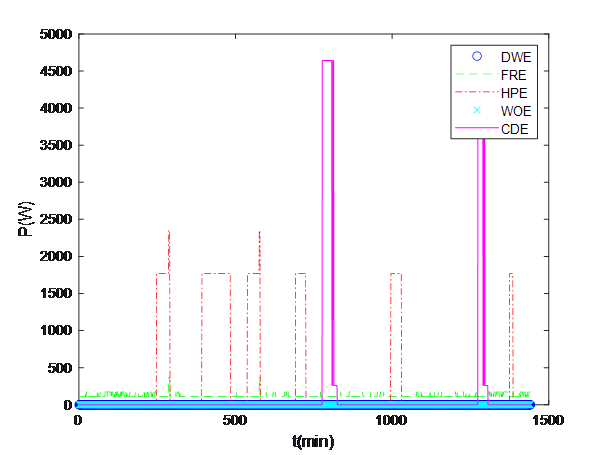
In Non-Intrusive Load Monitoring (NILM), the commonly used methods based on hidden Markov model (HMM) often neglect time feature of load states, leading to increased bias in the estimation of HMM parameters. To address this issue, this paper proposes a time feature based hidden Markov model for NILM. It employs an improved K-means algorithm to identify appliance states at different times slots and establishes the correspondence between state combinations and hyper-states through binary encoding. Finally, a simplified Viterbi algorithm is used for state estimation. Experimental results on the AMPDS2 dataset show that this method can enhance the monitoring accuracy of HMM in NILM, providing more precise identification of individual load states and power consumption.